Research Article
Behavioral Measures of Gain-Loss Asymmetry as a Function of Gender and of Virtual v. Actual Money
Diego G Flores*, Harold Miller, Jr., Bruce Brown, Chongming Yang, Michael Seeley, Darin Costello, Frank Robertson, Marcia Ventura and Blake Hansen
Department of Psychology, Brigham Young University, USA
Diego G Flores, Department of Psychology, Brigham Young University, USA.
Received Date: March 31, 2023; Published Date: May 04, 2023
Abstract
Cognitive psychologists have used cognitive methods involving hypothetical scenarios and outcomes to identify and measure the asymmetrical effects of gains and losses on human choice and decision-making. In our experiments, we used behavioral methods and measures instead. The participants (N=26 with 15 females) played a computer game during daily 36-min sessions. Embedded in the game were conjoint interdependent concurrent variable-interval schedules for both gains and losses. Occasionally, clicking produced an on-screen indication of a gain (“+10¢”) or a loss (“-10¢”). The research design was three-part. We first determined participants’ loss/gain asymmetry ratios. The overall mean ratio using a mixedmodel analysis was 2.18. Second, we parsed the results by gender. The mean ratios were 2.49 for females and 1.92 for males. Third, we compared the effects of (a) converting +/- points that appeared on the screen during the game to money at the game’s end versus (b) displaying points on the screen and simultaneously delivering money through a coin-dispenser or requiring the participant to deposit coins instead. All participants showed increased loss aversion in the latter condition. This result was unaffected by gender. We discussed our findings in the context of prospect theory, a leading cognitive account of risk aversion.
Keywords:Humans; Video game; Concurrent variable-interval schedule; Gain (reinforcer); Loss (punisher); Gain-loss asymmetry gender difference; Prospect theory; Gain/loss asymmetry; Loss aversion; Risk aversion
Behavioral Measures of Gain-Loss Asymmetry
How humans make decisions has been a recurring topic in the history of psychology. Two views currently dominate the undertaking, one based on the perspective that decision-making occurs rationally and the other on the view that decision-makers are emotionally biased and thus irrational. The utilitarian approach of classical economists was the rational-agent model (Homo economicus), a dispassionate and unbiased maximizer of personal gain. However, the Nobel laureate Daniel Kahneman and Amos Tversky, his long-time partner in research and theory, among other founders of behavioral economics, developed a deliberately cognitive model emphasizing human irrationality after identifying several violations of utility theory [1]. They pointed out that most people prefer a sure gain over a risky prospect—a preference known as risk aversion. Additionally, some individuals prefer a risky gamble over a specific loss, a preference known as riskseeking. According to LeBoeuf and Shafir [2], “With the exception of prospects that involve very small probabilities, risk aversion is generally observed in choices involving gains, whereas risk seeking tends to hold in choices involving losses” (p. 245). The hedonic asymmetry of gains and losses is also exhibited in loss aversion. Individuals may make decisions based on the potential value of losses and gains separately rather than on an aggregated outcome, and they evaluate losses and gains using heuristics. Kahneman, Knetsch, and Thaler [3] reported that the effect of losses was two times more punishing than the effect of gains was rewarding. The research on which this claim rested typically involved participants’ exposure to a series of hypothetical scenarios that offered choices in the form of probabilistic gains or losses that were also hypothetical. Rasmussen and Newland [4] used a behavior-analytic method, found a substantial bias towards the unpunished alternative, and concluded that “. . . when the monetary gain is the same on the other options, it appears that losing a penny is three times more punishing than earning the same penny is reinforcing (p. 65). That is, the hedonic impact of punishers was half-again greater than Kahneman et al. had found.
The Matching Law
We interpreted the differential effects of gains and losses on behavioral choice in terms of the matching law (see Herrnstein, 2000). The basic principle of reinforcement was formulated by Thorndike (1911) [6] as the law of effect, which stated that actions followed by feelings of satisfaction were more likely to be repeated, but actions followed by feelings of annoyance are not. The matching law was first formulated by R. J. Herrnstein (1961) following an experiment with food-deprived pigeons exposed to concurrent variable-interval (conc VIVI) schedules of reinforcement. Herrnstein’s matching law and the generalized matching law (Baum, 1974) [7] both refer to solely to behavioral events, such as responses or time spent responding, and to environmental events, such as reinforcers, to measure choice between alternatives. That is, they do not rely on cognitive processes or events, which are, by definition, only indirectly observable and inferred. Both laws have been applied effectively in cases where reinforcers were used to influence an individual’s behavior.
