Research Article
Enhancing Virtual Geographic Environments for 3D Geosimulation with Cutting-Edge Urban Visual Building Information Mobile Capturing
Igor Agbossou
University of Franche-Comté, Laboratory ThéMA UMR 6049, IUT NFC, Belfort, France
Igor Agbossou, University of Franche-Comté, Laboratory ThéMA UMR 6049, IUT NFC, Belfort, France
Received Date:November, 14, 2023; Published Date:December 01, 2023
Abstract
The conceptualization and development of Virtual Geographic Environments (VGE) have been instrumental in transcending traditional boundaries in spatial analysis, simulation, and decision making. Over the years, researchers and practitioners have strived to create virtual scenes that mirror the complexities of our physical environment. The applications of VGE extend across diverse domains, from urban planning and environmental science to disaster management and healthcare. In scientific contexts, the integration of cutting-edge technologies becomes paramount in elevating the capabilities of VGEs. This paper presents a comprehensive framework for enhancing VGE through the integration of cutting-edge technologies, particularly focusing on Urban Visual Building Information Mobile Capturing (UVBIMC). By leveraging these advanced techniques, the paper aims to enrich 3D geosimulation models for more accurate and detailed urban planning and analysis. The study showcases the potential benefits of this integrated approach, providing insights into how it can advance the field of urban digital twin science and significantly improve decision-making processes in urban development via the possibilities of simulations and prospective analyzes.
Keywords:3D Geosimulation, Urban Visual Feature; Virtual Geographic Environment; Urban Digital Twin; Photogrammetry
Introduction
In contemporary urban planning and geographic information science, the integration of advanced technologies has become imperative for the development of accurate and detailed geosimulation models. Also, in the realm of spatial analysis, urban simulation, and decision-making, the evolution of Virtual Geographic Environments (VGE) has been transformative, transcending traditional boundaries and opening new avenues for exploration [1, 2]. VGEs serve as dynamic digital counterparts to our physical surroundings, facilitating an indepth understanding of complex spatial relationships. The applications of VGE span diverse domains, encompassing urban planning [3, 4], environmental science [4], disaster management [5], and healthcare [6]. As the demand for more realistic and detailed virtual representations intensifies [79], researchers and practitioners are increasingly integrating cutting-edge technologies into VGE frameworks. This integration becomes particularly critical for addressing the intricate challenges posed by urban environments [10, 12]. This paper introduces a comprehensive framework designed to enhance VGE through the infusion of cutting- edge technologies, with a specific focus on the application of Urban Visual Building Information Mobile Capturing (UVBIMC) which represents a paradigm shift in the capture and integration of visual building information within VGE. Indeed, in the eve revolving landscape of technology, a diverse array of sensors has emerged, offering a comprehensive toolkit for gathering geospatial data in urban environments. This suite of sensors facilitates the capture of multifaceted data types, encompassing spatial coordinates, 3D point clouds, images, and depth information. Among these, the Light Detection and Ranging (LiDAR) scanner stands out as a widely employed instrument for precise data acquisition. Furthermore, the ubiquity of mobile devices, particularly smartphones and tablets, has surged in recent years [13, 14].
These compact yet powerful devices are equipped with sophisticated
cameras and sensors, including RGB cameras and depth
sensors. While the cameras adeptly capture high-resolution images,
the depth sensors offer precise distance measurements from the
sensor to objects within the scene. This harmonious integration of
sensors empowers researchers to capture a rich spectrum of data,
encompassing both visual and depth dimensions, instrumental for
advancing urban modeling efforts. This paper delves into the intricate
details of this technology, exploring its potential to advance
3D geosimulation models. The primary objective is to bolster the
accuracy and granularity of urban planning and analysis, providing
a more nuanced understanding of the urban landscape. So, we endeavor
to achieve four objectives.
1. Integration of Cutting-edge Technologies: Explore and
elucidate the integration of cutting-edge technologies, specifically
UVBIMC, within the framework of VGEs.
2. Enrichment of 3D Geosimulation Models: Showcase how
the integration of advanced techniques contributes to the enhancement
of 3D geosimulation models, enabling more accurate
and detailed representations of urban environments.
3. Advancement of Urban Digital Twin Science: Contribute
to the evolution of Urban Digital Twin science by leveraging the
capabilities of UVBIMC and other innovative technologies.
4. Improved Decision-Making in Urban Development:
Demonstrate how the proposed framework facilitates improved
Decision-Making processes in urban development through simulations
and prospective analyses.
