Research Article
On Some One Parameter Lifetime Distributions and their Applications
Vijay Sharma
Department of Mathematics, B.B.M. College, India
Ravi Shanker
Department of Mathematics, N.P University, India
Rama Shanker*
Department of Statistics, Devendra Mishra Institute of Statistics, India
Rama Shanker, Department of Statistics, Devendra Mishra Institute of Statistics, Patna, India.
Received Date: September 11, 2019; Published Date: September 18, 2019
Abstract
The analysis and modeling of lifetime data are crucial in almost all applied sciences including medicine, insurance, engineering, and finance, amongst others. In the present paper an attempt has been made to have a comparative study on statistical properties and applications of some wellknown one parameter lifetime distributions available in statistics literature to model lifetime data from biomedical science and engineering. This comparative study would be of great help to scientist working in biomedical science and engineering for modeling waiting time and survival time data in their respective fields of knowledg.
Keywords: Lifetime distributions; Index of dispersion; Statistical properties; Estimation of parameter; Applications
Introduction
The time to the occurrence of event of interest is known as lifetime or survival time or failure time in reliability analysis. The event may be failure of a piece of equipment, death of a person, development (or remission) of symptoms of disease, health code violation (or compliance). The modeling and statistical analysis of lifetime data are crucial for statisticians and research workers in almost all applied sciences including engineering, medical science/ biological science, insurance and finance, amongst others. The classical lifetime distribution namely exponential distribution and Lindley distribution introduced by Lindley (1958) distribution are popular in statistics for modeling lifetime data. But these two classical lifetime distributions are not suitable from theoretical and applied point of view. Shanker et al (2015) have done a critical and comparative study regarding the modeling of lifetime data using both exponential and Lindley distributions and found that there are several lifetime data where these classical lifetime distributions are not suitable due to their shapes, hazard rate functions and mean residual life functions, amongst others. Recently, a number of one parameter lifetime distributions have been introduced by Shanker, namely Shanker, Akash, Rama, Suja, Sujatha, Amarendra, Devya, Shambhu, Aradhana, and Akshya, respectively. The probability density function (pdf), cumulative distribution function (cdf) one parameter lifetime distributions along with their introducer and years are presented in the following Table 1.
The statistical properties and some applications of these distributions have been given in the respective papers.
Table 1:

Dispersion of One Parameter Lifetime Distributions
The conditions of dispersion of one parameter lifetime distributions for values of the parameter θ have been presented in Table 2.
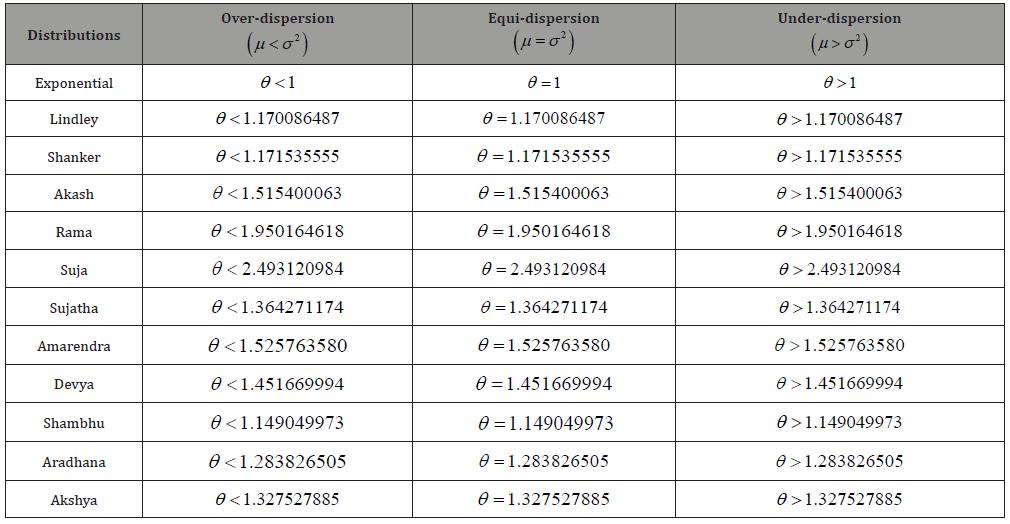
Maximum likelihood estimation of parameter of one parameter lifetime distributions
Assuming as a random sample of size n from the
respective distribution, the log likelihood function to find maximum
likelihood estimate (MLE) of parameter of one parameter lifetime
distributions is presented in the following table 3. Note that these
log likelihood function have been maximized using R software for
the MLE of parameter of respective distribution (Table 3).