However, efforts to introduce punishers into the original formulations of have created complications. Deluty (1976) and de Villiers (1977, 1980) [8,9,10] developed different quantitative models of punishment that were versions of the avoidance theory of punishment and of the negative law of effect, respectively (Mazur, 2006) [11].
In the de Villiers model, punishers are subtracted from reinforcers. Rasmussen and Newland (2008) [4] used this model to describe the outcome of experiment. In one type of condition only reinforcers were delivered at both response alternatives. In a different type of condition, both reinforcers and punishers were delivered at one response alternative, but only reinforcers were delivered at the other. As previously noted, the authors observed that adding punishers tended to reduce the value of reinforcers as measured by the proportion of behavior allocated to the punished alternative.
We used a procedure like that of Rasmussen and Newland (2008) [4] and applied both the subtractive model as well as an indirect model of punishment to our results. The latter does not directly include punishers but, instead, represents the effect of punishers by variations in the effects of reinforcers. In other words, using this model, it is not necessary to include both reinforcers and punishers to measure their asymmetrical effects. Also, the use of this model eliminates the problem of negative values when the subtractive model is used.
The matching law specifies an equality between the ratios of the frequencies of two behaviors (B) and the reinforcers (R) they produce:
BL/BR = RL/RR, (1)
where the subscripts denote the locations, left or right, of the response alternatives in the procedure. The generalized matching law (Baum, 1974) is:
BL/BR = k(RL/RR)c , (2)
where k and c are parameters representing bias (due to reinforcer quality or to spatial preference, for example) and sensitivity (to changes in the distribution of reinforcers between two alternatives, for example), respectively (Miller, 1976). In logarithmic form, the equation is:
log (BL/BR) = log k + c log (RL/RR). (3)
We used a generalized-matching-law approach in the current research. In our version, B represented the total number of clicks of a computer mouse and R the total number of reinforcers that appeared on a computer monitor. The subscripts identified the left (L) and right (R) panels on the monitor screen. The parameter k represented bias, that is, a consistent preference for one response alternative over the other (Miller, 1976). The parameter c referred to the sensitivity of behavior to reinforcers or punishers, that is, the degree to which the ratio of responses to the two alternatives was affected by changes in the ratio of reinforcers or punishers that responding produced.
Method
Participants
The 26 participants (15 females) were students at Brigham Young University in Provo, UT who volunteered by means of an online recruitment system. Each signed an informed consent agreement prior to participating per the requirements of the BYU Institutional Review Board.
Materials and Procedure
The SubSearch Game. Figure 1 contains a screenshot from the SubSearch computer game that was created for the experiment. Participants played the game in a 9-ft by 9-ft room containing a wooden table and chair. The table contained a Dell® desktop computer equipped with a 17-in monitor and a mouse. The room had no windows and was artificially illuminated. The participant was seated in front of the monitor and asked to read the printed instructions for the game. The instructions appeared in English. Participants were instructed to guide a small submarine using the computer mouse to retrieve as many yellow objects shown on the screen as possible. After the participant clicked the “Start– OK” message that initially appeared on the screen, a 36-min session commenced. Each participant completed eight sessions. Experiment 1 included the first six sessions. During these sessions,participants received only virtual points (“+10¢” or “-10¢), which were displayed on the screen. However, in sessions 7 and 8, in addition to the virtual points, participants collected dimes when a reinforcer was delivered via a coin dispenser or deposited them when a punisher was displayed.