This research holds significant implications for the fields of urban planning, digital twin science, and Decision-Making processes in urban development. By harnessing the potential of UVBIMC and cutting-edge technologies, the paper aims to push the boundaries of what is achievable in the realm of VGEs. The insights garnered from this research are anticipated to catalyze advancements in urban modeling, simulation, and analysis, fostering a more sustainable and informed approach to urban development. The remainder of this paper is structured as follows: in section 2, we provide a Background and Related Works of Virtual Geographic Environments (VGE) and Geosimulation models. This section sets the stage by elucidating the foundational concepts and challenges within the realm of VGEs. We delve into the intricacies of geosimulation models, examining their significance and the limitations they currently face. Additionally, we discuss the critical role of cutting-edge technologies in addressing these challenges and pushing the boundaries of VGE capabilities. Section 3, titled “Materials and Methods,” delves into the specifics of our approach to enhancing VGEs. Here, we focus on the integration of cutting-edge technologies, with particular attention to Urban Visual Building Information Mobile Capturing (UVBIMC). We provide a detailed account of the methodologies, tools, and techniques employed in our study. This section serves as a practical guide for researchers and practitioners interested in replicating or building upon our framework. In section 4, “Experimental Real Application,” we present a real-world application of our proposed enhancements. This section outlines a practical framework for implementing the integration of cutting-edge technologies, showcasing how UVBIMC can be effectively utilized. We explore the potential impact of our framework on urban planning and Decision-Making processes, offering concrete examples and insights derived from the application of our methodology. The final section, Conclusion and Future Directions, synthesizes the key findings of our study. We draw conclusions based on the outcomes of the experimental real application and reflect on the broader implications for the field of VGEs. Furthermore, we outline potential avenues for future research, identifying areas where additional exploration and advancements are needed to continue pushing the boundaries of geosimulation and VGE capabilities.
Background and Related Works
Virtual Geographic Environments and Geosimulation
The dynamic nature of modern urban environments, characterized by rapid population growth, increased spatial complexity, and diverse infrastructural development, has propelled the need for sophisticated tools and methodologies in the domain of geographic information science. VGEs represent a critical paradigm in contemporary geographic information science, enabling the creation of dynamic and interactive representations of real-world spatial environments [15]. These digital environments serve as powerful tools for simulating and analyzing various geographic phenomena [16], providing researchers [17, 19], policymakers [20], and urban planners with the means to explore and understand complex spatial relationships and dynamic processes within urban and natural landscapes [21]. Through the integration of spatial data, remote sensing technologies, and computational modeling [16, 22, 23], VGEs facilitate the development of sophisticated geosimulation models, allowing for the dynamic visualization and analysis of complex spatial systems and their interactions. In the realm of urban planning and design, VGEs have emerged as indispensable tools for stakeholders involved in envisioning and shaping urban landscapes [24]. VGEs facilitate a dynamic and immersive experience that goes beyond traditional planning methods. Stakeholders can navigate through virtual cityscapes, gaining insights into proposed developments, and assessing their impact on the existing environment. This virtual exploration aids in visualizing architectural designs [25] and urban layouts, fostering a more informed Decision-Making process [19].
Architects and city planners can analyze the spatial relationships between buildings, infrastructure, and natural elements, optimizing designs for functionality and aesthetics [25-27]. Applications range from modeling the effects of climate change to understanding the consequences of deforestation and predicting the impact of natural disasters [28, 29]. Researchers can simulate various scenarios to understand how diseases may propagate in different environments, informing public health strategies and resource allocation [26, 30-32]. Through geosimulation within VGEs, researchers can create dynamic models that simulate environmental changes over time. This capability contributes significantly to predictive modeling, enabling scientists to forecast potential outcomes based on varying scenarios. Geosimulation, a key application of VGEs, involves the construction of computational models that simulate and analyze geographical phenomena, including urban growth, transportation networks, environmental changes, and social dynamics [31, 32].
Geosimulation models enable researchers to explore and predict the behavior of complex spatial systems, offering valuable insights into the impacts of various interventions and policy decisions on urban development and environmental management. These models play a crucial role in supporting evidence based Decision-Making processes, providing stakeholders with the necessary tools to assess the potential outcomes of different scenarios and develop informed strategies for sustainable development and resource management. Despite the significant advancements in VGEs and geosimulation methodologies [24-26], the accurate representation of intricate urban landscapes and dynamic spatial processes remains a challenge [15]. In the current decade, the evolution of VGEs continues with a focus on enhanced data integration and interactivity. Recent developments include realtime data feeds and dynamic updates to virtual environments, ensuring that the information within VGEs remains current and relevant. Interactive features have become paramount, allowing users to manipulate and explore the virtual space in realtime [24, 25, 28].
Scientific Challenges for VGEs and Geosimulation
Conventional geosimulation models often encounter challenges in accurately capturing the intricate spatial dynamics and urban complexities that define contemporary cities [26, 28, 30-32]. As such, there exists a pressing demand to enhance the fidelity of VGEs, ensuring a more comprehensive and nuanced representation of real-world urban landscapes. The motivation behind this research stems from the recognition of the limitations in existing geosimulation techniques, underscoring the necessity to incorporate cutting-edge technologies that can facilitate the seamless integration of urban visual building information within virtual environments. Despite the significant progress made in the field of VGEs and geosimulation, several scientific challenges persist, hindering the accurate representation and simulation of complex spatial phenomena. Some of the key challenges and limitations are documented in Table 1.
Table 1:Challenges related to the limitations of current VGEs and Geosimulation.
