Research Article
A Two-Parameter Power Garima Distribution with Properties and Applications
Berhane Abebe, Mussie Tesfay, Tesfalem Eyob and Rama Shanker*
Department of Statistics, Eritrea
Rama Shanker, Department of Statistics, College of Science, Eritrea Institute of Technology, Asmara, Eritrea.
Received Date: January 05, 2019; Published Date: January 24, 2019
Abstract
In this article we propose a two-parameter power Garima distribution which includes Garima distribution as particular case. Its statistical properties including behavior of its probability density function for varying values of parameters, moments, hazard rate function and mean residual life function has been discussed. The estimation of the parameters of the distribution has been discussed using maximum likelihood estimation. Applications of the distribution have been investigated using real lifetime datasets from engineering and medical science.
Keywords:Garima distribution; Moments; Hazard rate function; Mean residual life function; Maximum likelihood estimation; Applications
Introduction
Shanker [1] introduced one parameter lifetime distribution having probability density function (pdf) and cumulative distribution function (cdf) as
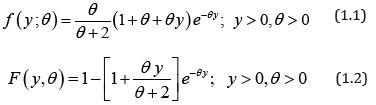
Garima distribution (1.1) can be easily expressed as a mixture
of exponential (θ ) and gamma (2,θ ) distributions with mixing
proportion . We have
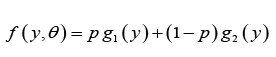
where

Shanker [1] has discussed some of its statistical properties including shapes for varying values of parameter, moments, skewness, kurtosis, hazard rate function, mean residual life function, stochastic ordering, mean deviations, order statistics, Bonferroni and Lorenz curves, entropy measure and stressstrength reliability. The condition under which Garima distribution is over-dispersed, equi-dispersed, and under-dispersed has been also discussed along other one parameter lifetime distributions. Maximum likelihood estimation and method of moments have been discussed for estimating its parameter. An application of Garima distribution for modeling life time data from behavioral science has been also discussed in Shanker [1]. Shanker [2] has also introduced discrete Poisson-Garima distribution and discussed its statistical properties, estimation of parameter and applications. Shanker & Shukla [3,4] have studied size-biased Poisson-Garima distribution and Zero-truncated Poisson-Garima distribution.
The pdf and the cdf of Power Lindley distribution (PLD) obtained by Ghitany et al [5] are given by
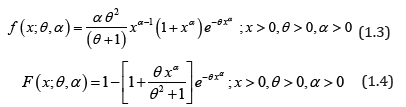
It can be easily shown that at α =1, PLD reduces to one parameter Lindley distribution,introduced by Lindley (1958) having pdf and cdf
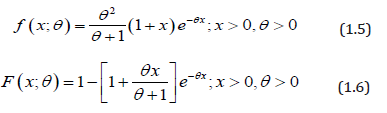
The detailed study regarding various statistical properties, estimation of parameter and application of Lindley distribution are available in Ghitany et al. [7]. Shanker et al. (2015) have critical and comparative study on applications of Lindley distribution and exponential distribution to model real lifetimes data from engineering and biomedical sciences. Garima distribution, being one parameter lifetime distribution, has less flexibility to model data of various natures. In the present paper an attempt has been made to obtain two-parameter power Garima distribution using power transformation of Garima distribution which includes one parameter Garima distribution as particular cases. The statistical properties of the distribution including shapes of density for varying values of parameters, the moments, survival function, hazard rate function and mean residual life function have been discussed. The maximum likelihood estimation has been discussed. The goodness of fit of the proposed distribution has been discussed with two real lifetime data set from engineering and medical sciences and fit shows quite satisfactory over other one parameter and two-parameter lifetime distributions.
Power Garima Distribution
Assuming the power transformation X = Y1/α in (1.1), the pdf of the random variable X can be obtained as
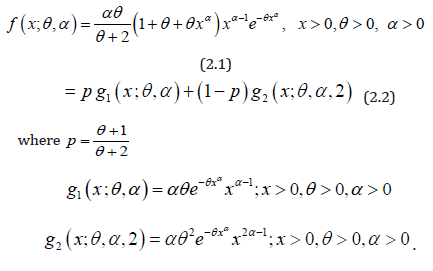
We would call the density in (2.1) as power Garima distribution (PGD) with parameters θ and α , and it is denoted by PGD(α ,θ ) . Like Garima distribution, PGD is also a convex combination of Weibull(θ ,α ) distribution, a generalized gamma(2,α ,θ ) distribution.