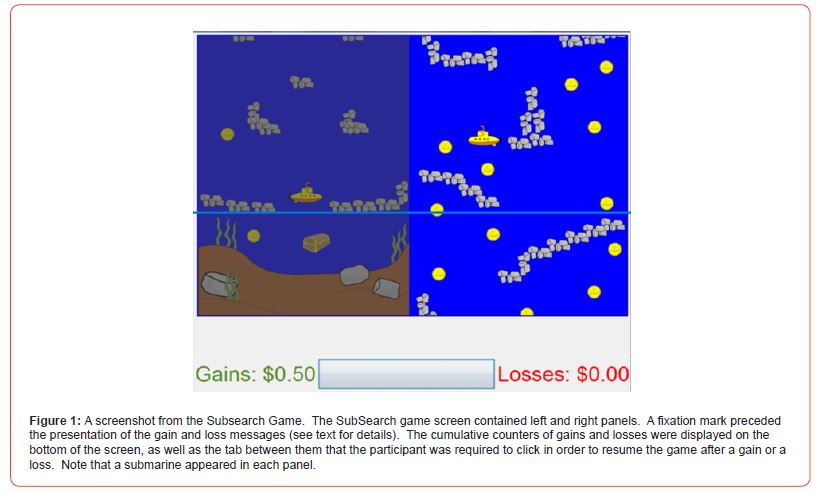
The game was played on two different vertical panels separated by a vertical line (see Figure 1). The participant could move the cursor from the left panel to the right panel and vice versa. However, each switch produced a changeover delay of 2 s. During this interval, no reinforcers or punishers were delivered. Moreover, movement from one panel to the other panel dimmed the former and paused the action there. In the active half of the screen, the display slowly scrolled upward until the sea bottom was reached. Then the display was reset to its original position, and the scrolling resumed. With each reset (approximately 10 times per game), the number of on-screen barriers increased. Reinforcers and punishers were signaled by separate on-screen messages that appeared in the center of the screen, each accompanied by a distinctive, brief sound. As previously noted, the messages were “+10¢” and “-10¢”. Cumulative counters of gains and losses appeared at the bottom of the screen and were separated by a tab. Whenever a message appeared on the screen, the participant was required to click on the tab to update the respective counter and to resume the game.
The game included conjoint interdependent conc VIVI schedules of reinforcement and punishment. Unlike independent conc VIVI schedules in which the two schedules are independent of each other, the interdependent version assigns a reinforcer (or a punisher) according to a preset probability generator. For each panel there were both a schedule of reinforcement and a schedule of punishment. The interdependency of the constituent conc VIVI schedules assured that the ratio of the frequency of received reinforcers or punishers closely approximated the programmed ratio.
Each session consisted of a fixed sequence of six 6-min conditions (1-6). Three of them (1, 3, and 5) contained conc VIVI schedules of reinforcement and three (2, 4, and 6) contained conjoint conc VIVI schedules of reinforcement and conc VIVI schedules of punishment. Table 1 summarizes the conditions. Each no-punisher condition was followed by a similar condition that included punishers only in the left panel delivered according to a schedule identical to that for the reinforcers that were delivered in that same panel. The schedule of punishment in the right panel was extinction (ext.), that is, no punishers were delivered. It should be noted that the values of the conc VIVI schedules were selected to produce the same overall rate of reinforcement (6/min) despite the difference in the ratios of those values (1:3, 1:1, and 3:1). Also, as previously noted, the ratio of reinforcers to punishers was 1:1 in the left panel. Daily sessions were conducted until there was no visually observable trend in the response ratio. At the end of each session, participants received the net amount of money they had accumulated during the session. Once they completed the experiment, each received a one-time bonus of $30 (Table 1).
Table 1:Reinforcer and Punisher Rates per Condition. Rates are Numbers per Minute.
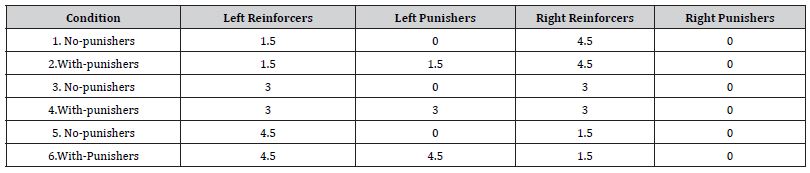
Data Analysis. All analyses were performed using IBM SPSS Statistics 23 and Microsoft Excel®. Measures included the number of responses (clicks) to the left and right alternatives (BL and BR) and the number of reinforcers (RL and RR). The response ratio BL / BR and the reinforcer ratio RL / RR were employed in the analysis. The generalized matching law (Baum, 1974) [7] was the basis for the participant-by-participant and the aggregate analyses. We adopted it without explicit formal consideration of punishers, an approach that we identified as the “indirect method”. The reinforcer ratios were calculated by dividing the net reinforcers received in the left panel (RL) by those received in the right panel (RR) and then log-transformed. Together with the logs of the response ratio, these data were fitted using linear regression. Then the antilogarithms of the intercept (log k) of the fitted equations for unpunished conditions 1, 3, and 5 and for the punished conditions 2, 4, and 6 were used to calculate the loss/gain ratio, which became the measure of asymmetry.
