Research Article
Enhancing User Experiences in Ai-Driven Decision-Making
Marran Aldossari*
Department of Computer Sciences, Shaqra University, P.O. Box 11991, Shaqra, Saudi Arabia
Marran Aldossari, Department of Computer Sciences, Shaqra University, P.O. Box 11991, Shaqra, Saudi Arabia
Received Date:May 23, 2024; Published Date:June 07, 2024
Abstract
This study examines user experiences in AI-aided decision-making where system accuracy, transparency, and ser interface design are the foci. Drawing upon existing literature and the synthesis results, it is evidenced ow crucial it is for developers to ensure precision and reliability, promoting user satisfaction. Transparency in decision-making processes that require adherence to moral obligations introduces a basis for user trust and ethical factors, while interaction design becomes an agent of overall satisfaction. Traditionally, user experiences present a dynamic picture that is shaped and reshaped by changing trends and cultural factors, which exposes the concept of vigilance as one that needs to be constantly attentive. The recommendations suggest that value on the protocol of accuracy and transparency, user-centric design, monitoring of new trends, reduction of research constraints, and collaboration between disciplines is preferred. To summarize, this research provides critical knowledge that is fundamental in improving the performance of AI systems to meet user needs and also demonstrates ethics and adaptability to emerging technological settings.
Keywords:AI; Decision-making; AI-aided decision-making; User experiences; HCI
Introduction
Background
Artificial intelligence has brought about a new dimension in problem-solving, transforming decision-making across various range of domains (Shehzad et al., 2022) [1]. Several decisionmaking tools have formed AI-aided systems, which are now regarded as primary influential leaders that increase accuracy and efficiency in making decisions. According to Shehzad et al. (2022), AI technology has grown rapidly in the last decade, with innovation providing surprising discoveries across many technologies - from powerful machine learning algorithms to complexity within neural networks. These innovations have spurred the development of AI systems that can perform more complicated tasks than human beings, such as image recognition, natural language processing, and decision-making. The application of AI to the decision-support processes has resulted in an era that promises unprecedented benefits on quality and timeliness regarding any positive decisions. With the AI analytic tools in place, businesses from various fields are able to use oceans of data for information purposes, which will lead to informed decision-making and how people make decisions. This study examines the user experience environment associated with artificial intelligence and decision support to reveal different peculiarities that affect a person while interacting within this type of system.
This observable growth of AI in the decision-making process is evident through its widespread application by many different industries, including finance, the healthcare sector, and manufacturing logistics. AI algorithms shine the most in the financial field by helping uncover complex market patterns and control investment portfolios and risk management decisions. AI in the health sector aims to improve diagnosis by increasing accuracy, individualized treatment schedules, and drug production to transform patient management. Manufacturing companies gain by reducing production lead time and streamlining operations, predicting failure with equipment breakdowns, and using supplychain management provided through AI. In logistics, AI allows algorithms that better route optimization, inventory control, and demand forecasting, enabling firms to respond rapidly as they face changing market conditions. In this regard, AI-informed decision support systems are essential in quickly handling big data and detecting advanced patterns and forecasts. These findings are especially invaluable for uses where immediate actions based on vast information flows decisively determine whether success or failure is achieved. This general acceptance represents AI’s adaptability and effectiveness in confronting several issues that may differ among fields.
The emergence of AI technologies helps an increase in usercentric design. The growing prevalence of AI is the reason behind prioritizing the issues related to user experience, focusing on technologically superior yet intuitive and ethically sound systems. In this context, user-centered design is a critical approach that recognizes the importance of users in implementing and adopting AI systems. The essence lies not only in improving usability but also regarding ethical considerations resulting from AI applications like transparent processes and accountability for decisions made on the basis or mechanisms addressing bias that is inevitable even when producing algorithms. With the integration of AI technologies into different areas of people’s lives, only user-centric design practices can ensure synergy between technical innovations, ethical concerns, and user satisfaction.
Amidst the several benefits of AI-enabled decision-making, there is a need for deep insight into understanding how humans interact with such complicated systems better. This research creates an opportunity to understand the controversial area of user experience under AI decision support. Through systematic research, the study helps understand the drivers of positive and negative user experiences so that they can obtain valuable but subtle knowledge for facilitating significant implementation of AI systems. This aims to contribute towards developing new AI systems that match users’ expectations and needs, thus making it possible for them to use this kind of decision-making judgment appropriately. However, the contribution of this study does not lie only in theoretical concepts but also acts as a guide for developers, policymakers, and organizations in seeking where they should turn when there are more AI integration expansions to come.