The corresponding cdf of PGD can be obtained as


The nature of the pdf of PGD has been studied with the help of the graphs for varying values of parameters and presented in Figure 1.
Further, the nature of the cdf of PGD has also been studied with the help of the graphs for varying values of parameters and presented in Figure 2.
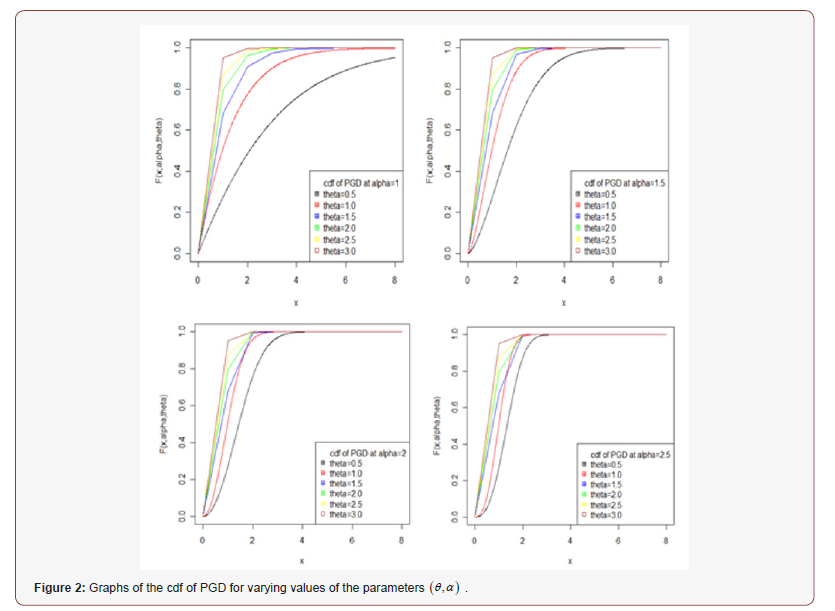
Moments of Power Garima Distribution
Using the mixture representation in (2.2), the rth moment about
the origin,
of PGD can be obtained as
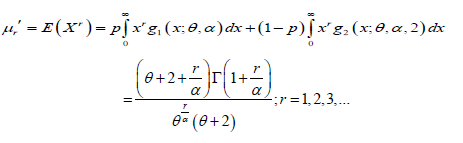
It should be noted that at α =1, the above expression reduces to the rth moment about origin of Garima distribution given by
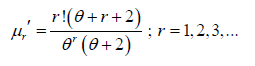
Thus the first four moments about origin of the PGD are thus obtained as
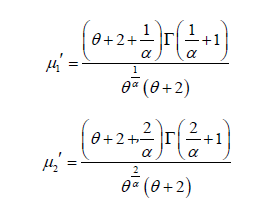
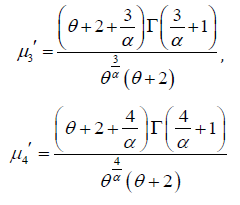
Therefore, the variance of PGD can be obtained as
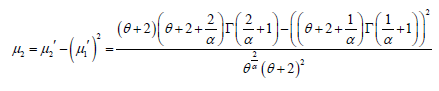
The higher order central moments are not being given here because their expressions are big. However, higher order central moments, if required, can be easily obtained using relationship between moments about mean and moments about origin. Finally, coefficient of variation, skewness, kurtosis and index of dispersion, if needed, can be obtained using their formulae in terms of central moments.
Reliability Properties of Power Garima Distribution
The survival function of PGD can be obtained as

The hazard rate function h(x;θ ,α ) and the mean residual function
m(x;θ ,α ) of PGD are given respectively as:
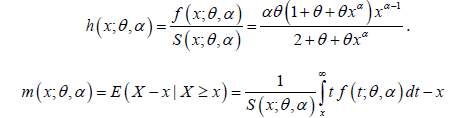
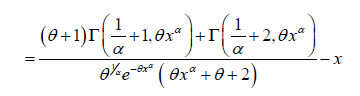
It can be easily verified that at x = 0 it reduces to the expression for the mean of PGD. The nature of hazard rate function of PGD for varying values of parameters has been shown graphically in figure 3. The graphs of hazard rate function of PGD are monotonically increasing for varying values of parameters. Again, the nature of mean residual life function of PGD for varying values of parameters has been shown in figure 4. The graphs of mean residual life function of PGD are monotonically decreasing for varying values of parameters.