We used a Linear Mixed Model (LMM) for comparison of the results from the two types of conditions. In the mixed model, log (RL/RR) was the independent variable and log (BL/BR) was a covariate. Punishment and Gender (male or female) were factors included in the model. The hypotheses were:
Hypothesis 1: Participants were expected to be more sensitive to losses than to gains and to exhibit an asymmetry ratio between 2.0 and 3.0, consistent with the earlier findings of Kahneman and Tversky (1979) [1] and Rasmussen and Newland (2008) [4].
Hypothesis 2: The asymmetry ratio would be higher for women than for men (see Charness & Gneezy, 2012).Hypothesis 3: When rewards and punishers were virtual (monetary amounts displayed on a monitor) and actual (coin delivery or deposit) , loss aversion would be greater than when rewards and punishers were only virtual.
Results
Experiment 1
The Type III test of fixed effects of the LMM analysis results are displayed in (Table 2). Intercept, Punishment, and Gender were the main factors in the model. The Intercept F (1,460) = 147.448, p = .000 and the Punishment F (1,460) = 91.566, p = .000 were significant at the p ≤ 0.001 level, and the difference between male and female participants was significant at the p ≤ 0.05 level, Gender F (1,460) = 3.954, p = .047. No interactions were significant at the p ≤ 0.05 level (Table 2).
Table 2:Linear Mixed Model Analysis: Type III Tests of Fixed Effectsa.
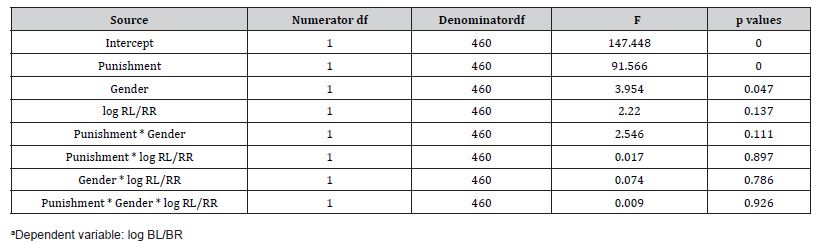
Table 3 shows the comparison between Punishment and No- Punishment conditions. The antilog of the mean in the conditions with no punishers was 0.411 and in the conditions with punishers was 0.897. Thus, the overall loss-gain ratio was 2.18. Table 4 exhibits the gender differences in the asymmetry of gains and losses. The antilog of the mean in the conditions with punishers for male participants was 0.912 and for female was 0.885. For the condition with no punishers, the antilogs were 0.476 for the male participants and 0.356 for the female participants. Thus, the overall loss/gain ratio for males was 1.91 and for females was 2.49.
Table A1 in the Appendix displays the means for responses, obtained reinforcers, obtained punishers, and changeovers for all 26 participants in the no-punisher conditions of the experiment. The corresponding results for the punisher conditions appear in Table A2. Table A3 exhibits the LMM results per participant as well as the corresponding gain/loss ratios (Table A1, Table A2, Table A3).
Table 3:LMM model: Asymmetry of Losses and Gains (Means and SEs); df = 460.

Table 4:LMM model: Asymmetry of Gains and Losses–Gender Differences (Means and SEs); df = 460.

Table 5:Results From No-Punishers Conditions Showing Mean Responses, Obtained Reinforcers, Obtained Punishers, and Changeovers Per Participant.
