Research Article
Artificial Intelligence Techniques Used for Analyzing the Voice of the Customer in the Quality 4.0 Era: A Systematic Review Using PRISMA Protocol
Cintia Zuccon Buffon1, Elizabeth A Cudney2, Ahmad Elshennawy3* and Sandra Furterer4
1Industrial Engineering and Management Systems, University of Central Florida, Florida, USA
2John E. Simon School of Business, Maryville University, St. Louis, Missouri, USA
3Industrial Engineering and Management Systems, University of Central Florida, Florida, USA
4Integrated Systems Engineering, Ohio State University Systems, University of Central Florida, Florida, USA
Ahmad Elshennawy, Industrial Engineering and Management Systems, University of Central Florida, Florida, USA
Received Date:January 22, 2024; Published Date:February 23, 2024
Abstract
This systematic literature review uses the Preferred Reporting Items for Systematic Reviews and Meta-Analyses (PRISMA) protocol. The PRISMA
for abstracts checklist suggests the following structure to be followed for the abstract portion of the study.
Background: Analyzing the voice of the customer (VOC) using AI techniques has been crucial for organizations in different areas in the industry
4.0 era. Traditional ways of gathering VOC can be misleading, costly, and time-consuming. In this new digital era, customers share information
about their needs and wants in social media posts and online reviews, which necessitates a more efficient way of analyzing the enormous amount of
customer-generated data. Therefore, automated techniques such as artificial intelligence, machine learning, data mining, and others have been used
to analyze the VOC for product development and design and process improvements.
Objectives: This study provides a literature review of articles related to using AI techniques to analyze the VOC to improve the quality of
products in the Quality 4.0 era. The main goal is to discuss what has been used in this area and address gaps for future work.
Methods: The PRISMA statement was used in this systematic literature review, where research questions were defined, and the search strategy
and eligibility criteria were developed to answer the research questions. This study searched six different databases and used peer-reviewed articles
published after 2010.
Results: The study discusses 30 journal articles using AI techniques to analyze the VOC. Five main categories of techniques were used in this
study to classify the articles, including supervised machine learning, unsupervised machine learning, data mining, natural language processing, and
sentiment analysis. Some articles did not fall into any specific category and were categorized as others. The results show ongoing research in this
area. However, these studies focus on using the techniques rather than on Industry 4.0 or Quality 4.0.
Conclusions: This review summarizes the techniques used to analyze the VOC. This study was able to answer the research questions posed and
provide direction for future work. The study concluded that AI techniques such as machine learning, data mining, natural language processing, and
sentiment analysis are used to analyze the large amount of data generated by the VOC in the Industry 4.0 era. It was also able to identify that the main
gap in the area is to relate those techniques to Quality 4.0 and improvements in Industry 4.0.
Introduction
Over the years, the use of technology has increased dramatically. Technology and smart technology are becoming increasingly present in our society every day. The market has become more competitive, and customers are becoming more demanding. Therefore, the analysis of the voice of the customer (VOC) has become critical to any organization that wants to succeed with its products or services. Companies must gather and analyze the VOC to be able to understand what their needs are. This process can be time-consuming, costly, and sometimes misleading.
Customers generate content about products and services online in the digital era. Data generated online provides valuable information to organizations wanting to retain customers and expand their market. Automated techniques are the solution for analyzing large amounts of data generated daily online. From social media posts to online reviews, those text data are full of significant content that can help companies design and improve their products better.
This research aims to systematically review the use of automated technologies to analyze the VOC in the Quality 4.0 era. Quality 4.0 is the use of automated technologies to improve the quality of products and services in the fourth industrial revolution [1]. Automated technologies considered in this study are the techniques related to artificial intelligence (AI), big data, machine learning, data mining, and deep learning.
The present study divides the selected articles into categories to examine better the techniques used. Machine learning is divided into supervised learning and unsupervised learning. The main difference between the two is that supervised learning needs labeled data to learn from, while unsupervised learning does not need labeled data [2]. Machine learning is englobed in AI, but they are not synonymous.
The other categories are data mining, natural language processing (NLP), and sentiment analysis. Those are all part of AI as well. Data mining is used to uncover patterns in data [3]. NLP studies use computers to understand text data and human language [4]. Sentiment analysis uses NLP and other areas of machine learning to understand the sentiment behind text data [5]. The category “others” was created to include publications that used different AI techniques or emphasized different techniques.
This review consists of seven sections. Section 3 provides the methodology used in this study. Section 4 discusses the results obtained from the methodology used. Section 5 presents a thorough literature review of 30 publications. Section 6 contains the conclusions and future work. The last section of the study presents the references used to produce this study.
Methodology
This systematic review followed the Preferred Reporting Items for Systematic Reviews and Meta-Analyses (PRISMA) protocol. The PRISMA statement consists of a checklist containing 27 items and a flow diagram, as explained by Moher [6]. The protocol helps researchers produce better systematic reviews and guarantee the quality of the work proposed. Two phases can be identified during the systematic literature review when using PRISMA: formulation of research questions and the search criteria with the collection and analysis of the studies selected [7]. The research questions guide the research to identify what is essential to be studied in that field and address the contribution the study will provide. It helps researchers define the study’s objective and delimit the topic. The search strategy to collect the articles is another critical point when performing a systematic literature review. At this step, key search terms are determined to structure the search (Library, n.d.). At this stage, it is also important to identify the eligibility criteria for the study, determining whether the results from the search are appropriate to the type of study being conducted. It helps the researchers decide what publications should be included in their study.