Significance of the Study
The main implication of the study into User Experience in AIAssisted Decision Making is exploring the various implications of optimising systems designs. As artificial intelligence is still advancing and changing how decisions are made in multiple ways, understanding user behavior becomes a prerequisite. This paper is the greatest navigational tool for developers as it unpacks factors that either increase or reduce user satisfaction. Armed with such knowledge, developers can meticulously fine-tune their designs in order to achieve perfect compatibility with AI-based decision support systems at the level desired by users. System design optimisation based on user experience evidence from empirical studies improves the performance and accuracy of such systems and helps them be applied in different industries, thus promoting innovation. Moreover, this study not only extends to technology development but also reaches other stakeholder groups, including policymakers, organisations, and users. By rendering the user experience landscape visible, this research gives policymakers some insight into the ethical issues and dilemmas that arise from AI deployments. This knowledge, derived from practical facts about the real world, enables policymakers to make rules that promote innovation alongside responsible AI use. The study provides a strategic instrument for organization’s user satisfaction and strategy management leadership individuals who integrate AI technologies. That is, the value of this study lies in its potential ability to revolutionize AI integration’s landscape through usercentered design data collection, addressing ethical issues and contributing toward informed decision-making systems based on artificial intelligence.
Another central significance of this study is its direct evaluation and optimisation for quality improvement toward user satisfaction in an AI-based decision support environment. To comprehensively analyse the total level of user satisfaction, systematic reviews of users’ experience will be reviewed. This gives the developers an insight into other aspects that make up a good user and leads them to focus on certain features or functionalities. This sort of realisation ensures target optimisation, which brings user satisfaction. Results that emerged from this section of the research will be instrumental to developers in establishing an approach by which they develop AI systems based on what users want. The study can serve as an introduction to the continued use of such systems among different industries regarding the underlying relationship between user satisfaction and successful AI implementation. Also, the study can act as a guide for AI policymakers. The policymakers are key in spearheading AI deployment’s ethical and regulatory maze. This study is invaluable to policymakers since detailed information about user experiences on using AI-based systems in decisionmaking can be provided. Regulatory undertakings grounded on the data gathered through such research can guide policymaking to encourage ethics and responsible use of AI. The user perspective knowledge is highly sophisticated to ensure that the rules are not only cutting-edge but also mitigate problems associated with transparency, accountability, and bias. Specifically, the research serves as a kind of guidance for authorities and helps to issue principles that are based on creating harmony between progressive technology and moral ones. Through actively constructing the regulating context, this research contributes to socially acceptable and reasonably well-balanced implementations of AI technologies in decision-making at higher societal levels.
Moreover, this study aids in addressing ethical concerns related to AI-assisted decisions. There are a number of considerations that surface in the arena of ethics for AI deployment, including transparency, accountability, and minimising bias. This research is key to addressing these ethical issues in elaborating how users understand and use an AI system. As evidenced by empirical analysis, the study promotes transparent and accountable AI systems that are essential for building trust amongst users. The study, thus, illuminates the ethical dilemmas in decision-making algorithms to assist organisations and policymakers with valuable information that can serve as a guide on innovation without compromising ethics. Consequently, the study plays an active part in creating a suitable ethical system required for the safe implementation of AI technologies at a societal level. Also, this study aims to act as a guide for enhancing user-centric design. The growth in focus on human-centered design approaches accompanies the development of AI technologies. In this respect, the study under discussion becomes highly relevant as it informs of user experience to improve the principle design from a user perspective. Establishing user preferences, challenges, and expectations revealed by the research enables the system development to incorporate these insights. This guarantees that AI technologies fulfill functional needs and match users’ roles and appreciation. Effectively, the study catalyses AI systems that focus primarily on end-user experience development, making one feel more intuitive when dealing with decision-support technology.
Study Objectives
This research aims to focus on several aspects of the user experience in AI aided decision making. Generally, it aims to discover and measure the general user satisfaction with artificial intelligence share decision-making. The research attempts to determine and analyze the crucial features that significantly shape the users’ experiences with a focus on use of AI in decision processes and decision processes surrounding the use of AI. In addition, the study aspires to measure the impact of vital factors such as system accuracy, user interface design, and transparency on user satisfaction. The study further aims at identifying possible correlations between individual attributes among users, such as their degree of familiarity with AI and technical skill, and their perceived performance when using an AI-guided decision-making system. This multidimensional approach allows for a thorough analysis of multiple nature of user satisfaction and experience in the field of AI-based decision support.
Literature Review
The broad literature on User Experience (UX) in AI-assisted decision-making provides a complex framework that interlaces various aspects presented by the changing technological capabilities, human behavior, and ethics. This literature represents a multi-sided inquiry, providing deep thought into the dynamic between users and AI-tailored decisions.