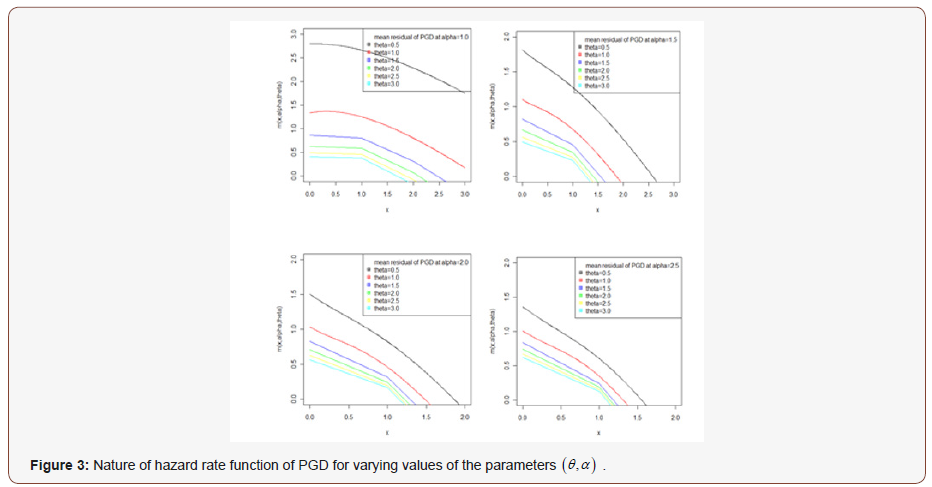
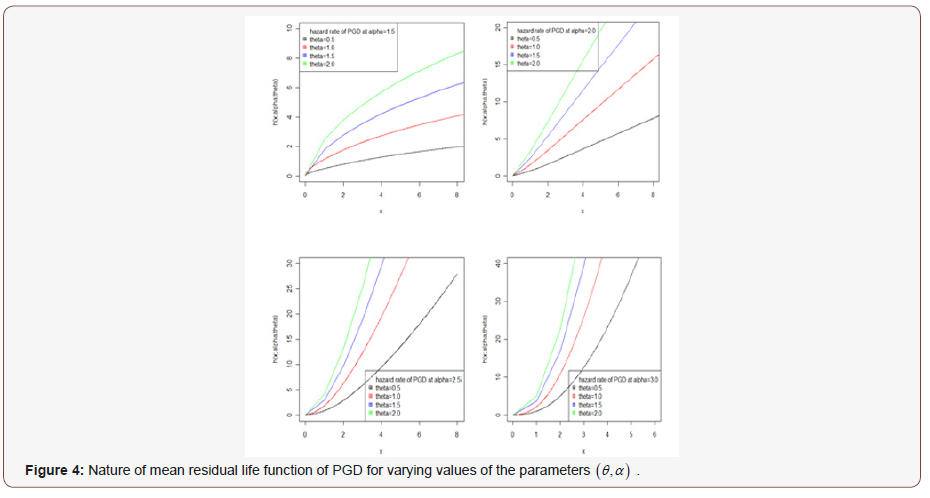
Maximum Likelihood Estimation of Parameters of Power Garima Distribution
Suppose ( x1, x2,.....xn> ) be a random sample of size n From PGD (θ ,α ) The log-likelihood function of PGD can be expressed as
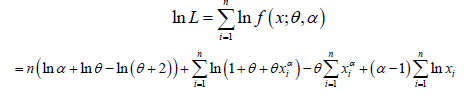
The maximum likelihood estimates of parameters (α ,θ )
of PGD are the solution of the following log-likelihood equations
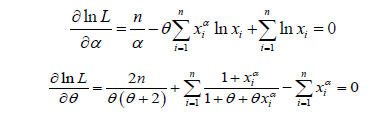
These two natural log likelihood equations do not seem to
be solved directly, because they cannot be expressed in closed
forms. The (MLE’s) of (θ ,α ) can be computed directly by
solving the natural log likelihood equation using Newton-Raphson
iteration using R-software till sufficiently close values of θˆ andαˆ
are obtained.
Goodness of Fit of Power Garima Distribution
In this section, the goodness of fit of PGD using maximum likelihood estimates of parameters has been discussed with two real datasets. The goodness of fit has been compared with other one parameter and two-parameter lifetime distributions.