The following research questions were determined for this
study:
RQ1: How are AI techniques being used to improve the process
of product development in the industry 4.0 era?
RQ2: What are the leading AI techniques being used in the field
to improve the analysis of the VOC?
RQ3: What gaps need to be filled so those techniques can
be more widely used to improve understanding of customers’
requirements in the Quality 4.0 era?.
Search Strategy and Eligibility Criteria:
At the beginning of this study, it was decided to use different
key terms to search different databases for studies related to AI
techniques to analyze the VOC. Synonyms and related terms were
considered. For example, instead of just searching for “voice of
the customer,” terms such as “customers’ requisites,” “customer
feedback,” and “customer experience” were used. The search
using those terms provided many results; however, while scanning
through them, it was found that they were mostly unrelated to the
topic of this study. Therefore, the strategy changed to using only
precise search terms. The combination of specific search terms
resulted in the best search results where most articles were related
to the research topic discussed in this study. The search function
used in all databases was the following: (“machine learning” OR
“AI”) AND “voice of the customer”.
This study searched peer-reviewed journal articles from 2010 to the present year. Since this topic is relatively new, older studies would not provide relevant information for this research. Only peer-reviewed articles were included in this research. Therefore, conference proceedings, books, or other types of grey literature were excluded from this study. Five different databases were used in this study. ProQuest, EBSCOhost on Applied Science & Technology Source, Engineering Village, IEEE, and Web of Science were used to gather publications relevant to this topic. In addition to these databases, the University of Central Florida (UCF) Library Primo Search was also used to improve the number of valid publications.
Other criteria were used to narrow down the number of publications to be considered. The articles must have been written in English and used AI techniques to analyze the VOC. Since the primary purpose of this systematic review was to analyze the AI techniques used to develop better processes related to the VOC, the search was focused on generating results that truly represented the topic. The articles from the searches were screened based initially on their title and abstracts. If the article was considered consistent with the topic, it was selected for further analysis of the full-text content. If the article was not related to the specific topic of this study, it was excluded from the search.
Risk of bias could occur during the selection of the publications; therefore, the risk of bias must be considered when performing a systematic review using the PRISMA protocol. Some other points are also considered in the PRISMA checklist whenever data from the articles are collected. Some topics discussed in the checklist are non-applicable for this study and are not addressed in this research.
Results
The PRISMA protocol uses a flow diagram to help illustrate the different phases of the literature review. This flow diagram is a visual tool to show the search results proposed in section 3. The flow diagram generated for this study is available in Figure 1. In total, 207 publications were retrieved from the six databases with existing filters. Only peer-reviewed articles published after 2010 were retrieved. Due to the inclusion constraints, the final number of publications included in this literature review is 30 (Figure 1).
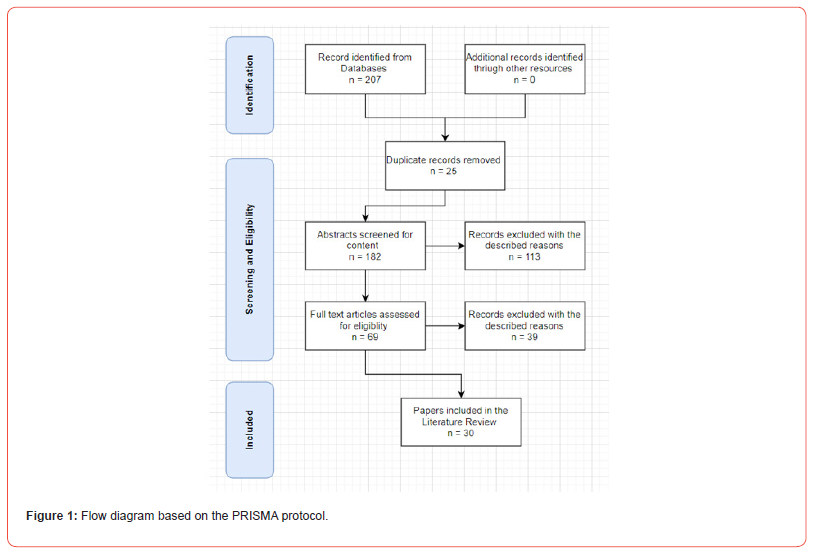
Even though machine learning and AI topics have been around for quite some time, only in recent years has the expansion of those technologies in areas different from purely computer science started. Quality 4.0 and Industry 4.0 are relatively new topics. Therefore, the publications chosen were published after 2010. As shown in Figure 2, the first year of publications retrieved is 2011, which is befitting with the start of that topic with the fourth industrial revolution (Figure 2).
The 30 publications used in this study were published in 27 different journals. Those journals are from four different areas: engineering, AI, marketing, and quality. The publications differ also in the number of authors, as shown in Figure 3. The publications are primarily from one, two, or three authors; however, some publications have four or five authors. This finding demonstrates that in those areas, a smaller group of people are usually involved in working on publications than in other areas such as psychology or human sciences (Figure 3).