Evolution of AI in Decision Making
The evolution of AI integration in the decision-making process traces back to primitive rule-based systems, unlike the current state modernised by powerful machine learning algorithms (Duan et al., 2019) [2]. According to Duan et al. (2019), in the initial stages of AI, decision-making processes were guided by explicit rule sets. These pioneering AI systems, however impressive, may have been due to their overreaching vision of a flexible model based on cumulative behaviour change that lacked adaptability. Chances to cope with complexities and diversification features alone among ruleoriented kinds face an inevitable diminution in their capabilities to contend with these conditions because of the deterministic nature of the set standards. The history of AI’s emergence experienced a breathtaking era with the invention of machine learning, which was a turning point regarding decision-making powers (Delipetrev et al., 2020). Delipetrev et al. (2020) [3]. highlight that machine learning algorithms brought in a progressive and responsive consideration, causing programs to discover the data examples. This liberty from the explicit rule set to a data-driven principle has greatly increased and widened the application and efficacy values of artificial intelligence decision context. From this point onward, one may identify autonomy in meaning patterns and relationships among them inside large data sets as a symbol of the evolution that took place, an era where AI systems can overcome limitations imposed by a rule-based approach.
A different study by Ouyang and Jiao (2021) [4]. presents that in the contemporary AI-driven decision-machine world, deep learning can serve as a legitimate milestone in this development process. However, deep learning algorithms were structurally and operationally based on the connectionist network structure, which excelled so controversially with complex representations. According to Ouyang and Jiao (2021), AI systems with deep learning functional conducts using multiple-layered neural architectures can recognise complicated data patterns and features without support. It has made this ability so evolutive since it greatly improved AI decision-making powers and made such software capable of functioning in areas where data did not fit into specific structures. Since AI is a supporting decision-making tool, it slowly but surely continues even to this day, which is why understanding such progress by itself can be an equally important part of connecting the contemporary situation with its historical context. The path of evolution from rule-based systems to machine learning and ending in deep learning highlights the transition of decision dynamics from rigid but concise to complex yet flexible. Instead, this change is more than capable in its ability to demonstrate technological progress; it lays out a foundation for grasping complexity and future possible complications, thus being inherent in modern AI systems. As the storyline of this evolution grows, it becomes apparent that AI innovation development in decision-making is a technological transformation through which modern artificial intelligence tools have become necessary for managing difficult triggers.
User-Centric Perspectives in AI
A key transformation highlighted in the literature is the visible change to user-oriented design principles as AI development takes place (Cronholm & Göbel, 2022; Cirqueira, Helfert, & Bezbradica, 2021) [5,6]. This paradigmatical shift points to an increasing awareness of users’ critical contribution towards acceptance and success by using AI technologies. With the continued prevalence of artificial intelligence across many industries, the literature repeatedly stresses that UX should be provided with primary emphasis. Replacing the status quo with another is no cosmetic adjustment; rather, it constitutes a fundamental realignment with an anti-thesis principle whereby consideration for the user should be given particular focus in AI design and implementation at every point.
A study conducted by Varghese et al. (2022) [7] resonates with a clear message: With increasingly embedded AI technologies in different realms of human life, IAA’s changeability and modifiabilities become normative for the understanding/adaptation process to user expectations, preferences, or cognitive capacities. Varghese et al. (2022) study on the user-centric approach is based upon the idea that/ claims to reach a primary goal by introducing a system that easily embeds into users’ cognitive processes and a part of their lifestyle. Such recognition exceeds the functional side of AI and touches upon human thinking and representation as being in flux when interacting with something or someone. Despite the significant efforts scientists put into finding effective ways to guide their development of AI systems, scholars point out a lack of proper alignment with user expectations and preferences (Gabriel, 2020; Haleem et al., 2022) [8,9]. In this regard, the alignment of capacities associated with human beings, namely logical reasoning, and understanding, among others, find utility as a linchpin for ensuring that AI technologies have excellent decision-making abilities that unfold conveniently. It is postulated in the literature that usercentered design principles, which are at the heart of improving overall end users’ satisfaction, have implications for the successful adoption and sustained usage of AI applications.
Essentially, the meaning of literature that converges on some increased chances for a complicated bridge between AI meanings and those varieties of cognitive landscape users. Through collaborating user-oriented perspectives into the introduction and use of AI systems, developers are positioned to develop solutions that, apart from being technically ingenious, can, at best, be analogous with the perceptions informed by users themselves. This transformation is not only about improving the performance of AI in processes and responses but also about providing a positive content experience, which contributes, among other things, to making these technologies accessible, transferrable, adaptive, and profitable as an application range for several industries.