Data Set 1
The data set represents the strength of 1.5cm glass fibers measured at the National Physical Laboratory, England. Unfortunately, the units of measurements are not given in the paper, and they are taken from Smith & Naylor [9]

In order to compare the considered distributions, values of
MLE along with their standard errors, -2ln L, AIC (Akaike
Information Criterion), K-S (Kolmogorov-Smirnov Statistics)
and p-values for the real lifetime dataset 1 have been computed
and presented in Table1. The best distribution is the distribution
corresponding to lower values of -2ln L, AIC, K-S statistic (Table 1).
Table 1: MLE’s, -2ln L, se, AIC, K-S statistic, and P-value of the
fitted distribution of dataset1.
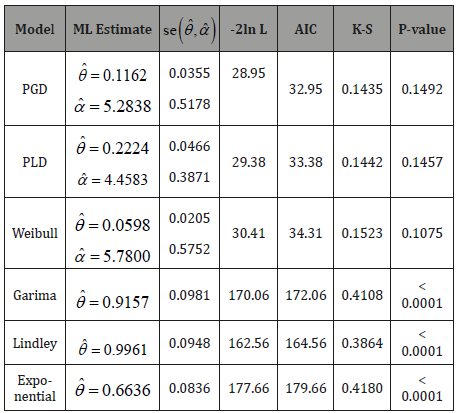
The Variance-Covariance matrix and 95% confidence interval (CI’s) for the parameters
and
of PGD for the given dataset 1 has been presented in
Table 2.
Table 2: Variance-Covariance matrix and 95% confidence interval (CI’s)
for the parameters
and
of PGD.
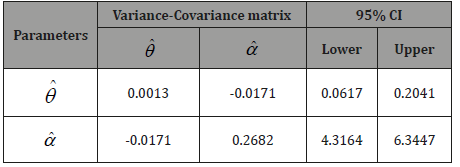
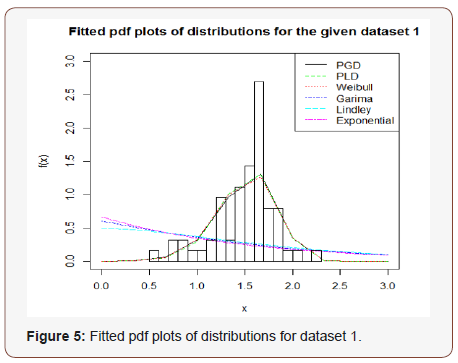
In order to see the closeness of the fit given by one parameter exponential, Lindley and Rama distributions and two-parameter Weibull distribution, PLD and PGD, the fitted plot of pdfs of these distributions for the given dataset have been shown in Figure 5. It is also obvious from the fitted plots of the distributions along with the histogram of the original dataset that PGD gives much closer fit as compared to other considered distributions (Figure 5).
Data Set 2
The following dataset represents waiting time (in minutes) of 65 dental patients, waiting before OPD (Out Patient Diagnosis) from the 25th to 29th December, 2017 at Halibet Hospital, Asmara, available in the master thesis of Berhane Abebe [10], Department of Statistics, College of Science, Eritrea Institute of Technology, Eritrea [11].

The maximum likelihood estimates of parameters, standard error of estimates of parameters, AIC, K-S and p-values of the fitted distributions for dataset 2 are shown in Table 3.
Table 3: MLE’s, -2ln L, se, AIC, K-S statistic, and P-value of the
fitted distribution of dataset2
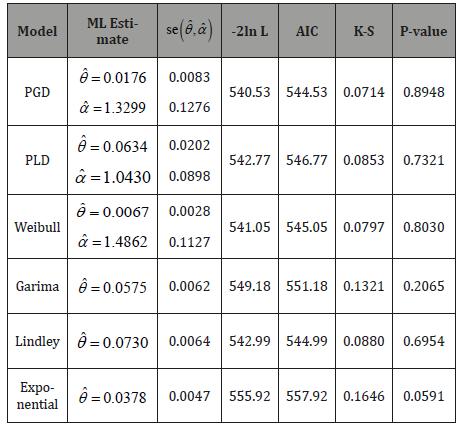
The Variance-Covariance matrix and 95% confidence interval
(CI’s) for the parameters
and
of PGD for the given dataset 2
has been presented in Table 4.
Table 4:Variance-Covariance matrix and 95% confidence interval (CI’s)
for the parameters and
of PGD.