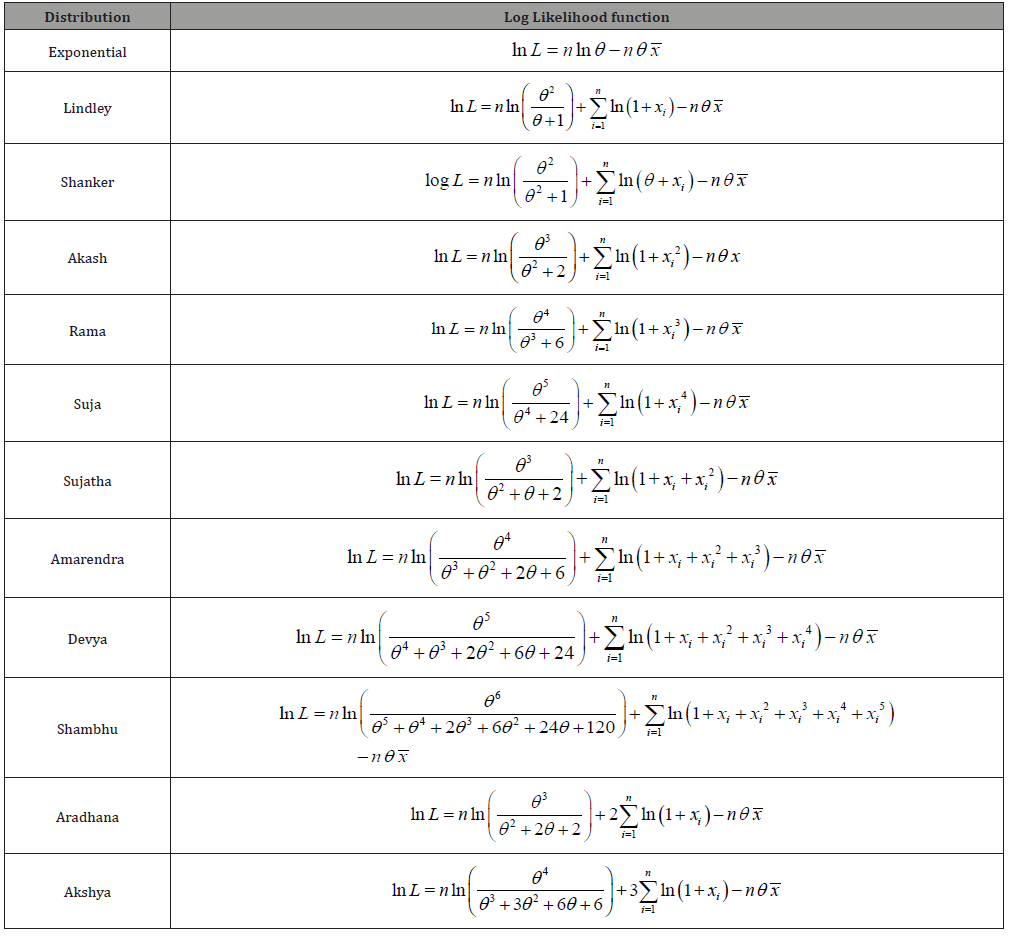
Applications
The one parameter lifetime distributions have been fitted to a number of real lifetime data to test their goodness of fit. The goodness of fit of these one parameter life time distributions based on maximum likelihood estimates are presented in this section.
In order to compare one parameter life time distributions values of −2ln L , AIC (Akaike Information Criterion), K-S Statistics ( Kolmogorov-Smirnov Statistics) for all five real lifetime datasets have been computed. The formulae for computing AIC and K-S Statistics are as follows:
where k the number of parameters, n is the sample size and (Fnx ) is the
empirical distribution function. The best distribution corresponds
to lower values of −2ln L , AIC and K-S statistics. The following real
lifetime datasets have been considered.