Factors Influencing User Experiences
The critical literature investigation has led to exploring the complicated factors that determine user experience in decisionmaking using artificial intelligence. The research underscores the intricacy of these aspects, but attention is drawn to their mutual influence on user contentment and successful deployment. First of all, the most important feature of system accuracy is that it always correlates with positive experiences because human beings play an explicit role (Semerádová et al., 2020; Deng et al., 2015) [10,11]. The effect of AI-aided decision-making systems’ accuracy level on user confidence and trust highlights how strong it is for shaping a general perception of satisfaction with such products. In a different study by Nagaraj and Bryant (2016), [12] transparency emerges as an important keystone in user experience for research after research has confirmed its formidable role in users’ perceptions. Transparent decision-making mechanisms and models that interpret AI algorithms are critical enablers of user trust. According to Nagaraj and Bryant (2016), when users understand and can trace back how AI technology has been used in decision-making, they are more willing to take on a positive attitude towards it. The openness of systems not only increases users’ awareness and their level of understanding but also leads to trust formation, an integral part of building proper user experience in the AI environment where it provides support.
More importantly, the role of user interface (UI) design in shaping overall UX cannot be overemphasised as a constant theme across the literature (Pratama & Cahyadi, 2020) [13]. A user-friendly interface is a critical aspect of the development process that enhances usability and allows for interaction with AI systems. According to Pratama and Cahyadi (2020), the visual characteristics and practical elements that make up the user interface (UI), which encompass the intuitive design of its use, are integral to how people navigate through AI-driven decision support concepts. The literature emphasises that a well-thought-out UI not only provides good interaction but also, to some extent, increases user satisfaction when using AI technologies. The working of these elements together constitutes a much-composited ecosystem under which the user experience occurs. The literature highlights the importance of balancing three elements-the accuracy of a system, its transparency, and the design choices within the user interface – to create a pleasant and enriching experience for a user. The subtle interplay of these factors shapes users’ attitudes toward AI-aided decision-making systems as reflected through their confidence levels, satisfaction and overall acceptance of the technology. Simply put, the literature brings out an issue related to the holistic consideration of these influencing factors for successful AI technology integration into decision-making procedures in multiple sectors.
Conceptual Framework
The conceptual framework below is based on the conducted literature, showing key themes.
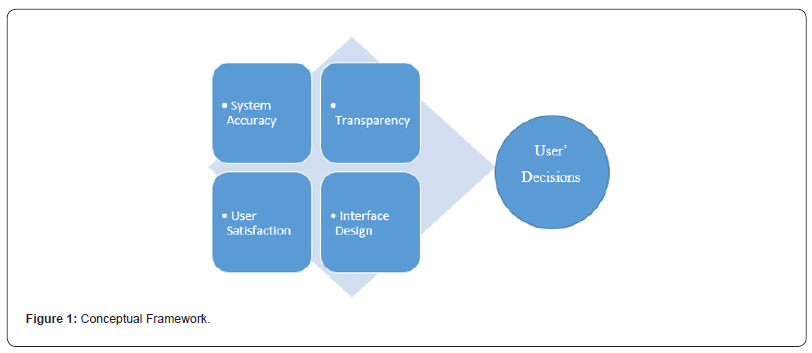
Research Methodology
This study utilises a mixed-methods research methodology, drawing upon quantitative and qualitative data collection approaches to offer an in-depth perception of users’ experiences with the technology that helps automatise decision-making. Based on the nature of this research, secondary data gathering is used with insights from large volumes of scholarly literature, academic journals and respectable sources on AI, human-computer interaction decisions and science.
Study Design
The study used a mixed-methods approach to recognise the broad nature of research questions. Integrating quantitative and qualitative approaches allowed a more thorough but less focused study of the subject under analysis. The fusion of both methods permits findings triangulation, which increases the validity and reliability of data. The quantitative approach involved systematic analysis of numerical information received during researchrelevant studies, so this data could be analyzed in numbers and offer such ratios as patterns, relationships or trends related to user experiences using artificial intelligence within a decisionmaking process. Techniques like regression analyses will be used to determine the relationships between variables. Simultaneously, the object of analysis on the qualitative level involved a thematic description of text material in selected studies, including interviews and open-ended survey answers. With this qualitative approach, in-depth insights into what influences user satisfaction have been revealed.
Secondary data collection was used to conduct an in-depth review of contemporary literature, academic works and reliable sources available through AI human-computer interaction. It is indispensable to use various resources such as databases, which allowed for accumulating valuable info from a wide range of research papers, resulting in more comprehensive knowledge about how people use AI in decision support. Since the study adopted secondary data, no participants actively participated in obtaining instant loans. However, the study builds on common experiences outlined by many investigators and scholars who have discussed user effects in AI-influenced decision-making from various angles. This technique gives a broad generalised insight across studies or research works.
Data Analysis
The quantitive data from relevant studies undergo statistical analysis. This entailed conducting statistical tests to quantify relationships between left variables and descriptive statistics for summarising the crucial quantum results. In turn, the quantitative analysis intends to uncover patterns and correlations in the extant literature about user experiences of AI-assisted decision-making. On the other hand, the qualitative data undergoes thematic analysis. This qualitative data included unstructured content such as the text, interviews, and open survey responses. In a qualitative approach, combining recurring themes and careful insights leads to identifying factors influencing user satisfaction with AI-assisted DSS.