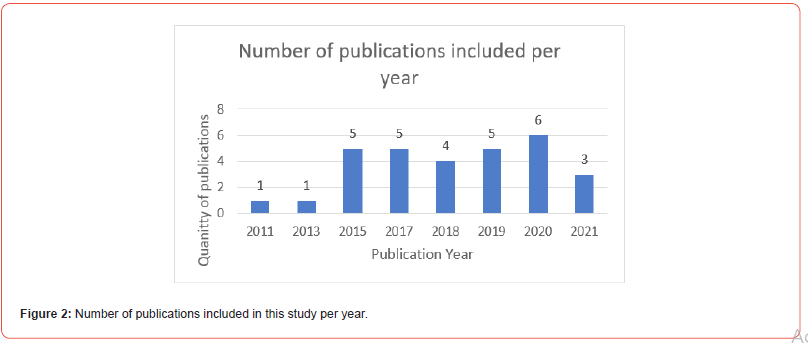
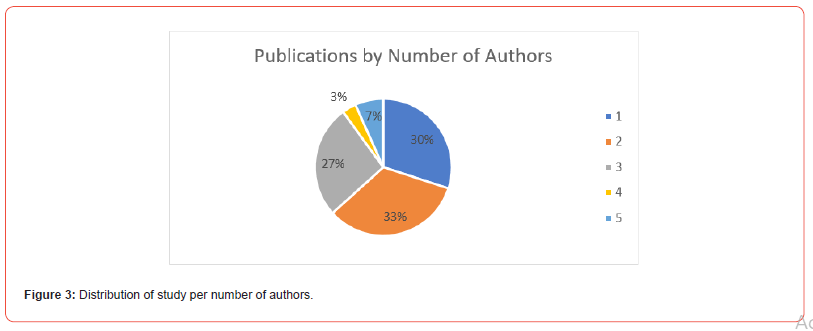
AI techniques are used to improve how the VOC is considered throughout development. The results demonstrated in Figures 1 and 2 indicate that this study area has yet to be researched as much as other areas related to quality, machine learning, and AI. During the search, it was also noted that a literature review posing the same questions as this study did not exist. Other areas, such as marketing, have been exploring the use of those technologies but not from a quality perspective, as this study proposed.
Literature Review
After reviewing the search results and analyzing the publications, it was possible to classify them into six categories related to the type of technique used, proposed, or discussed in the study. The categories were created to facilitate the literature review of the publications and help discuss the use of AI techniques in the context of the VOC. The categories are unsupervised machine learning, supervised machine learning, NLP, sentiment analysis, data mining, and others. The publications from each category are shown in Table 1.
It is important to highlight that this classification is only for this study, and it is not how machine learning and AI techniques are usually classified. The methods used in each study were identified and classified accordingly. Some publications did not specify any method or used different methods, or the method did not fall under the previously classified methods. Therefore, those publications were classified as others.
The category with the most publications is supervised learning, with 11. This category includes publications that used fuzzy, Naïve Bayes, support vector machine (SVM), and other supervised machine learning techniques. Data mining and NLP are the second categories with most publications, each with four articles. Unsupervised learning and sentiment analysis follow them (Table 1).
Table 1:The literature divided into categories.
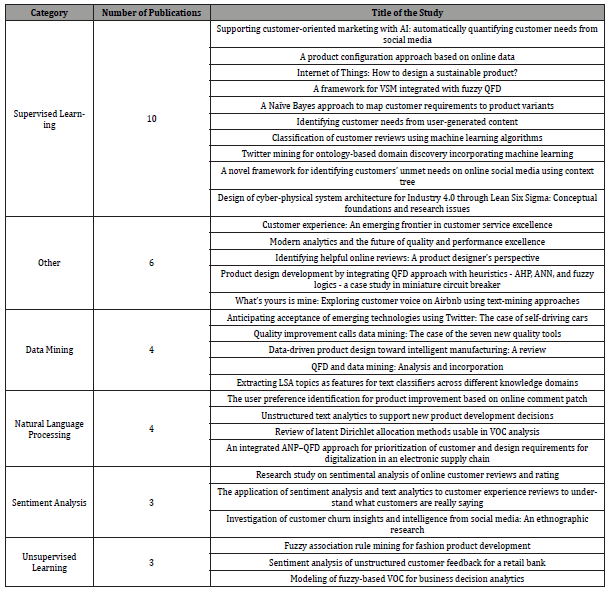
This section discusses each category to answer the research questions proposed in section 3 of this study. RQ1 and RQ2 are mainly addressed in this section, leaving RQ3 to be answered in a later section. It is essential to highlight that the publications considered in this study were selected based on the search strategy and eligibility criteria established by the PRISMA protocol. Each study was read and analyzed, which are discussed in this section.
Supervised Learning:
Supervised learning is a technique in machine learning that uses a training set to train the machine to classify new inputs based on the training set’s inputs and outputs. Supervised learning has been used in different areas, from information retrieval, data mining, speech recognition, and market analysis [8]. One of the areas in which supervised learning has been used is to identify important aspects of the VOC and improve customer satisfaction. Quality 4.0 is where smart techniques are used in quality tools and to improve quality concepts. This section analyzes publications where supervised learning techniques are used in the context of the VOC.
A critical aspect of the VOC is that the customer does not always have a clear idea of their needs. Questionnaires and forms are good, but they can fail to capture all customers’ desired aspects of their products or services. Also, those methods can be time-consuming and expensive if done correctly. Another point is that many customers do not want to be interviewed for new products, and the results generated by those methods can be misleading. In this new era of Industry 4.0 and the advent of technology, businesses must understand their customers’ needs better than the customers themselves to succeed in the competitive market [9]. In this new era, people share considerable amounts of valuable information on social media platforms that can be used to provide better products or services to customers.