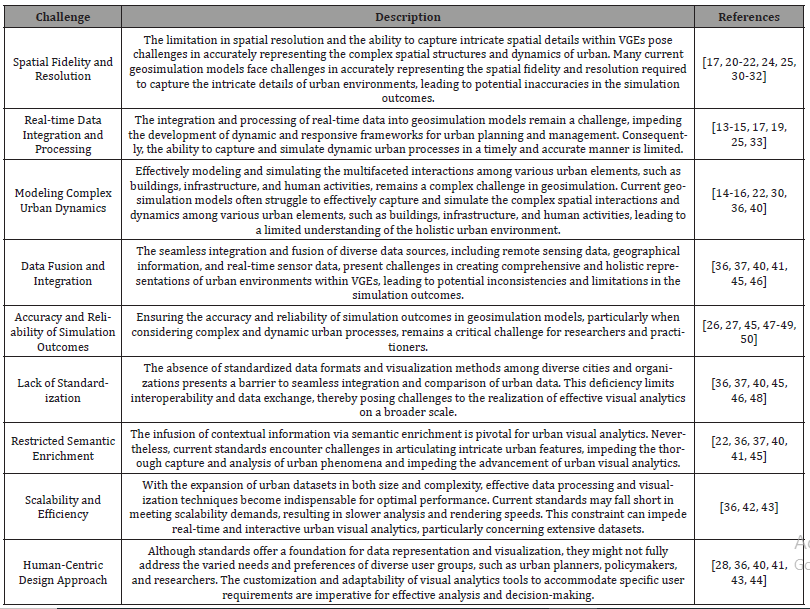
By addressing partially this gap, our study aims to contribute to the advancement of a more robust and refined approach to geosimulation, enabling stakeholders to gain deeper insights into the complex interplay between spatial elements and dynamic urban processes. Furthermore, the integration of mobile capturing techniques allows for the timely acquisition of high-resolution data, fostering a more responsive and adaptive framework for urban planning and management.
Materials and Methods
Urban Visual Building Information Mobile Capturing Technologies
The integration of urban visual building information into VGEs constitutes a pivotal step towards enhancing the accuracy and realism of geosimulation models. Traditional geosimulation models often struggle to represent the intricate details of urban landscapes, and the incorporation of cutting-edge technologies in the form of urban visual building information mobile capturing addresses some of the identified in section 2.2. Advancements in Terrestrial Laser Scanning (TLS), Structure from Motion (SfM) Photogrammetry, Mobile Mapping Systems (MMS), and Unmanned Aerial Vehicles (UAVs) have revolutionized the acquisition of urban visual building information [15, 16, 33-35]. These technologies facilitate the rapid and precise capture of detailed 3D models, enabling the creation of comprehensive representations of the built environment.
TLS has emerged as a pivotal technology for capturing highly detailed and precise 3D building information in urban environments. They facilitate the rapid and accurate acquisition of complex spatial data, enabling the creation of detailed point cloud representations of buildings and structures. This technique employs laser beams to measure the distance to various surfaces, generating dense and accurate 3D point clouds that can be utilized for comprehensive building information modeling and virtual reconstruction. The utilization of TLS in the context of urban visual building information capture has significantly contributed to the enhancement of data acquisition techniques, enabling the creation of more comprehensive and detailed virtual representations of urban environments within geosimulation models [51].
SfM photogrammetry has emerged as a prominent technique for generating accurate 3D models from 2D images, contributing significantly to the advancement of urban visual building information capture. SfM photogrammetry enables the reconstruction of detailed 3D representations of buildings and urban structures by analyzing the structure and motion of various elements captured in a sequence of 2D images [15, 16]. This technology has proven to be cost-effective and efficient, facilitating the rapid acquisition of detailed building information, particularly in situations where access to buildings or structures may be challenging. The integration of SfM photogrammetry within the realm of urban visual building information capture has significantly contributed to the development of accurate and detailed 3D models, fostering the advancement of geosimulation models for enhanced urban planning and analysis.
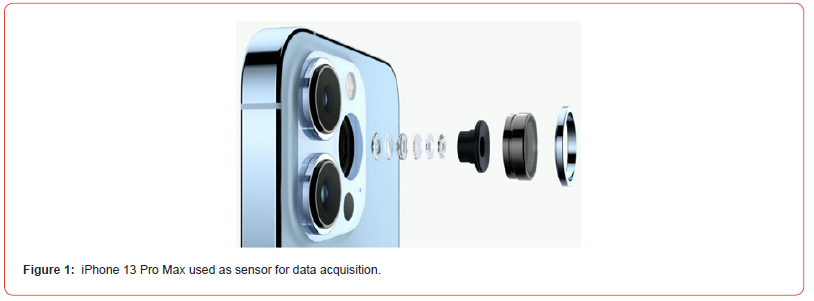
In recent years, there has been a surge in the popularity of mobile sensors, particularly smartphones and tablets [13, 14]. These devices come equipped with sophisticated cameras and sensors, including RGB cameras and depth sensors. The highresolution images captured by cameras, along with distance measurements provided by depth sensors, enable the acquisition of both visual and depth data for urban modeling. The choice of sensor depends on various factors such as specific data requirements, study area scale, budget constraints, and logistical considerations. Researchers need to thoroughly assess the capabilities and limitations of different sensors to ensure accurate and comprehensive data acquisition for augmented reality modeling in urban environments. For our specific data acquisition needs, we opted for the iPhone 13 Pro Max as our sensor of choice, with previous versions of the iPhone Pro family, starting from version 12, also proving suitable. This smartphone model offers advanced features that enhance the quality of captured images. Integrated sensors in modern smartphones provide capabilities aligning with the requirements for data acquisition in photogrammetry, making them well-suited for capturing high-quality images for 3D modeling [52]. Notable features include wide color capture for photos and live photos, lens correction for accurate representations, retina flash for optimal lighting condi tions, auto image stabilization to reduce blurriness, and burst mode for capturing multiple frames rapidly. The amalgamation of these features positions the iPhone 13 Pro Max as an excellent fit for our research purposes. Refer to Figure 1 for a visual representation of the iPhone 13 Pro Max, illustrating its pivotal role in acquiring essential data for our endeavors in UVBIMC.