Ethical Considerations
As the study used secondary data, the ethical concerns primarily focused on the specialized treatment of data acquired from preexisting sources. A main ethical concern was citing the source to avoid plagiarism by recognizing that those authors and researchers are presenting their findings. As a contribution to academic integrity, it is essential in this study that we recognize the legacy of those scholarly contributions, which were their intellectual predecessors. However, the high priority here was given to citation practices that are essential to ensuring ethical standards of academic discourse and safeguarding the intellectual property rights of researchers whose work serves as a foundation for this research.
Further, ethical compliance prevailed in observing the ethics adopted by the original studies, including secondary data analysis. This involved an organized examination of the primary researchers’ ethical framework, consent processes adopted, and their data protection procedures. Following such standards and respecting these guidelines were critical in preserving the legitimacy of information and ensuring that a study was grounded on ethical rules set by broader academia. There was also a need to be transparent, navigating and recognizing the ethics of ethical concerns governing such original studies for trust to take root and establish what it knew and how values are sought.
Findings
Introduction
An intricate overview of the factors determining users’ experience when making AI-backed decisions is derived from various secondary data sources. Existing studies have shown that, by incorporating insights from other authors, the concept on which it is based can be further understood and developed to cover particular areas of emphasis, such as those that are critical in creating satisfaction among consumers within this dynamic environment.
Identified Secondary Sources
Table 1:Identified Key Sources.
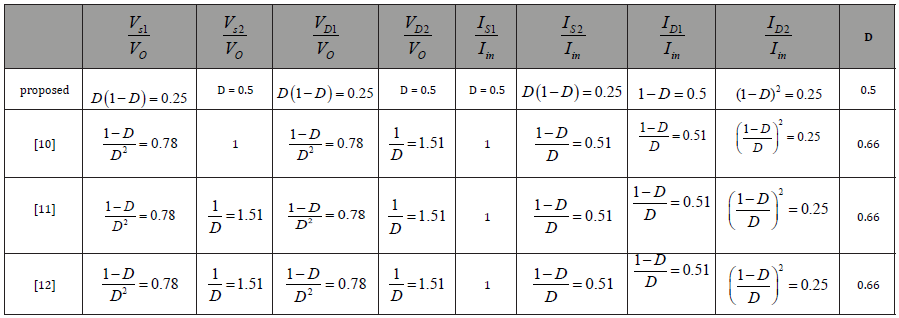
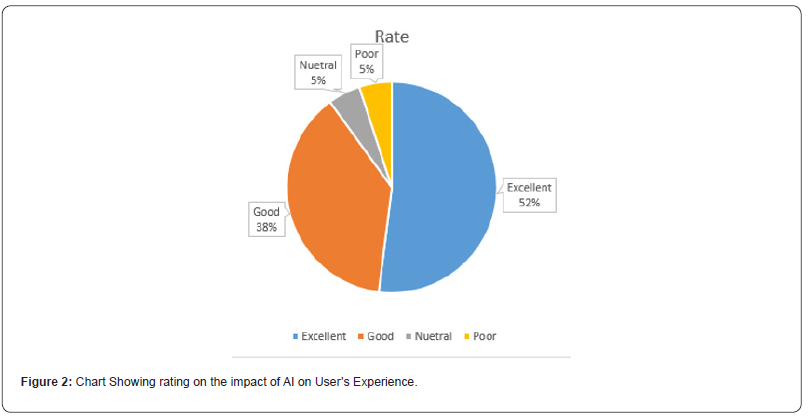
Impact of AI on Systems’ Accuracy
The evidence of the role system accuracy that is revealed in the synthesised research clarifies the essential function played by the accuracy of AI-based decision-making systems to determine users. This theme is recurring, ultimately implying that the users often favor a certain preference for systems whose outcomes are highly accurate (Zhang et al., 2020; Tajmir et al., 2019) [14,15]. Accuracy in the world linked to AI-based decision support encompasses a system capable providing results that closely conform to the correct or desired outcome with a limited margin of error. The literature consistently says that satisfaction ensues when interacting with AI systems with a reputation for providing accurate and guaranteed results on numerous occasions. This satisfaction is based on the notion users have about the accuracy of closing decisions, creating an atmosphere of trust. If the users can be confident in that AI system will give them valid outcomes, they will enjoy this digital form more than the human one because when you make something reliable your mind works differently. The ability of AI systems to make accurate predictions does not end in user satisfaction since errorless work is also inherent in the efficacy and reliability of decision-making procedures. It is no doubt that human users widely accept AI technologies once they perceive high precision and rigorousness when used in decision-making, which can have huge consequences for the user involved. This finding highlights the catalytic nature of accuracy as a basis for establishing trust and confidence with users in automated decision-making. Based on the chat above, 52% of the articles collected showed that AI accuracy significant impacts the users’ satisfaction.