Data set 1: This data set represents the lifetime’s data relating to relief times (in minutes) of 20 patients receiving an analgesic and reported by Gross and Clark (1975, P. 105).

Data Set 2: This data set is the strength data of glass of the aircraft window reported by Fuller et al (1994):

Data Set 3: The following data represent the tensile strength, measured in GPa, of 69 carbon fibers tested under tension at gauge lengths of 20mm (Bader and Priest, 1982):
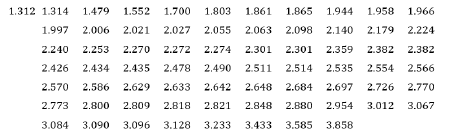
The ML estimates, −2ln L , AIC , K-S and p-value of the respective distribution for data sets 1, 2, and 3 have been presented in tables 4, 5, and 6. The best distribution has been marked with bold ( Tables 4-6).
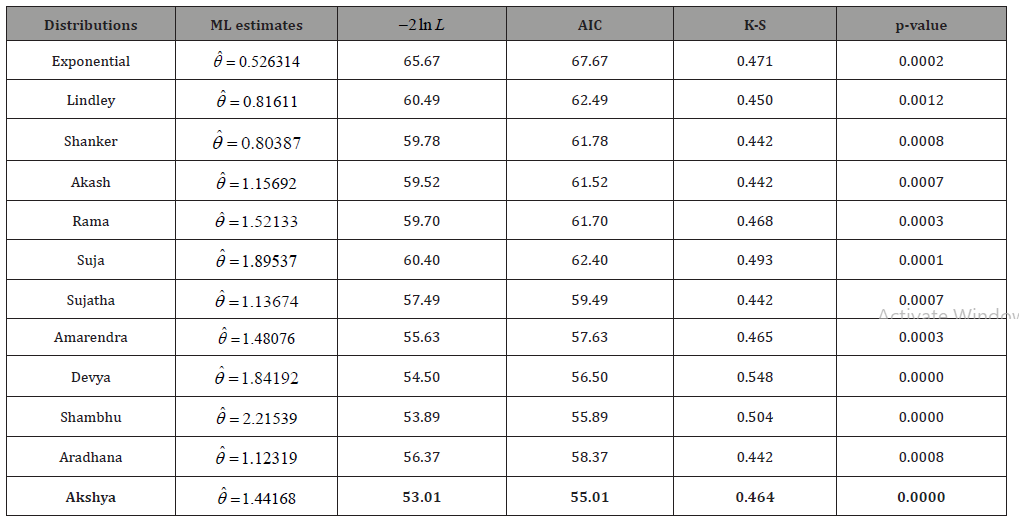
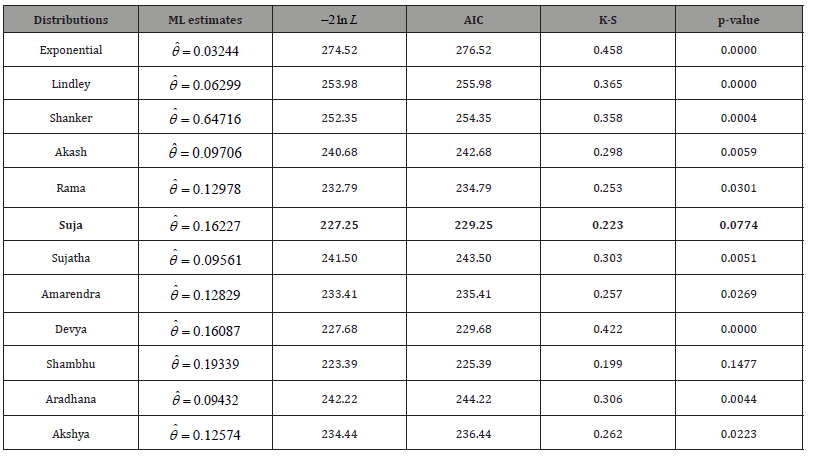
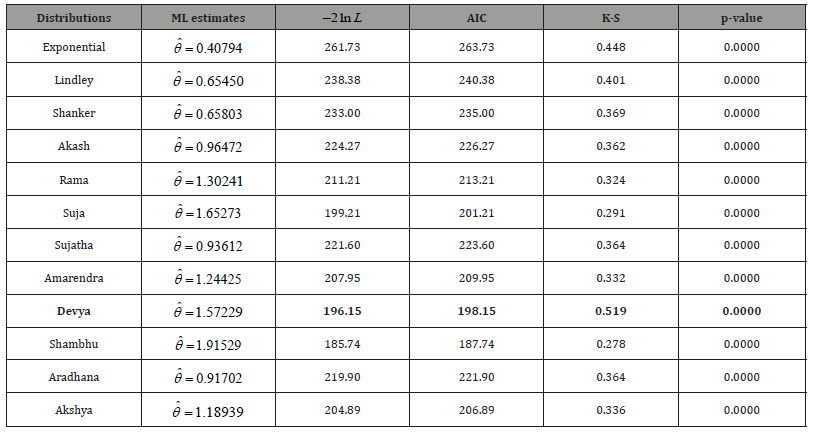
Concluding Remarks
The analysis and modeling of lifetime data are crucial in almost all applied sciences including medicine, insurance, engineering, and finance, amongst others. In the present paper three examples of observed real lifetime datasets have been considered for testing the goodness of fit of various one parameter lifetime distributions. The index of dispersion of these one parameter lifetime distributions has been presented which will give idea about the distribution to be used as per the dispersion of the data. This study would be of great help to scientist working in biomedical science and engineering for modeling waiting time and survival time data in their respective fields of knowledge.
Acknowledgement
None.
Conflict of Interest
No conflict of interest.
References
- Bader MG, Priest AM (1982) Statistical aspects of fiber and bundle strength in hybrid composites. In: hayashi T, Kawata K, Umekawa S (Eds), Progressin Science in Engineering Composites, ICCM-IV, Tokyo, pp. 1129-1136.
- Epstein B (1958) The exponential distribution and its role in Life-testing. Ind Qual Control 15: 2-7.
- Fuller EJ, Frieman S, Quinn J, Quinn G, Carter W (1994) Fracture mechanics approach to the design of glass aircraft windows: A case study, SPIE Proc 2286: 419-430.