Methodology and UVBIMC Workflow
Numerous technologies contribute to advancing VGE for 3D Geosimulation systems, and a comprehensive understanding of these technologies is pivotal for designing effective solutions. Computer vision [2, 7, 8, 13-17, 24-27] plays a central role in recognizing and comprehending the physical environment. This involves analyzing visual data [36, 53, 26], such as images or video streams, to detect and track objects, estimate their pose, and extract relevant features [42, 43]. The implementation of computer vision algorithms facilitates the seamless registration of virtual objects into the real world, enabling integration and interaction. The creation of accurate and detailed 3D models of the urban environment is foundational for realistic and contextually relevant augmentations. Techniques like laser scanning [16], photogrammetry [15, 16], and CAD modeling contribute to generating high-fidelity 3D models [8, 38]. These models capture both geometric and semantic information about buildings, streets, and other urban elements, forming the basis for precise and visually consistent augmentations [19, 26, 27]. Machine learning techniques [15, 16, 24, 25] are integral to the process, enabling object recognition, semantic understanding, and real-time tracking. These algorithms can be trained to recognize and classify urban objects, facilitating intelligent augmentations and predictive analysis [54, 55]. Additionally, AI based algorithms can adapt and improve over time, enhancing the accuracy and effectiveness of enhanced VGE for 3D Geosimulation systems. Stakeholders in urban planning and design can leverage enhanced VGE and urban visual building information to visualize and analyze proposed changes to the built environment in real-time. Refer to Figure 2 for an illustration of the different components involved in this intricate process.
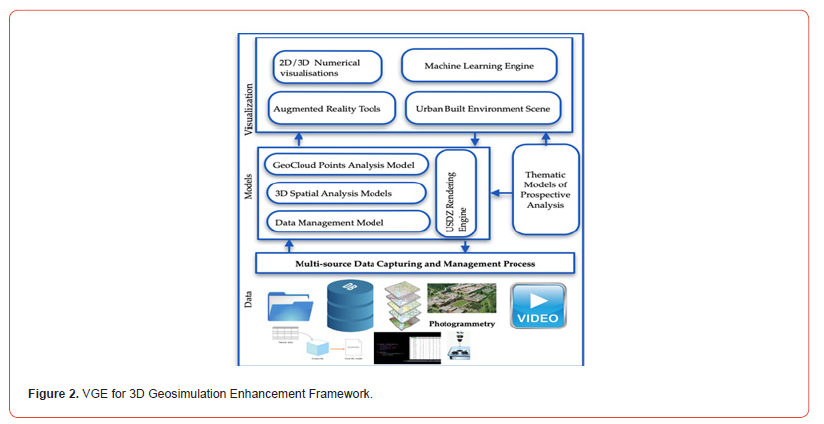
The integration of cutting-edge urban visual building information into VGEs necessitates the development of a robust Geosimulation model enhancement Framework. This framework outlines a systematic approach for incorporating advanced technologies, ensuring the creation of dynamic and accurate geosimulation models. The steps and methodological workflow required to implement such a system are summarized in Table 2, (Figures 3-5).
Table 2:Steps and methodological workflow required to implement an enhanced VGE.
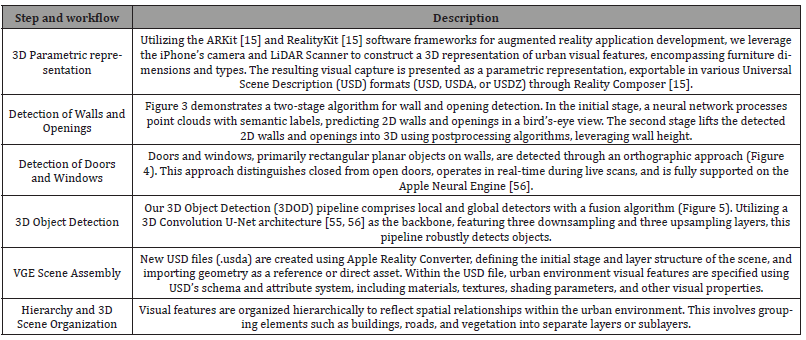
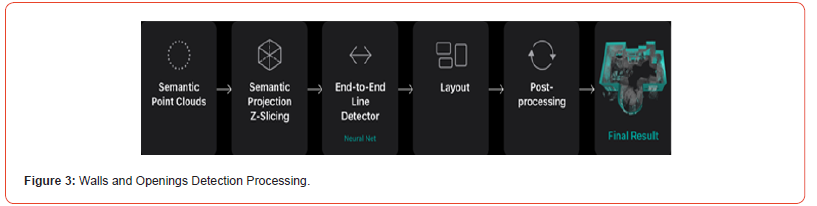
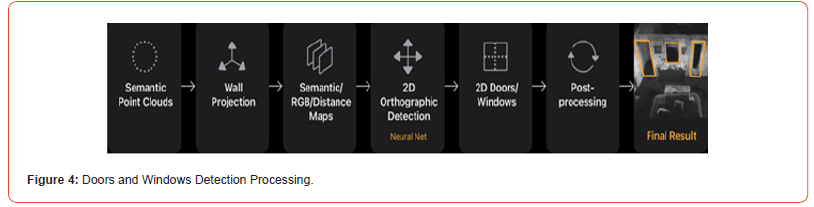
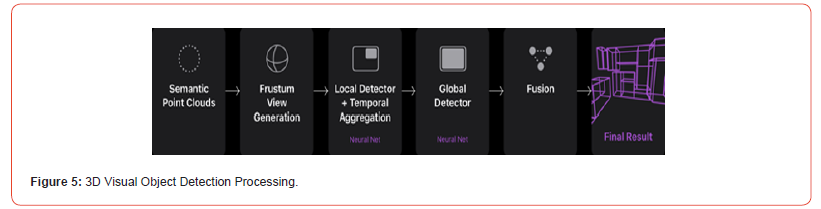
Experimental Real Application
Experimental Urban Area