Transparency in AI
The importance of transparency in study synthesis indicates that this factor plays a crucial role in defining how the user responds towards satisfaction, perceived as dependent on artificial intelligence-driven decisions. Transparency means users’ ability to understand how the systems reason and predict certain outcomes based on well-known algorithms as factors triggering such actions (Balasubramaniam et al., 2022) [16,17] Larsson and Heintz (2020) The studies throughout the literature base stress that transparency in decision-making procedures and comprehendible AI models to a high extent promote user trust. The transparency behind AI in the decisions that it arrives at is essential, as a lack of such transparency may have ramifications on both low-stakes and highstakes situations. Transparency is a median between the dark machinery of AI algorithms, which delivers massive amounts of data and produces decisions that are often bewildering to users.
One of the main conclusions in studies by Andrada et al. (2023) and Felzmann et al. (2019) [18-20] is that AI systems directly influence the level of trust in their use and in users’ experience with these systems. Especially in situations where AI impacts critical decisions, it makes people likely to end up being more comfortable with the decision-making process if they understand the logic of such making. The power to interpret why AI produced the results it did is a positive aspect located by users to contribute to an individual’s sense of control and understanding. This can significantly impact AI-assisted decision-makers, developers, and designers. It highlights the need to promote transparency in design as far is concerned, and it should be clear that the decision-making operations are not complex but simple, and relevant to end-users. This can include building interfaces that explain the AI decisions or implement interpretable machine learning models by which features are identified; they influence results. The diagram below highlights the different key aspects collected from different articles, relating to how AI transparency impacts users’ experience.
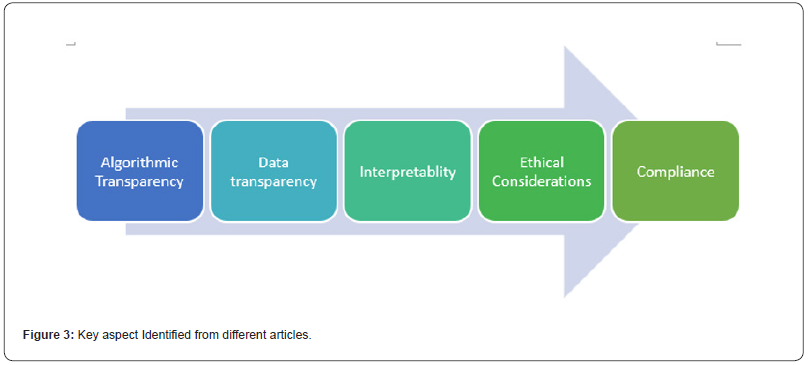
Role of AI on User Experience
An underlying role that has been a notable, constant theme to emerge from the literature synthesis is User Interface (UI) design as an important driver of user satisfaction. UID refers to all aspects of the visual and interactive factors these users come across when interacting with AI-powered decision-making systems. A cursory review of relevant literature notes that properly designed user interfaces are characterised by features such as intuitiveness and usability, which tremendously enhance these systems’ overall usability (Han et al., 2023; Stige et al., 2023) [21,22]. The findings of these studies continue to show that both the appearance and functional UI elicits positive behaviour in AI elements. In turn, a user-friendly interface combined with a design that allows it to navigate with ease and understanding it is an important part of giving people a good experience. The user’s contentment is bolstered not only by the AI-based decision-making made by people but also when they feel that the system interface appears to be natural and comfortable, as it can enhance the effectiveness of interactive aspects [23].
The focus on user-focused design right spins and designing AIbased decision interfaces for assistors is essential. This includes modification of the design to reflect proper cognitive processes as well as the expectations of users. Interfaces focusing on intuitive Friendliness have made the customer curve easier, making their interaction clearer and more effective (Dudley & Kristensson, 2018) [24]. In the decision-making process, this user-centric approach is paramount as one can note that the interface’s accuracy and simplicity greatly impact users’ confidence while also enhancing their satisfaction. In a real sense, developers and designers ought to think of user-centric design principles along the implementation life cycle stages of AI systems. They involve conducting user tests, receiving feedback, and, in an iterative manner, revising the UI to correspond with what average users want and expect. Moreover, the appealing personalities of many UI interfaces will not be complete simply in terms of a single functional appraisal if an individual does not remain up to date to progress on UI technologies and include cutting-edge designs.
The quantative data below summarizes the different key aspects relating to the user experience based on how they are rated from different collected studies.
Table 2:Rating of different factors based on how they impact users’ experience.