The product design phase is where the VOC is the most critical input. Spending time, money, and effort on an organization to produce a failed service or product can harm the business. Jiao and Yang [5] proposed using online transaction data and customer reviews to map customers’ requirements to produce product configurations that exceed their expectations. Their method is based on supervised learning, where they initially scrub the transaction data to create all possible configurations. Then, they created a training dataset considering the positive customer review data. The algorithm is trained to learn the patterns in the data and find the best product configurations [5]. Jiao and Yang [5] used sentiment analysis to identify the positive reviews. However, the primary method used in the framework is the supervised learning algorithm.
Jiao and Yang [5] demonstrate the use of their proposed method in the design of a case of smartphones. They developed a framework that can be applied to different cases and areas by using online data to enhance customer satisfaction. Their approach has also been used in different publications, and it is a common approach when considering recommendation systems. Their novel configuration approach helps designers understand the VOC and use only important characteristics defined by the customer for the products, saving time, cost, and resources. When using this approach, companies become more competitive in a process known for being costly and time-consuming. Its efficacy was illustrated by the case study, where the use of the framework generates a more efficient and competitive configuration approach, reducing time, cost, and human resources and improving customer satisfaction. Jiao and Yang [5] pointed out some limitations to using their framework. For example, when extensive data is available, and the product has a large number of possible attributes, the training set can become large, making the algorithm slower and inefficient.
Another study that uses supervised learning to map the VOC to create product variants is the work of Wang and Tseng [10]. They used a Naïve Bayes approach to classify existing customer choices. The study also uses the proposed framework in a case study to illustrate its effectiveness. Since customers are not always clear in their requirements, companies must identify their needs based on available information. The case study exemplifies how to facilitate the designers’ job when designing new products and variants of the products. The Naïve Bayes algorithm learns from previous customers’ choice data and translates them into product variants. This framework is more applicable for a smaller number of variables. Otherwise, the problem can become too large to handle.
The research study from Kühl et al. [9] proposed using Twitter data to identify and prioritize customers’ needs. When using supervised learning, a training set is needed. Kühl et al. [9] highlighted that this part can be time-consuming, but once done, the algorithm can learn from the new data, producing sustainable results. They also proposed using semi-supervised and unsupervised learning for future work. Their case study contains a small sample of labeled tweets to exemplify their proposed method. While not ideal, it is a good way of gathering the VOC by means different from traditional questionnaires. The work of Kühl et al. [9] provides an essential difference between other works where they identified the need and its relevance. Their study identified multiple points related to the need for electric cars. Also, based on their study, they want to quantify which of those needs is more important to most customers. In that way, companies can target specific needs that will improve overall customer satisfaction.
The work of Alptekin [11] shows the use of an SVM applied to big data for a product design process in the Internet of Things (IoT) era. The study proposes an approach to the use of big data in product design in an automated way. They used their proposed method in a case study in the printing services industry where users do not change their setup and, most of the time, do not pay any attention to how the machine is set up before use. Their method provided cost reductions, and they could use supervised learning to correctly identify 92.23% of the media types used in their case study. This study shows a case where the methodology can proactively identify the customers’ needs and design the use of the service more efficiently.
Another supervised learning methodology was used by Mohanraj et al. [12]. Fuzzy Quality Function Deployment (QFD) was used when they proposed a framework for Value Stream Mapping (VSM). They conducted a pilot study where they could test and confirm their framework’s efficacy. QFD is a common technique used in companies that want to adopt Lean Six Sigma concepts. This study used the information gathered by the QFD process to improve the processes mapped using VSM.
In that study, they mapped the current state of the process and used fuzzy logic to map the critical weights of the House of Quality generated by the QFD processes. When using this technique to evaluate the VOC, they were able to identify points of improvement in the current process to focus on what is important to the customer. The prioritization generated by fuzzy logic in the QFD process helped to identify and improve the process to better meet the needs of the customer [12].
The research by Timoshenko and Hauser [13] is an important study about identifying the VOC by using machine learning techniques. This study proposed a hybrid machine learning framework using supervised learning and natural language processing to identify customer needs. The proposed method is important because it provides a better way of analyzing the VOC more efficiently based on feedback gathered from different sources. The method of User-Generated Content (UGC) reduces the time allocated to analyze the customers’ needs by 40%. They also identify possible additional applications for the framework and propose using UGC online to update the requirements as instantly as desired.
The use of supervised machine learning techniques can be advantageous for different industries. Many publications have been published about using those techniques to gather information from customer reviews. The use of those results can lead to better-designed products and satisfied customers. The research conducted by Noori [4] uses different supervised machine learning algorithms to extract content from online reviews to better design products. Review categorization is used, assigning documents to a pre-defined set of categories.
The study by Noori [4] uses SVM, artificial neural networks (ANN), Naïve Bayes, decision trees, and k-Nearest Neighbor (kNN) as classification algorithms. The study also used NLP techniques but mainly focused on the performance evaluation of the classifiers. They used three datasets with different features, proving that different algorithms are better for the different number of features. A decision tree is the best alternative when the number of features to be considered is large, which, in their case, is 1800 features. Naïve Bayes worked best on the small number of features (25 features considered). In the middle, SVM performed best for 100 features.
The study by Noori [4] highlights the importance of listening to the VOC in the hospitality industry, where most customers give feedback online as reviews on different websites. They also stress the importance of their work compared to the other studies in the area, where they mostly capture only the reviews. In their case, they compared different machine learning algorithms and their performance, considering the different features. Those results can and must be used to improve the customers’ experience by attentively listening to their feedback.