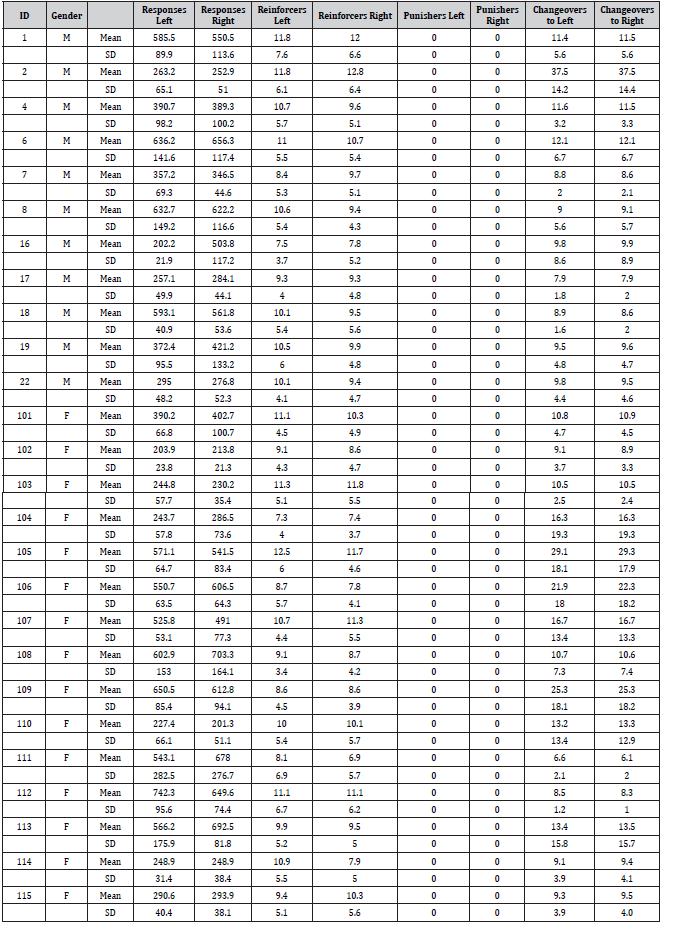
Table 6:Results From With-Punishers Coditions Sowing Mean Responses, Obtained Reinforcers, Obtained Punishers, and Changeovers for the Punished Alternative Per Participant.
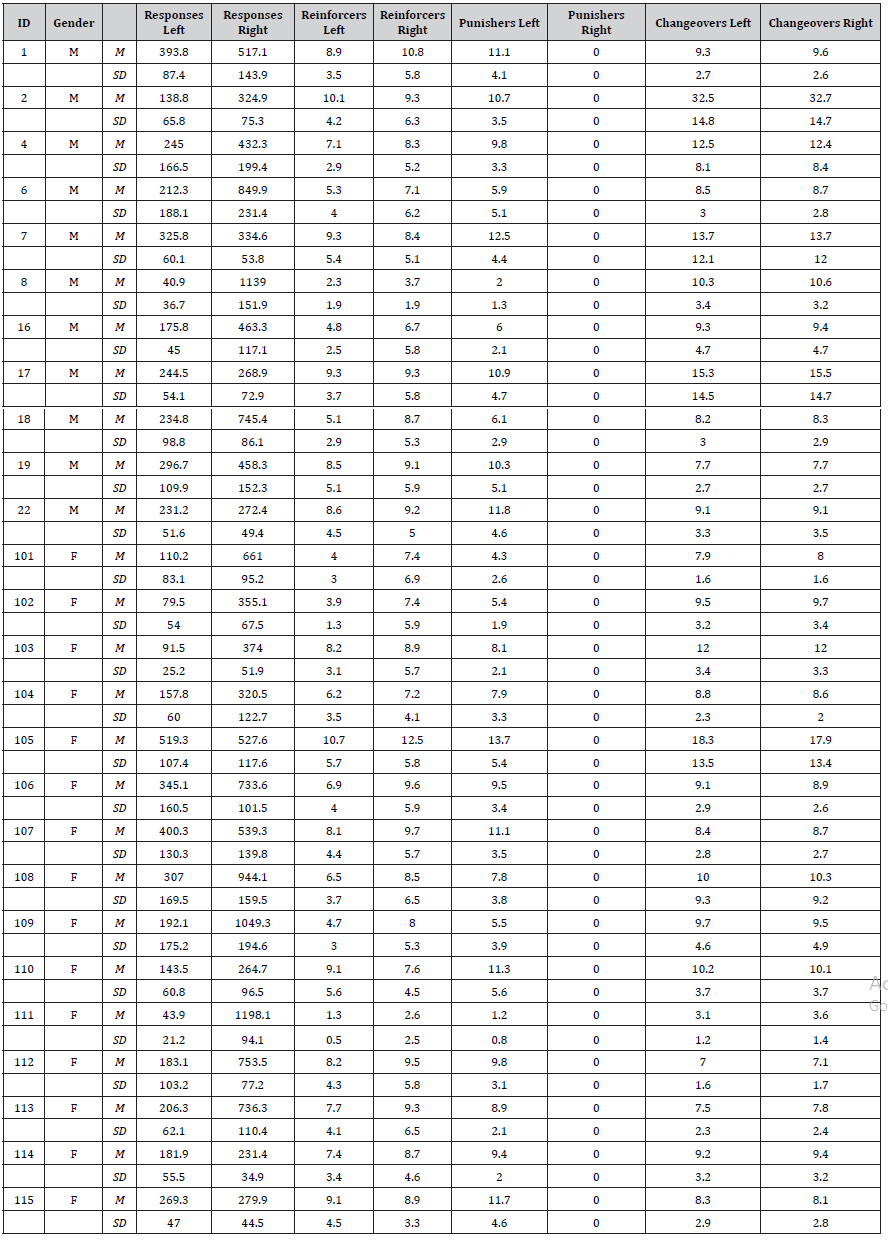
Table 7:Linear Mixed Model Results: Individual Loss-Gain Ratios - Df= 7.