- Gross AJ, Clark VA (1975) Survival Distributions: Reliability Applications in the Biometrical Sciences, John Wiley, New York.
- Lindley DV (1958) Fiducial distributions and Bayes’ Theorem, Journal of the Royal Statistical Society, Series B 20: 102-107.
- Shanker R (2015 a) Shanker Distribution and Its Applications, International Journal of Statistics and Applications, 5 (6), 338 – 348.
- Shanker R (2015 b) Akash distribution and Its Applications, to appear in International Journal of probability and Statistics, 4(3).
- Shanker R, Hagos F, Sujatha S (2015) On modeling of Lifetimes data using exponential and Lindley distribution Biometrics & Biostatistics International Journal 2(5): 1-9.
- Shanker R (2016 a) Sujatha Distribution and Its Applications, Statistics in Transition-New series 17(3): 391- 410.
- Shanker R (2016 b) Amarendra Distribution and Its Applications, American Journal of Mathematics and Statistics 6(1): 44 – 56.
- Shanker R (2016 c) Devya Distribution and Its Applications, International Journal of Statistics and Applications 6(4): 189- 202.
- Shanker, R. (2016 d): Shambhu Distribution and Its Applications, International Journal of Probability and Statistics 5(2): 48-63.
- Shanker R (2016 e) Aradhana Distribution and Its Applications, International Journal of Statistics and Applications 6(1): 23-34.
- Shanker R (2017 a) Rama Distribution and its Application, International Journal of Statistics and Applications 7(1): 26-35.
- Shanker R (2017 b) Suja Distribution and its Application, International Journal of Probability and Statistics 6(2): 11-19.
- Shanker R (2017 c) Akshaya Distribution and its Application. American Journal of Mathematics and Statistics 7(2): 51-59.
-
Clement Boateng Ampadu. The Cot-X Family of Distributions with Application. Annal Biostat & Biomed Appli. 3(1): 2019. ABBA. MS.ID.000556.
Trigonometric functions; Statistical distributions; Breast cancer; Odds T-X Contents
-
This work is licensed under a Creative Commons Attribution-NonCommercial 4.0 International License.