Another study that uses different machine learning classifiers on Twitter data is the work of Abu-Salih et al. [14]. The work highlights the importance of research to understand the VOC in order for businesses to maintain their competitiveness. They used tweets, classified some of them to create the training sets, and later tested the quality of the predictions generated by the algorithms used. The study used methods like Logistic classifier, SVM, decision tree, random forest, and gradient boosting as supervised machine learning. As with any other text data, the research also used NLP. However, it was not the main topic of the study. Companies can use the results of this research to gather and analyze customers’ requirements and identify the most important topics and off-topic features related to the products or services they are designing.
The last study analyzed in the category of supervised learning is the work of Ko et al. [15], where they propose a framework using a context tree. Their work differs due to the focus on extracting information about the unmet needs of customers using social media information. The research applied the proposed framework to developing new home appliance products. A context tree consists of a tree structure searching for related keywords hierarchically. They also used NLP techniques to analyze the text, but it was not the study’s focus, which is why it was classified into the supervised learning category. The work shows different scenarios and can demonstrate how efficiently using context trees identifies customers’ unmet needs. The same proposed method can be used to identify what a customer needs and does not specify clearly.
Supervised learning is a powerful tool that can extract and analyze the VOC in different ways and ultimately enhance the customers’ experiences. Many different techniques are being proposed and used. However, none of the studies mentioned Quality 4.0 and how the use of those techniques relates to the Industry 4.0.
Unsupervised Learning:
Unsupervised learning can be translated when no training data is needed. Clusters are formed based on patterns without any past guidance. For the present study, three publications were classified in this category. Two of them used unsupervised fuzzy methods. It is essential to highlight that fuzzy methods can be used as supervised or unsupervised because they can allow an item to pertain to different categories.
Aguwa et al. [16] proposes a methodology to transform text data from customer reviews to a quantitative format to generate a mapped Integrate Customer Satisfaction Index (ICSI). The study uses association rules to discover relationships among items in the comments and gather them together. The study also uses a classifier to indicate the level of positivity or negativity in the comments. Also, the comments are classified as insignificant, moderate, or significant to show their impact on the overall data. Then, they used fuzzy logic to convert the data processed into a numerical value to express the customers’ opinion about each critical to quality (CTQ) point defined previously. The case study demonstrated by Aguwa et al. [16] used data from the auto industry. They applied their methodology to the dataset and were able to identify the ICSI to six mapped CTQ points. The quantified amount for each CTQ point can help in different decision-making processes related to warranties, customer satisfaction, product development, and product design.
In another study, Lee et al. [17] employed the fuzzy association rule for product development in the fashion market. The study proves the efficiency of its method by applying it to data from a fashion company. The proposed method consists of four phases: identifying parameters, defining fuzzy characteristics of the parameters, generating fuzzy association rules, and evaluating rules. The case study demonstrated that the method could generate important information about the new products. Using that technique can improve customer satisfaction and increase the efficiency of new product development processes.
The last study in this category is the work by Bhattacharyya and Dash [18]. Their research uses online user-generated content in an ethnography approach to understand users’ behavior in the telecommunication industry. The study is complete, and it can identify patterns in the data that provide a good indication of the customers’ behavior based on the data. For the specific industry, they were able to identify attributes of churn and to identify what are the main change before actual churn occurs. The study also proposes solutions for the problems identified.
Data Mining:
Data mining is part of knowledge discovery in data (KDD). Its main goal is to identify patterns in data that will provide valuable insight into the data. Data mining transforms data without meaning into knowledge [3]. Data mining is usually combined with machine learning techniques to obtain the best analytical results. One of the uses of data mining is to obtain the VOC through raw datasets. The publications in this section used data mining to obtain valuable information about customers’ requirements and needs.
Zhang [19] uses text mining, a technique of data mining using text, to explore Airbnb reviews to identify valuable information about the VOC. This study sought to identify what is being promoted correctly and what is not working from the customers’ perspective through the use of different techniques, such as context analysis and topic modeling. The study used the linguistic inquiry and word count (LIWC) program to analyze the content of Airbnb reviews. The study also compares the reviews given to Airbnb and hotels. The purpose was to identify patterns related to the positive and negative comments data. The result demonstrated that most of the reviews were positive. However, negative reviews were more authentic. The study gathered the VOC from online reviews and translated them to topics in the hospitality industry. Companies can use those topics to improve their services.
Another study that used data mining to gather and analyze the VOC is the research by Kohl et al. [20]. They analyzed the acceptance of a new product using Twitter data. This study shows a way of using machine learning techniques in the early stages of product development. In the earliest design stages, changes are cheaper and have a small impact on the overall product. As time passes and the design becomes more robust, any changes will interfere with the product and the processes. The costs and impact of the change will be higher [21]. Also, whenever the VOC is heard in the early stages of design, the company can decide if the project is worth it. The study by Kohl et al. [20] used text mining algorithms to classify the tweets they collected for 19 months. The research developed two metrics to evaluate new products’ risk rate (RR) and the benefit rate (BR). The study also uses sentiment analysis and SVM, classified as data mining due to its main application, which is to use the VOC to evaluate the acceptance of self-driving cars. They demonstrated that their results are consistent and provide the same trend as the other methods to analyze the VOC, such as surveys and questionnaires.