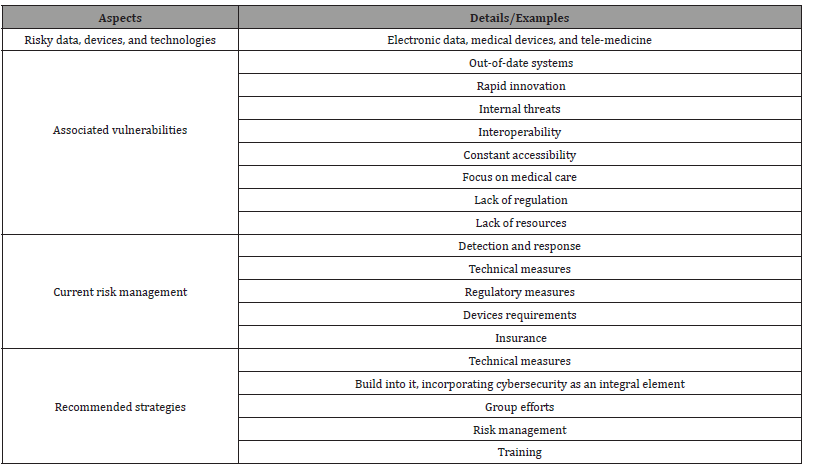
The rating in the table above presents its impact on users’ experience and satisfaction with AI. For instance, accurate and transparent systems showed high ratings across different articles.
Emerging Variances and Trends
The dynamic nature of user experience in this fast-developing knowledge field is shown by the exploration of the most recent trends and variances within the current literature on AI-assisted decisionmaking. The outcomes indicate that user preferences are not constant and change over time. Most importantly, the expectations and demands of users in AI systems are based on cultural shifts, improved technology, and changing societal attitudes (Levy et al., 2023) [25]. Developers and practitioners should be aware that users’ tastes are ever-changing and, therefore, they need to remain agile in their approach to designing AI systems. By recognizing and adjusting to evolving user needs, developers can ensure that artificial intelligence-reliant decision-making software is currently pertinent. This flexibility, however, becomes particularly vital in areas where user satisfaction plays a determining role in ensuring that AI technologies are deployed and accepted.
Advancements in technology become a prominent driver of change in the environments of end users. As AI technologies continue to advance and embrace innovations like natural language processing, explainable AI, and decentralized models, transformational improvements in the ways that users interact with automated systems designed for aiding making are inevitable. It is crucial for developers who want to provide their users with the best user experience possible by integrating new inventions in the continual evolution of artificial intelligence. The influences of environmental and contextual stimuli on user experiences involve another stage of complexity. The literature review helps shed light on the need to factor in cultural peculiarities and situational differences in creating and utilizing AI algorithms (Shahzad et al., 2023; Gill et al., 2022) [26-28].
What might be a positive UX in one cultural setting would change when viewed from the perspective of another. Recognising these differences is essential for promoting cultural inclusivity, aligning with AI-guided decisional processes among varied population segments across different societies and communities. In conclusion, the study recognises trends and differences in the literature and demonstrates that an active approach to AI-supported decision-making should be utilised. Developers, policymakers, and researchers must constantly be watchful of the ever-changing environment regarding user preferences, technological innovations, and cultural factors. In this manner, they can adequately prepare AI systems per the changing needs and desires of an extensive array of end users, which will lead to the full integration and wide adoption of AI as a decision-making tool [29-30].
Discussion
The integrated model of theoretical literature focused on user experiences in AI-enabled decision support provides a delicate view of the complex factors that reproduce patterns across user satisfaction development. This section discusses the implications, limits, and ways in which future research may take on, thus emerging from the synthesized findings.
Implications of AI to Systems Accuracy
The persistent stress on the importance of system accuracy throughout synthesized literature reflects significant implications for AI-empowered decision support systems in terms of design and deployment. Accuracy is, indeed, the crucial thing that sets preferences for user experiences developers and practitioners should highlight the necessary correctness of these systems. Reliability and accuracy of decision outcomes are central characteristics that promote user trust and confidence in the AI platform for making a decision on their part. Thus, investment efforts in improving algorithms and developing accuracy are critical to establishing the proper adoption and development of AI technologies. To address the need for increased accuracy, developers are introduced to include cutting-edge algorithms and accept frequent learning models. The ability to implement stateof- the-art algorithms in systems such as machine learning or deep learning models can improve the decision-making of AI systems. The continuous learning mechanism enables the systems to adjust and refine over time based on novel information and changing tendencies, hence together with accuracy optimization. Besides, considering that advanced technologies are used strategically in this regard, they not only meet users’ expectations of accuracy but also establish AI-driven decision-making systems as reliable tools in different areas.