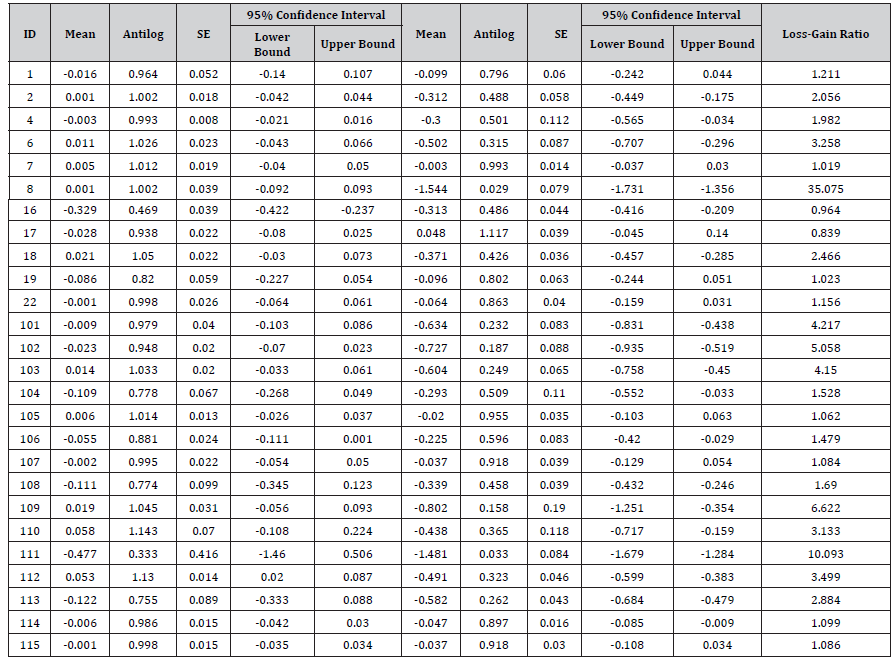
Experiment 2
The results of the comparison between Virtual Money Plus Cash Versus Virtual Money were: Punishers F (1.754), 223.917, p = 0.000; Dispense/Deposit Device F (1.754) = 7.156, p = 0.008; Punishers * Dispense/Deposit Device F (1.746), 12.968, p = 0.000. All other interactions were not significant at the p ≤ 0.01 level. The estimate of the covariate residual was 0.167586 and the SE = 0.008631. The estimated marginal grand mean was -0.293 and the SE = 0.015, df = 754, 95% CI [-0.293,-0.233].
Table 4 is a summary of the means, SEs, df, and 95% CI for the interaction of punishment and coin dispenser/collector. The gain/loss ratios were calculated by dividing the antilogarithms (M) of the No Punishment condition by the Punishment condition. In addition, the variable Gender was included in the LMM to determine whether differences resulting from virtual outcomes alone versus the combination of virtual-plus-cash outcomes were mediated by gender. The results were: Punishment F (1.746), 205.942, p = 0.000; Gender F (1.746) = 2.344, p = 0.126; Dispense/Deposit Device F (1.746) = 7.387, p = 0.007; Punishment * Dispense/Deposit Device F (1.746), 12.107, p = 0.001. All other interactions were not significant at the p ≤ 0.01 level. The estimate of the covariate residual was 0.167510 and the SE = 0.008673. The estimated marginal grand mean was -0.259 and the SE = 0.016, df = 746, CI [-0.289, -0.228]. Table 3 displays the means, SEs, df, and 95% CI for the interaction of punishers and the dispense/deposit device mediated by gender. When the male participants played the game with virtual points only, the gain/loss ratio was 1.92 and for the female participants was 2.48. When the male participants played the game with virtual points plus cash, the mean gain/loss ratio was 3.21 and for the female participants was 4.04. The loss/gain ratio for all participants when playing the game with virtual points only was 2.23 and for the alternative that included cash was 3.70. The results per participant are displayed in Tables 3 and Table 4.