Data mining is also explored in the work of Feng et al. [22]. The study aims to summarize the existing research for data-driven product design processes. The study highlights that data mining methods come with the pre-requisite of having data available for analysis. They differ between online data (current) and historical data and the implications of using them to design new products. The study points out that studies in this area are scarce. This study can also confirm that even though much has been done in this area in the near past, much more is available for exploration. This study focuses more on the manufacturing side of the application of data to understand the VOC.
Data mining can also be used to analyze the VOC through complaints. The study by Tsironis [23] uses data from vehicle damages to implement data mining techniques for quality improvement. In this study, the customer was considered internal since the complaints collected were from vehicles used by the company. The study proposes a more automated process to generate seven new quality tools to solve problems related to the VOC. Using data mining techniques on the raw data enabled the identification of hidden patterns and the automatic generation of relationships, matrices, arrows, and other diagrams.
The last study considered in the data mining category is the work of Sharma et al. [24], the oldest literature used in the present study. The research by Sharma et al. [24] proposed using data mining in the QFD process. The research highlights that human analysts cannot easily identify the hidden pattern in the data as a trained computer. The QFD process can generate enormous amounts of data that can be hard to analyze. Using data mining techniques in that process helps reduce the time, improve the quality of the analysis, and consequently reduce the costs incurred by that process. The use of data mining in the VOC data can show the patterns, avoiding the tedious work of looking at and filling in the matrices of the QFD process. The QFD process can feed itself from the data collected and mined by the algorithms. The research by Sharma et al. [24] does not provide a case study where data mining techniques are used in the QFD process, but the technique was implemented in some studies such as the one by Ni et al. [25], which was not analyzed in this study.
Natural Language Processing
NLP is a field of AI that uses computer algorithms to analyze human language [26]. Many different algorithms and methods can be used to analyze text data. The articles in this category present those techniques as a main subject. NLP can be used in different categories when text is present. For example, sentiment analysis or text mining can only be done by using NLP algorithms prior to or with their algorithms. The main problem when analyzing text data is the fact that the data is usually in the form of unstructured text. Text is considered unstructured data, while numbers are structured data [27]. Comments on social media, reviews of products, or answers to questionnaires can have different formats and contain different types of structures and symbols. Also, people can be ironic or sincere using the same set of words. Those peculiarities usually make it hard to automate the analysis of text data. NLP techniques are used to make sense of text data.
The research presented by Li et al. [28] brings an application of NLP algorithms to analyze the VOC to identify users’ preferences based on online comments. They experimented with the users’ preferences for laptop products. The study uses different methods, such as sentiment analysis, preference mining, the Kano model, word2vec, and convolutional neural network (CNN). The experiment results show how valuable the data from comments can be to support design decisions considering the VOC and their preferences.
Evangelopoulos and Amirkiaee [29] use latent semantic analysis (LSA) to show how feature extraction can work in text data. Feature extraction is essential for the use of classifiers. The study from Evangelopoulos and Amirkiaee [29] uses an exploratory
case study to answer their research questions. The use of LSA in a unified corpus and a separate corpus was analyzed. The results show that the unified corpus provides better classification features to identify the topics than the separated corpus. The results show that the unified corpus provides better classification features to identify the topics than the separated corpus. The study used 4914 articles published in specified academic journals over a 25-year period as data. Even though the data set was large, using topic extraction features in articles is easier than in comments from social media, for example. That is because articles from different areas use different specific words.
The use of unstructured text analysis to understand the VOC and its use in the development of new products is also addressed in the study by Markham et al. [27]. The study applies NLP techniques to two cases to show how unstructured data can be used to gather the VOC subjectively. The first case uses the data to support the design of a new product to be launched in a new market. The second case uses textual data to identify new customers and market opportunities by understanding the customers’ needs. In both cases, the well-analyzed data provided the companies with information about their customers and supported their decisionmaking.
One important point made by Markham et al. [27] was that the data cannot provide valuable information if the right questions are not asked. It is important to highlight this because the analysis and the selection of the correct methodology are critical to successfully implementing automated analysis. If the organization can collect valuable data and build the capability to analyze, it will create a competitive advantage by using that information to exceed its customers’ expectations.
The work of Sperkova [30] focuses on reviewing topic modeling techniques of Latent Dirichlet Allocation (LDA) used to analyze the VOC data. LDA is an NLP model to find topics in an unsupervised way. The study identified 38 articles that modified the LDA model applied to textual data related to the VOC of any kind. The resulting literature review contains information about the principles and performance of the models proposed in the 38 studies reviewed.
Sentiment Analysis:
Sentiment analysis is a part of machine learning techniques used to analyze a text to obtain the sentiment related to it. Usually, it is classified as positive, negative, and neutral [31]. Different studies have been published on sentiment analysis, especially using social media data related to politics, news, products, or other topics. The use of sentiment analysis can be valuable in analyzing the VOC data. It is an important topic because it can automatically distinguish points of improvement from negative sentiment comments and strengths from positive sentiment comments.
Kazmaier and van Vuuren [31] use sentiment analysis to gather information about a retail bank in South Africa. They analyze reviews of products and services from the specific bank. The data is pre-processed, the first step for any textual data analysis, so sentiment analysis techniques can be used. Some reviews were randomly selected and classified as positive, negative, and neutral. This step is also critical to the algorithm’s success since its results depend on how accurate the training set is. As in reviews or comments, the data presented challenges like misspelling and punctuation, which a good pre-processing step can correct. The study results show a promising use of customer reviews to gather their sentiments about products and services.