In this experimental investigation, our VGE for 3D Geosimulation Enhancement Framework, coupled with the defined methodological workflow, was deployed in a section of the recently developed residential area known as “Jardins du MONT” in Belfort, France (Figure 6). This housing estate project encompasses 25 plots featuring individual houses ranging from 600 to 900 m2. Notably, “Jardins du MONT” represents a contemporary development characterized by high-quality architectural design. Conveniently located, it is within a 10minute travel distance from the city center of Belfort via car, bus, or bike. Moreover, it enjoys proximity to the vibrant “Techn’Hom” business park, housing major companies such as GE and Alstom. This strategic location offers residents a tranquil and green urban setting, affording exceptional views of Belfort and its fortifications. The primary focus of our research in this study revolves around 3D spatial analysis, the temporal evolution of new housing estates, and the practical implementation of smart city concepts utilizing advanced tools in artificial intelligence. Given the dynamic nature of this urban area’s ongoing development, the application of our enhanced VGE was deemed crucial to conducting a prospective analysis of the evolving urban built environment.
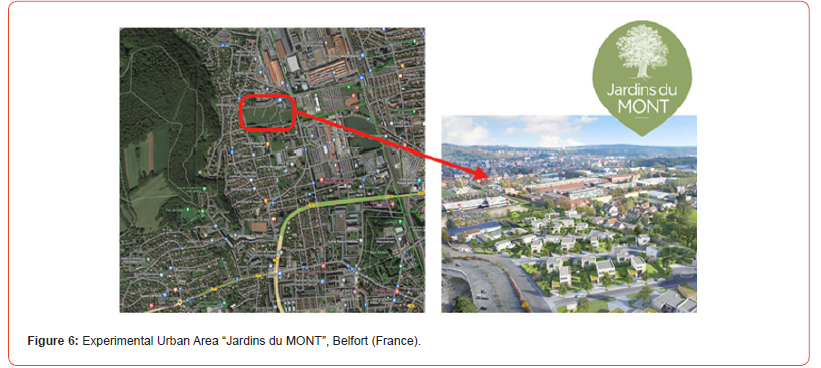
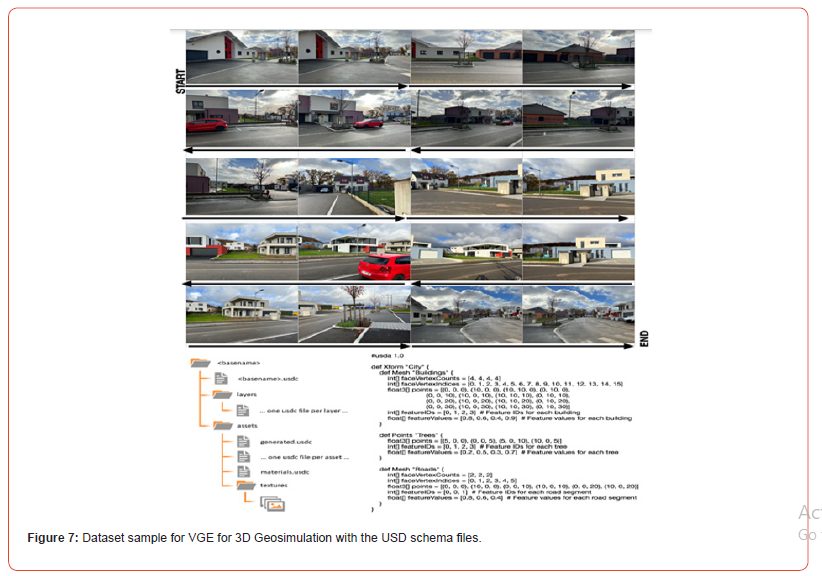
In the pursuit of accommodating the diverse visual characteristics of various urban residences, including houses, apartments, and other dwellings, a comprehensive database was meticulously compiled. This database, integral to our algorithmic developments, encompasses 800 photos capturing a hundred distinct houses. Rigorous adherence to overlap constraints was maintained during the data collection process to ensure the robustness of our algorithms. The database is curated with 799 calibrated image pairs, meticulously organized based on stereovision image matching constraints. To enrich the dataset and enhance the adaptability of our algorithms to different scanning patterns, we also recorded a set of video sequences. These sequences, captured using an iPhone and iPad, aimed to comprehensively cover all surfaces of the structures. Notably, each sequence followed a distinct motion pattern, contributing to the algorithm’s resilience across varied scan patterns. Figure 7 provides a visual representation of the captured data, illustrating the reading direction of the photos from start to end. It’s essential to note that the number of pictures required for achieving an accurate 3D representation varies, contingent upon factors such as the image pair quality, the complexity of the built environment, and its size.