Discussion
In experiments, behavior-analytic researchers typically examine the behavior of a small number of individuals and use withinparticipant or within-subject experimental designs with repeated measures across multiple conditions. Moreover, the analysis of data tends to be descriptive (for example, linear regression) rather than inferential. By contrast, cognitive researchers, including Kahneman and Tversky (1979) [1], typically engage larger numbers of participants in group designs for which inferential statistical tests, such as the LMM, are used for analyzing the data.
In Experiment 1, we conducted an exploratory study of the effects of gender on the asymmetry of gains and losses using a similar procedure to that of Rasmussen and Newland (2008) [4] and found that female participants were more sensitive to losses than male participants were. Thus our findings were consistent with the conclusion drawn by economists Croson and Gneezy (2009) in their review article entitled “Gender differences in preferences: “We found that women are indeed more risk averse than men” (p. 448). Additionally, in Experiment 2, we studied the differential impact of virtual money (amounts of money displayed on a computer monitor) and actual money (which was dispensed by or deposited into a coin machine) on distributed choice. The topic is of increasing interest in a world where electronic transactions are becoming standard practice. For example, Chatterjee and Rose (2012) found that participants spent more money on products when using a credit card versus using cash. According to Prelec and Loewenstein (1998), people are more sensitive to loss when payment is made with cash rather than a credit card at the time a product or service is acquired. Thus, the use of credit cards may be mitigating in this instance. We hypothesized that the use of virtual money (points shown on a computer screen) has a similar effect namely, that loss aversion will be greater when the loss occurs as actual money rather than virtual money. In other words, the hedonic asymmetry of gains and losses is magnified when real money is in play. Given our interest in employing a single procedure to study the differential effects of gender and virtual v. actual money on loss aversion, we conducted a study in which our 26 participants included 15 females. The model for overall differences produced a highly significant difference between the No-Punishment and Punishment conditions. The estimated marginal mean for the latter was substantially greater than that for the former. The mean estimated asymmetry of losses to gains was 2.18. These values were closer to those reported by Kahneman and Tversky (2000) than to those reported by Rasmussen and Newland (2008). The mean estimated loss-gain ratios were 1.91 for male participants and 2.49 for female participants. Thus, both hypotheses 1 and 2 were confirmed.
Although the estimates of the loss-gain ratio were closer to those produced by the cognitive researchers whom we cited than by the behavior analysts, the paucity of published findings by the latter militates against drawing conclusions about the relative reliability or validity of the two approaches. The disparity between our results and those of Rasmussen and Newland (2008) [4] is readily assignable to procedural differences, including our recruitment of participants of both genders and our use of punisher conditions in which the frequency of punishers was equal to that of reinforcers (in their experiment, the latter was always higher). Our use of conjoint interdependent conc VIVI schedules also contrasted with their reliance on independent conc VIVI schedules. The agreement between the programmed ratios of reinforcers and punishers and the actual ratios was much tighter in our experiment than in theirs. With our research design, we sought to reduce the impact of extreme preferences on the actual distribution of reinforcers and punishers between the two response alternatives.
We offer no hypotheses for the gender difference we observed. Though it would be possible to cite gender differences that have been identified in other psychological subdisciplines (for example, social psychology, developmental psychology, cognitive psychology, and personality psychology), we did not measure any potential correlates. That is an obvious direction for future research, together with investigation of the differential effects of on-screen increments and decrements of points exchanged for money following the session as opposed to the actual receipt and forfeiture of money during the session. Another potential direction is the correspondence between behavioral measures of choice and electrophysiological correlates, such as the electroencephalogram. For the present, we have added evidence of human irrationality by finding asymmetrical differences between the Punishment and No-Punishment conditions as well as gender-specific differences in loss-gain asymmetry. Hypothesis 3, namely, that participants would exhibit greater loss aversion when playing the game using the dispense/deposit device, was confirmed (see Table 2).