Another study that uses sentiment analysis to analyze the VOC is by Kaur and Gupta’s [32]. The research demonstrates the creation of a system to analyze the opinions of customers based on their online reviews and ratings. The study does not specify the database used for the results demonstrated in the study. However, it demonstrated using sentiment analysis to classify different products according to customers’ preferences.
Gallagher et al. [33] present a practical application to compare the products’ ratings to verbatim feedback given by customers. The study aimed to analyze whether customers have the same opinion when rating a product on a scale of 1 to 5 than when asked or allowed to give free feedback. The study found a discrepancy between the product ratings and the sentiment of the customer reviews on their phone case study. This discrepancy is important because even though customers rated the product with the lowest score, the sentiment towards that product was not negative. Therefore, understanding the VOC is important for companies wanting to stay in business.
Other:
This category has different machine learning approaches to analyze the VOC. The publications in this category do not have a primary method, use a method not categorized previously, or do not present a method but an overview of other methods not listed.
The work of Evans [34] discusses modern analytics and how they impact the future of quality performance excellence. It conveys the role big data, data analytics, and machine learning techniques play in pursuing quality to satisfy customers. It relates to the work of Batra [35], which discusses the customer experience as the main factor in the perception of customer satisfaction in the twentieth century. Both publications provide a theoretical background that includes automated analysis of the VOC.
The study by Sony [36] focused on the design of cyber-physical system (CPS) architecture in an Industry 4.0 environment. The study promotes using AI algorithms to learn based on the train to acquire information from the cyber-level system. Using the AI cognition system guarantees that the features that have value perceived by the customer are considered. Sony [36] also mentions the use of deep learning in this stage as well.
Two publications in this category discuss QFD. The first one is the work of TS and V [37], designing an integrated analytic network process (ANP) to be used with QFD. The second study by Kant [38] provides a case study where QFD is used with fuzzy logic. In the first study, they identify and prioritize the design and customer requirements using the methodology proposed. They tested their method in a supply chain setting for an electronic company. The work specifies the importance of the supply chain, especially in the design of new products through digitalization. The work of prioritizing requirements can be time-consuming and misleading, which can be avoided by automating the use of the data from QFD through the method proposed. The study provides the theoretical basis and the calculations necessary for the best understanding of the case study.
Conclusions, Limitations, and Future Work
This study offers a review of publications that used AI to analyze the VOC. The set of keywords was defined to gather the best results related to that topic. This study followed the PRISMA protocol to discuss three research questions related to the subject of the literature review. Those questions were defined to discuss the current automated techniques and how they are being used to analyze the VOC.
The answers to the first and second research questions are addressed in section 5. AI techniques are being used to analyze the large amount of data generated by the VOC in the Industry 4.0 era. However, the studies focus on using the techniques and generating results rather than improving the product development process. The main AI techniques used in the field are supervised and unsupervised machine learning, data mining, NLP, and sentiment analysis.
As with any research, there are limitations to this study. The main limitation of this study is that only peer-reviewed journal publications that were published in English were included. Grey literature was excluded as well as publications in other languages. Therefore, additional research may exist that was not included.
This study was able to identify that the central gap in the area is to relate the use of those techniques to Quality 4.0 and improvements in Industry 4.0 since most of the publications were focused on the use of the technique but not on the impact of those techniques for the field in the fourth industrial revolution. Those gaps can be filled by future work in the area where AI techniques can be used to analyze the VOC and implement online improvements into products and processes [39-43].
Acknowledgment
None.
Conflict of Interest
No conflict of interest.
References
- Quality 4–0 | ASQ. (n.d.). ASQ.
- Delua J (2021) Supervised vs. unsupervised learning: What’s the difference? IBM.
- Education IC (2021a) Data Mining. IBM.
- Noori B (2021) Classification of customer reviews using machine learning algorithms. Applied AI 35(8): 567–588.
- Jiao Y, Yang Y (2019) A product configuration approach based on online data. Journal of Intelligent Manufacturing 30(6): 2473–2487.
- Moher D (2009) Preferred reporting items for systematic reviews and meta-analyses: The PRISMA statement. Annals of Internal Medicine 151(4): 264.
- Farahani FV, Karwowski W, Lighthall NR (2019) Application of graph theory for identifying connectivity patterns in human brain networks: A systematic review. Frontiers in Neuroscience, 13.
- Liu Q, Wu Y (2012) Supervised Learning. Encyclopedia of the Sciences of Learning, pp. 3243–3245.
- Kühl N, Mühlthaler M, Goutier M (2019) Supporting customer-oriented marketing with AI: Automatically quantifying customer needs from social media. Electronic Markets 30(2): 351–367.
- Wang Y, Tseng MM (2013) A Naïve Bayes approach to map customer requirements to product variants. Journal of Intelligent Manufacturing 26(3): 501–509.
- Alptekin SE (2017) Internet of Things: How to design a sustainable product? Journal of Management & Engineering Integration 10(2): 28–35.
- Mohanraj R, Sakthivel M, Vinodh S, Vimal K (2015) A framework for VSM integrated with Fuzzy QFD. The TQM Journal 27(5): 616–632.
- Timoshenko A, Hauser JR (2019) Identifying customer needs from user-generated content. Marketing Science 38(1): 1–20.