Enhanced VGE for 3D Geosimulation with UVBIMC Implementation
Our research introduces a framework for enhancing VGE through the integration of cutting-edge technologies, with a particular emphasis on UVBIMC. This section delves into the practical implementation of our approach, highlighting key components and their impact on the fidelity of VGE and 3D geosimulation models. The backbone of our approach lies in the meticulous collection and processing of data. Leveraging UVBIMC involves the use of advanced tools. Simultaneously, mobile devices record video sequences, employing varied motion patterns to ensure robustness in our algorithm’s response to different scan patterns. Ground truth information is derived from labeled data, aligning it with reconstructed scenes to ensure the accuracy of our 3D representations. Our framework is not confined to theoretical concepts; it is robustly implemented in real-world settings. This practical application underscores the framework’s efficacy and feasibility in diverse urban environments. The seamless integration of UVBIMC showcases the practicality of our approach, setting the stage for its adoption in real-world scenarios. The real-world application of our framework goes beyond technical implementation. It explores the tangible impact on urban planning and Decision-Making processes. By providing a more accurate and detailed representation of the urban environment, our enhanced VGE becomes a valuable tool for informed Decision-Making in urban development. Figure 8 illustrates the transformative potential of our approach, depicting the enhanced VGE contributing to a more nuanced understanding of the urban landscape.
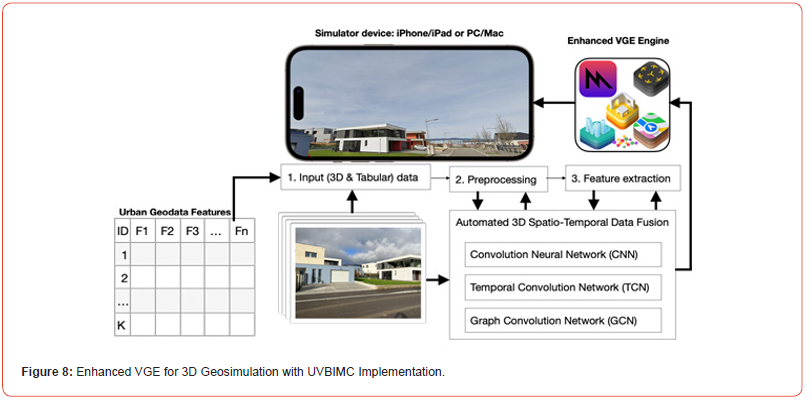
Conclusion and Future Work
In this paper, we presented a comprehensive framework for enhancing VGE through the integration of cutting-edge technologies, with a specific focus on UVBIMC. Our approach, grounded in the mobile device captures, has demonstrated significant advancements in the fidelity of VGE and the enrichment of 3D geosimulation models.
About key findings, our research contributes to the field by addressing the challenges in VGE and geosimulation models. The integration of UVBIMC provides a more detailed and accurate representation of the urban environment, surpassing traditional approaches. Key findings include the seamless alignment of labeled data with reconstructed scenes, ensuring the reliability of 3D representations, and the real-world application showcasing the practicality and effectiveness of our framework. In term of impact on urban planning and Decision-Making, the enhanced VGE resulting from our framework has significant implications for urban plan ning and Decision-Making processes. The detailed and realistic 3D geosimulation models offer a nuanced understanding of the urban landscape, empowering stakeholders to make informed decisions. The potential benefits extend to urban development scenarios, providing a valuable tool for simulating and analyzing prospective changes.
While our framework marks a substantial leap forward, there
are avenues for further exploration and refinement.
1. Integration with Emerging Technologies: Explore the integration
of our framework with emerging technologies such as
augmented reality and virtual reality to enhance user interaction
and engagement in urban planning processes.
2. Semantic Understanding: Enhance the semantic understanding
of captured scenes to enable more intelligent analysis
of urban features, supporting applications in fields like autonomous
navigation and environmental monitoring.
3. Scalability and Accessibility: Investigate methods to optimize
the scalability and accessibility of our framework, ensuring
its applicability in a broader range of urban settings and
making it accessible to a wider audience of urban planners and
decisionmakers.
4. Longitudinal Studies: Conduct longitudinal studies to assess
the long-term impact and sustainability of our enhanced
VGE in supporting urban development decisions.
Our research provides a foundation for advancing the capabilities of VGE and 3D geosimulation models through the integration of UVBIMC. The real-world application demonstrates the transformative potential of our approach in informing urban planning decisions. As we move forward, the identified future directions will guide further research to unlock the full potential of enhanced VGE for sustainable and informed urban development.
References
- Hui Lin, Min Chen, Guonian Lu, Qing Zhu, Jiahua Gong, et al. (2013) Virtual Geo-graphic Environments (VGEs): A New Generation of Geographic Analysis Tool, Earth-Science Reviews 126: 74-84.
- Goodchild MF, (2009) Virtual Geographic Environments as collective constructions. In: Lin, H., Batty, M. (Eds.), Virtual Geo-graphic Environments. Science Press, Beijing pp. 15-24.
- Goodchild MF, (2009) Geographic information systems and science: today and tomorrow. Annals of GIS 15(1): 3-9.
- Lin H, Zhu Q, (2006) Virtual Geographic Environments. In: Zlatanova, S, Prosperi, D. (Eds.), Large-scale 3D Data Integration: Challenges and Opportunities. Taylor & Francis, Boca Raton pp. 211-230.