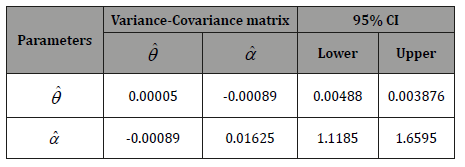
In order to see the closeness of the fit given by one parameter exponential, Lindley and Garima distributions and two-parameter Weibull distribution, introduced by Weibull (1951), PLD and PGD, the fitted plot of pdfs of these distributions for the given dataset have been shown in Figure 6. It is also obvious from the fitted plots of the distributions along with the histogram of the original dataset that PGD gives much closer fit as compared to other considered distributions (Figure 6).
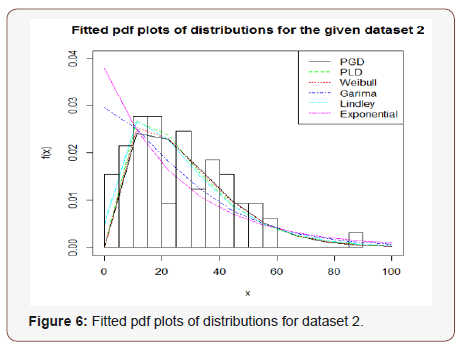
Conclusion
A two-parameter continuous Power Garima distribution (PGD) has been introduced which includes Garima distribution, introduced by Shanker [1], as a particular case. The statistical and reliability properties including shapes of density for varying values of parameters, the moments about the origin, the variance, survival function, hazard rate function, mean residual function of PGD have been discussed. The method of maximum likelihood for estimating the parameters has been discussed. Finally, the goodness of fit of PGD has been discussed with two real lifetime datasets and the fit has been found quite satisfactory as compared to one parameter Garima, Lindley and Exponential distributions and two-parameter Power Lindley distribution (PLD) and Weibull distribution. Therefore, PGD can be considered as an important lifetime distribution for modeling lifetime data from engineering and biomedical sciences.
Acknowledgement
None.
Conflict of Interest
No conflict of interest.
References
- Shanker R (2016) Garima distribution and Its Application to model behavioral science data. Biometrics and Biostatistics International Journal 4(7): 1-9.
- Shanker R (2017) The discrete Poisson-Garima distribution. Biometrics and Biostatistics International Journal 5(2): 1-7.
- Shanker R, Shukla KK (2017a) Size-biased Poisson-Garima Distribution with Applications. Biometrics and Biostatistics International Journal 6(3): 1-6.
- Shanker R, Shukla KK (2017b) Zero-Truncated Poisson-Garima Distribution with Applications. Biometrics and Biostatistics Open access Journal 3(1): 1-6.
- Ghitany ME, Al Mutairi DK, Balakrishnan N, Al-Enezi LJ (2013) Power Lindley distribution and Associated Inference, Computational Statistics and Data Analysis 64: 20-33.
- Lindley DV (1958) Fiducial distributions and Bayes’ Theorem, Journal of the Royal Statistical Society. Series B 20: 102-107.
- Ghitany ME, Atieh B, Nadarajah S (2008) Lindley distribution and its Application, Mathematics Computing and Simulation 78: 493-506.
- Shanker R, Hagos F, Sujatha S (2015) On Modeling of Lifetimes data using Exponential and Lindley distributions. Biometrics & Biostatistics International Journal 2(5): 1-9.
- Smith RL, Naylor JC (1987) A comparison of Maximum likelihood and Bayesian estimators for the three parameter Weibull distribution. Applied Statistics 36: 358-369.
- Berhane A (2018) Discretization of some continuous distribution and Their Applications, Master thesis submitted to Department of Statistics, College of Science, Eritrea Institute of Technology, Asmara, Eritrea.
- Weibull W (1951) A statistical distribution of wide applicability. Journal of Applied Mathematics 18: 293-297.
-
Berhane Abebe, Mussie Tesfay, Tesfalem Eyob, Rama Shanker. A Two-Parameter Power Garima Distribution with Properties and Applications. Annal Biostat & Biomed Appli. 1(3): 2019. ABBA.MS.ID.000515.
Two-Parameter, Power Garima Distribution, Statistics, behavior, Engineering and Medical science, Garima distribution, Moments, Hazard rate function, Mean residual life function, Maximum likelihood estimation, Applications.
-
This work is licensed under a Creative Commons Attribution-NonCommercial 4.0 International License.
- Abstract
- Introduction
- Power Garima Distribution
- Moments of Power Garima Distribution
- Reliability Properties of Power Garima Distribution
- Maximum Likelihood Estimation of Parameters of Power Garima Distribution
- Goodness of Fit of Power Garima Distribution
- Conclusion
- Acknowledgement
- Conflict of Interest
- References