- Abu Salih B, Wongthongtham P, Yan Kit C (2018) Twitter mining for ontology-based domain discovery incorporating machine learning. Journal of Knowledge Management 22(5): 949–981.
- Ko T, Rhiu I, Yun MH, Cho S (2020) A novel framework for identifying customers’ unmet needs on online social media using context tree. Applied Sciences 10(23): 8473.
- Aguwa C, Olya MH, Monplaisir L (2017) Modeling of fuzzy-based voice of customer for business decision analytics. Knowledge-Based Systems 125: 136–145.
- Lee CKH, Tse Y, Ho G, Choy K (2015) Fuzzy association rule mining for fashion product development. Industrial Management & Data Systems 115(2): 383–399.
- Bhattacharyya J, Dash MK (2020) Investigation of customer churn insights and intelligence from social media: a netnographic research. Online Information Review 45(1): 174–206.
- Zhang J (2019) What’s yours is mine: Exploring customer voice on Airbnb using text-mining approaches. Journal of Consumer Marketing 36(5): 655–665.
- Kohl C, Knigge M, Baader G, Böhm M, Krcmar H (2018) Anticipating acceptance of emerging technologies using Twitter: The case of self-driving cars. Journal of Business Economics 88(5): 617–642.
- Blanchard BS (2021) Systems Engineering and Analysis 5th (fifth) edition text Only. Prentice Hall.
- Feng Y, Zhao Y, Zheng H, Li Z, Tan J (2020) Data-driven product design toward intelligent manufacturing: A review. International Journal of Advanced Robotic Systems 17(2): 172988142091125.
- Tsironis LK (2018) Quality improvement calls data mining: the case of the seven new quality tools. Benchmarking: An International Journal 25(1): 47–75.
- Sharma AK, Sharma JR, Sharma SA, Agrawal PS (2011) QFD and data mining: Analysis and incorporation. National Journal of System and Information Technology 4(2): 162.
- Ni M, Xu X, Deng S (2007) Extended QFD and data-mining-based methods for supplier selection in mass customization. International Journal of Computer Integrated Manufacturing 20(2–3): 280–291.
- Education IC (2021b) Natural Language Processing (NLP). IBM.
- Markham SK, Kowolenko M, Michaelis TL (2015) Unstructured text analytics to support new product development decisions. Research Technology Management 26(3): 30–38.
- Li S, Zhang Y, Li Y, Yu Z (2019) The user preference identification for product improvement based on online comment patch. Electronic Commerce Research. Published.
- Evangelopoulos N, Amirkiaee SY (2019) Extracting LSA topics as features for text classifiers across different knowledge domains. Quality & Quantity 54(1): 249–261.
- Sperkova L (2018) Review of Latent Dirichlet Allocation methods usable in voice of customer analysis. Acta Informatica Pragensia 7(2): 152–165.
- Kazmaier J, van Vuuren J (2020) Sentiment analysis of unstructured customer feedback for a retail bank. ORiON 36(1).
- Kaur R, Gupta G (2017) Research study on sentiment analysis of online customer reviews and ratings. International Journal of Advanced Research in Computer Science 8(7): 393–397.
- Gallagher C, Furey E, Curran K (2019) The application of sentiment analysis and text analytics to customer experience reviews to understand what customers are really saying. International Journal of Data Warehousing and Mining 15(4): 21–47.
- Evans JR (2015) Modern analytics and the future of quality and performance excellence. Quality Management Journal 22(4): 6–17.
- Batra MM (2017) Customer experience: An emerging frontier in customer service excellence. Journal of Management & Engineering Integration 15(1): 198–207.
- Sony M (2020) Design of cyber-physical system architecture for industry 4.0 through Lean Six Sigma: conceptual foundations and research issues. Production & Manufacturing Research 8(1): 158–181.
- TS D, VR (2020) An integrated ANP–QFD approach for prioritization of customer and design requirements for digitalization in an electronic supply chain. Benchmarking: An International Journal 28(4): 1213–1246.
- Hundal GPS, Kant S (2017) Product design development by integrating QFD approach with heuristics - AHP, ANN, and fuzzy logics - a case study in miniature circuit breaker. International Journal of Productivity and Quality Management 20(1): 1.
- Davahli MR, Karwowski W, Taiar R (2020) A system dynamics simulation applied to healthcare: A systematic review. International Journal of Environmental Research and Public Health 17(16): 5741.
- Library (n.d.) Literature searching explained. University of Leeds.
- Liu Y, Jin J, Ji P, Harding JA, Fung RY (2013) Identifying helpful online reviews: A product designer’s perspective. Computer-Aided Design 45(2): 180–194.
- Radziwill NM (2020) Connected, Intelligent, Automated: The Definitive Guide to Digital Transformation and Quality 4.0 (1st ed.). Quality Press.
- Wang C, Li Y (2021) Digital twin aided product design framework for IoT platforms. IEEE Internet of Things Journal, p. 1.
-
Cintia Zuccon Buffon, Elizabeth A Cudney, Ahmad Elshennawy* and Sandra Furterer. Artificial Intelligence Techniques Used for Analyzing the Voice of the Customer in the Quality 4.0 Era: A Systematic Review Using PRISMA Protocol. Iris J of Edu & Res. 2(3): 2024. IJER. MS.ID.000539.
-
Voice of the customer (VOC), PRISMA Protocol, Artificial Intelligence Techniques, Artificial intelligence (AI)
-
This work is licensed under a Creative Commons Attribution-NonCommercial 4.0 International License.