- Lu GN (2011) Geographic analysis-oriented Virtual Geographic Envi-ronment: framework, structure and functions. Science China-Earth Sci-ences 54(5): 733-743.
- Xu BL, Lin H, Chiu LS, Hu Y, Zhu J, et al. (2011) Col-laborative Virtual Geographic Environments: a case study of air pollution simulation. The Information of the Science 181(11): 2231-2246.
- Bonczak B, Kontokosta CE (2019) Large-scale parameteriza-tion of 3D building morphology in complex urban landscapes using aerial LiDAR and city administrative data. Computers, En-vironment and Urban Systems 73: 126-142.
- Biljecki F, Ledoux L, Stoter J, Vosselman G, (2016) The variants of an LOD of a 3D building model and their influence on spatial analyses, ISPRS Journal of Photogrammetry and Remote Sens-ing 116: 42-54.
- Li L, Tang L, Zhu H, Zhang H, Yang F, Qin W (2017) Semantic 3D modeling based on CityGML for ancient Chinese- style archi-tectural roofs of digital heritage. ISPRS Int J Geo-Inf 6(5) 132.
- Ledoux H (2018) val3dity: validation of 3D GIS primitives ac-cording to the international standards. Open geospatial data, softw. Stand 3(1).
- Sinyabe E, Kamla V, Tchappi I, Najjar Y, Galland S (2023) Shapefile-based multi-agent geosimulation and visualization of building evacuation scenario, Procedia Computer Science 220: 519-526.
- Biljecki F, Ledoux H, Stoter J (2017) Generating 3D city models without elevation data. Computers, Environment and Urban Systems 64: 1-18.
- OV Gnana Swathika, K Karthikeyan, Sanjeevikumar Padmanaban (2022) Smart Buildings Digitalization. Case Studies on Data Centers and Automation. CRC Press 314p.
- Agbossou I (2023) Urban Augmented Reality for 3D Geosimu-lation and Prospective Analysis. In: Pierre Boulanger. Applica-tions of Augmented Reality - Current State of the Art. [Working Title] Intech Open.
- Agbossou, I (2023) Fuzzy photogrammetric algorithm for city built environment capturing into urban augmented reality mod-el. Advances in Fuzzy Logic Systems, IntechOpen.
- You L, Lin H, (2016) A Conceptual framework for virtual geographic environments Knowledge engineering, Int. Arch. Photogramm. Remote Sens Spatial Inf Sci XLI-B2: 357-360.
- Apple AR Kit (2017) More to explore with ARKit.
- Wang ZB, Ong SK, Nee AYC (2013) Augmented reality aided interactive manual assembly design. Int J Adv Manuf Technol 69: 1311-1321.
- Henderson P, Ferrari V (2020) Learning single-image 3D re-construction by generative modelling of shape, pose and shading. Int J Comput Vis 128: 835-854.
- Benenson I, Torrens P. (2002) Geosimulation: Automata-Based Modelling of Urban Phenomena. Chichester: Wiley.
- Li L, Tang L, Zhu H, Zhang H, Yang F, Qin W (2017) Semantic 3D modeling based on CityGML for ancient Chinese- style archi-tectural roofs of digital heritage. ISPRS Int. J. Geo-Inf 6(5): 32.
- Pharr M, Jakob W, Humphreys G (2017) Physically Based Ren-dering, Edition Morgan Kaufmann.
- Zheng Y, Capra L, Wolfson O, Yang H (2014) Urban computing: Concepts, methodologies, and applications. ACM Trans. Intell. Syst. Technol. 5(3) 55.
- Rao J, Qiao Y, Ren F, Wang J, Du Q (2017) A Mobile Outdoor Augmented Reality Method Combining Deep Learning Object Detection and Spatial Relationships for Geovisualiza-tion. Sensors 17(9): 1951.
- Huang MQ, Ninić J, Zhang QB (2021) BIM, machine learning and computer vision techniques in underground construction: Current status and future perspectives’, Tunnelling and Under-ground Space Technology pp. 108.
- Claudia M, Jung T (2018) A theoretical model of mobile aug-mented reality acceptance in urban heritage tourism, Current Issues in Tourism, 21(2): 154-174.
- Gautier J, Brédif M, Christophe S (2020) Co-Visualization of Air Temperature and Urban Data for Visual Exploration, in 2020 IEEE Visualization Conference (VIS), Salt Lake City, UT, USA : pp. 71-75.
- Bazzanella, Liliana. Caneparo, Luca. Corsico, Franco. Roccasalva, Giuseppe. (Eds) (2011). Future Cities and Regions. Simulation, Scenario and Visioning, Governance and Scales. New York, Hei-delberg Springer, USA.
- Batty M (2005) Cities and complexity. MIT Press: Cambridge, USA.
- Batty M, Torrens P (2005) Modelling and prediction in a com-plex world. In: Futures 37: 745-766.
- Portugali J (2000) Self-organization and the city. Springer-Verlag: New York Pumain, D.; Sanders, L.; Saint-Julien, T., 1989, Villes et auto-organisation. Economica, Paris.
- Smith MW, Carrivick JL, Quincey DJ (2016) Structure from motion photogrammetry in physical geography. Progress in Physical Geography: Earth and Environment 40(2): 247-275.
- Grešla O, Jašek P (2023) Measuring Road Structures Using A Mobile Mapping System, Int. Arch. Photogramm. Remote Sens Spatial Inf Sci 43-48.