These findings were inflected by gender. Male participants exhibited increased loss aversion when they played the game using virtual points plus cash in comparison to virtual points only. However, female participants exhibited an even greater increase in loss aversion.
Conclusion
Our results, namely, that losses weighed more heavily than gains did and in a direction opposite that of gains, confirmed those of Kahneman and Tversky (1979) and of Rasmussen and Newland (2008). Differences in methods and the data-analytic models, however, rule out a meaningful comparison between the approximations of gain/loss asymmetry reported by Kahneman and Tversky (2000) and Rasmussen and Newland (2008) and those we obtained. Moreover, those researchers did not report gender effects, whereas we found that loss aversion was 29% greater in females than in males in the virtual points-only condition and 26% greater for females than in males in the virtual points-pluscash condition. Loss aversion increased when the game was played using the coin dispenser/collector in addition to onscreen (virtual) points exchangeable for money. This finding was consistent with the assertions by Prelec and Loewenstein (1998) and of Kahneman and Tversky (1979) [1] that losses loom larger than gains. Future studies may take further factors in this differential as their object.
Acknowledgement
None.
Conflict of Interest
No Conflict of interest.
References
- Kahneman D, Tversky A (1979) Prospect theory: An analysis of decision under risk. Econometrica 47(2): 263-291.
- LeBoeuf RA, Shafir EB (2005) Decision making. In: KJ Holyoak, RG Morrison (Eds.), The Cambridge handbook of thinking and reasoning. Cambridge, Cambridge University Press, UK.
- Kahneman D, Knetsch JL, Thaler RH (1991) Anomalies:The endowment effect, loss aversion, and status quo bias. Journal of Economic Perspectives 5(1 Winter): 193-120.
- Rasmussen EB, Newland MC (2008) Asymmetry of Reinforcement and Punishment in human choice. Journal of the Experimental Analysis of Behavior 89(2): 157-167.
- Charness G, Gneezy U (2012) Strong evidence for gender differences in risk taking. Journal of Economic Behavior and Organization 83(1): 50-58.
- Thorndike EL (1911) Animal intelligence. New York, NY: Macmillan, USA.
- Baum WM (1974) On two types of deviation from the matching law: Bias and undermatching. Journal of the Experimental Analysis of Behavior 22(1): 231-242.
- Deluty MZ (1976) Choice and rate of punishment in concurrent schedules. Journal of the Experimental Analysis of Analysis of Behavior 25(1): 75-80.
- de Villiers PA (1977) Choice in concurrent schedules and a quantitative formulation of the law of effect. In: WK Honig, JER Staddon (Eds.), Handbook of operant behavior. Englewood Cliffs, NJ: Prentice-Hall, USA, pp. 233–287.
- de Villiers PA (1980) Toward a quantitative theory of punishment. Journal of the Experimental Analysis of Behavior 33(1): 15-25.
- Mazur JE (2006) Mathematical models and the experimental analysis of behavior. Journal of the Experimental Analysis of Behavior 85(2): 275-291.
- Miller HL (1976) Matching-based hedonic scaling in the pigeon. Journal of the Experimental Analysis of Behavior 26(3): 335-347.
- Kahneman D, Tversky A (2000) Choices, values, and frames. In D. Kahneman, & A. Tversky (Eds.), Choices, values, and frames. Cambridge, UK: Cambridge University Press, UK.
-
Diego G Flores*, Harold Miller, Jr., Bruce Brown, Chongming Yang, Michael Seeley, Darin Costello, Frank Robertson, Marcia Ventura and Blake Hansen. Behavioral Measures of Gain-Loss Asymmetry as a Function of Gender and of Virtual v. Actual Money. Arch Neurol & Neurosci. 15(2): 2023. ANN.MS.ID.000857.
-
Behavioral, Measures, Gain-Loss, Asymmetry, Psychology, Cognitive studies, hypothetical scenarios, behavior-analytic, Humans; Video game; Concurrent variable-interval schedule; Gain (reinforcer); Loss (punisher); Gain-loss asymmetry gender difference; Prospect theory; Gain/loss asymmetry; Loss aversion
-
This work is licensed under a Creative Commons Attribution-NonCommercial 4.0 International License.