- Elhashash M; Albanwan H, Qin R (2022) A Review of Mobile Mapping Systems: From Sensors to Applica-tions. Sensors 22: 4262.
- (2023) USDZ : Interopérabilité 3D autour du format de Réalité Augmen-tée.
- (2022) OGC CityGML 30 Conceptual Model.
- Liao T (2020) Standards and Their (Recurring) Stories: How Augmented Reality Markup Language Was Built on Stories of Past Standards. Science, Technology, & Human Values 45(4): 712-737.
- Jung J, Hong S, Yoon S, Kim J, Heo J (2016) Automated 3D wireframe modeling of indoor structures from point clouds us-ing constrained least-squares adjustment for as-built BIM. Jour-nal of Computing in Civil Engineering 30(4).
- Kutzner T, Chaturvedi K, Kolbe TH (2020) City GML 3.0: New Func-tions Open Up New Applications. PFG J. Photogramm. Remote Sens Geoinf Sci 88: 43-61.
- Agugiaro G, Benner J, Cipriano P, Nouvel R (2018) The Energy Application Domain Extension for CityGML: enhancing interop-erability for urban energy simulations. Open geospatial data softw stand 3(2).
- Bonczak B, Kontokosta CE (2019) Large-scale parameteriza-tion of 3D building morphology in complex urban landscapes using aerial LiDAR and city administrative data. Computers, En-vironment and Urban Systems73: 126-142.
- Zheng Y, Wu W, Chen Y, Qu H, Ni L (2016) Visual Analytics in Urban Computing: An Overview in IEEE Transactions on Big Data 2(3) 276-296.
- Li C, Baciu G, Wang Y, Chen J, Wang C (2022) DDLVis: Real-time Visual Query of Spatiotemporal Data Distribution via Densi-ty Dictionary Learning" in IEEE Transactions on Visualization & Computer Graphics, 28(1): 1062-1072.
- Kalogianni E, van Oosteom P, Dimopoulou E, Lemmen C (2020) 3D land administration: A review and a future vision in the context of the spatial development lifecycle. ISPRS Int J Geo-Inf 9(2): 107.
- Stoter J E, Ohori GA, Dukai B, Labetski A, Kavisha K (2020) State of the Art in 3D City Modelling: Six Chal-lenges Facing 3D Data as a Platform. GIM International: the worldwide magazine for geomatics 34.
- Wassermann B, Kollmannsberger S, Bog T, Rank E (2017) From geometric design to numerical analysis: A direct approach using the Finite Cell Method on Constructive Solid Geometry, Computers & Mathematics with Applications 74(7): 1703-1726.
- Ming H, Yanzhu D, Jianguang Z (2016) A topological ena-bled three-dimensional model based on constructive solid ge-ometry and boundary representation. Cluster Comput 19: 2027-2037.
- Kang TW, Hong CH (2018) IFC-CityGML LOD mapping automation using multiprocessing-based screen-buffer scanning including mapping rule. KSCE J Civ Eng 22:373-383.
- Zlatanova S, Rahman AA, Shi W (2004) Topological models and frameworks for 3D spatial objects. Computers & Geos-ciences 30(4): 419-428.
- Liu J, Azhar S, Willkens D, Li B (2023) Static Terrestrial Laser Scanning (TLS) for Heritage Building Information Modeling (HBIM): A Systematic Review. Virtual Worlds 2: 90-114.
- Cherdo L (2019) The 8 Best 3D Scanning Apps for Smartphones and IPads in 2019.
- Weinmann M (2013) Visual Features - From Early Concepts to Modern Computer Vision. In: Farinella G., Battiato S., Cipolla R. (eds) Advanced Topics in Computer Vision. Advances in Com-puter Vision and Pattern Recognition. Springer, London.
- Agbossou I (2023) Urban Resilience Key Metrics Thinking and Computing Using 3D Spatio-Temporal Forecasting Algorithms. In: Gervasi, O., et al. Computational Science and Its Applications - ICCSA 2023. ICCSA 2023. Lecture Notes in Computer Science 13957: pp 332-350.
- T Song, F Meng, A Rodríguez-Patón, P Li, P Zheng (2019) U-Next: A Novel Convolution Neural Network With an Aggregation U-Net Architecture for Gallstone Segmentation in CT Images," in IEEE Access 7: pp. 166823-166832.
- Gwak J, Choy C, Savarese S (2020) Generative Sparse Detec-tion Networks for 3D Single-Shot Object Detection. In: Vedaldi, A., Bischof, H., Brox, T., Frahm, JM. (eds) Computer Vision - ECCV 2020. ECCV 2020. Lecture Notes in Computer Science vol 12349. Springer, Cham.
- Apple Neural Engine Transformers GitHub. 2022
-
Golam Rabbani*, Anindita Hridita, Johayer Mahtab Khan and Khandker Tarin Tahsin. Exploring Climatic Hazards and Adaptation Responses to Address Problems of Climate Migrants in Selected Urban Areas in Bangladesh. Online J Ecol Environ Sci. 1(4): 2023. OJEES. MS.ID.000517.
-
Climate migrants, Vulnerabilities, Adaptation, CBF, Bangladesh, Population movement, Climatic hazards, Urban areas, Storms, Cyclones, Floods, Natural disasters`
-
This work is licensed under a Creative Commons Attribution-NonCommercial 4.0 International License.