Nonetheless, the pursuit of accuracy should be met with a need for interpretability as this forms an important challenge to consider when designing AI systems. Even though precise models are necessary, assessing and understanding the logic behind a certain decision is equally vital in relation to huge applications. One of the challenges in realizing a balance between accuracy and interpretability looms large and requires carefulness and further research. To achieve this balance, developers have to pay special attention to trade-offs between these two factors and hence can develop models of artificial intelligence that not only provide high precision but also remain transparent for end users. Resolving this bottleneck requires continued research initiatives toward the integration of AI models that achieve a compromise between accuracy and interpretability. The constant search for accurate and transparent models is key to conserving trust, fulfilling ethical specifications, and the prudent employability of AI initiatives. This balance is one of the most delicate aspects of AI, and as this field continuously develops, future research initiatives should provide innovative solutions to circumvent these limitations, leading to intelligible AI-enabled decision-making tools with high accuracy.
Necessity for Transparency Decision-Making Processes
This focus on the importance of transparent decision-making processes, highlighted throughout the synthesized literature, represents a vital ethical point critical to underlying AI system design and integration. Transparency in decision-making around and by means of AI is the foundation on which it becomes possible to build and sustain user satisfaction, as end users are provided with information about how decisions are made and why some outcomes occur instead of others. Transparency has its ethics when it comes to AI because the mechanisms underlying the workings of some sophisticated machine algorithms are not easily comprehended from an end user’s perspective. Given that AI systems participate increasingly in decision-making, transparency becomes crucial to maintain a trustworthy system and account for ethical standards. Greater understanding of the decision-making rationale and process through which a digital assistant or any other use of AI arrives at particular outcomes enhances human satisfaction levels as well as engagement.
In response to this demand, policy makers and developers must work together to outline explicit regulations that foster transparency in AI systems. Such policies should be intricate and ensure transparency without infringing on proprietary information or undermining competitive advantage of firms. Policymakers have a key position in formulating the regulatory framework motivated by transparency and ethical practices with regard to AI development. In addition, the efforts of the future research should be directed towards defining standardised transparency indicators. The formulation of common standards for measuring transparency in AI systems may also influence a more standardised and accountable world regarding the use of this technology. These standardised measures would not only show the way to developers for the transparent practices but also give users clear guidancelines on the AI-driven decision processes.
An analysis of the efficacy of transparency metrics in various settings represents an important area for future research. Knowing how transparency practices perform in various sectors, environments, and uses can improve the development of rules and lead to the articulation of adjustable and internationally applicable codeboxes. At its core, the quest for transparency in decisionmaking procedures reflects a broader commitment to ensuring responsible AI applications. With the focus on transparency, policymakers and developers has a chance not only to increase user satisfaction but also promote the ethical and accountable AI technologies implementation practice. This aligns with user’s desire to have processes that are clear and straightforward, which means that AI systems will be treated as reliable and responsible tools in different society situations.
User Experiences on Interface Designs
The highlighted role of user interface design, as a catalyst contradict the notion that user satisfaction is critical because it enables one to form a deep understanding of just how important the benefits gleaned from the design regarding AI systems is User interface or UI design is the mediator between the user and complex AI algorithms; this justifies its need to remain focused on continuous enhancement in interface development. Refferetent a good UI design is to do so based on the two cosutemennents mentioned above, aesthetics and inuttiness, while designers are advised to give importance to these elements. Not only does an interface popular with the user in terms of convenience and aesthetics influence overall positive feelings about the AI system but also serves to promoting it. But this is not enough as simplifying the aesthetic aspects of an interface is indeed possible, but it cannot be completely beautified, and this will cause some further difficulties. Their task should be to ensure that it is easy and users will not need a lot of effort since they have little cognitive load needed for fundamental processes.
In order to achieve these goals, designers are provided with the change for iterative User testing and feedback. The fact that the constant improvement of designs through real-users test makes it possible also for designers to understand what pain points, area which can be improved, and preferences are outlined by users. This incremental method of development makes sure that UI design is constantly adapted towards the variety of needs that users have and builds up their requirements in order to satisfy better overall satisfaction. In addition, considering the inclusion of new technologies developed nowadays into user interface design through augmented reality or natural language processing can lead to even further success. The interface can be enriched with augmented reality elements, and the user of it can have a more immersive experience because alongside speech recognition processes also natural language processing will allow for more transparent interaction between the person and AI. The added thoughtful approach of utilising this technology can lead to an allround user interface that has exciting engagement features while being convenient for the users, and at the same time keeping a forward-thinking attitude towards future demands.
Addressing Emerging Variances and Trends in AI
The recent developments and deviations brought about in the literature reveal that user experience with AI systems has a dynamic status. Such a dynamic nature requires proactive reaction from researchers, developers and practitioners to enable AI systems up-to datedness for continued use. It involves constant cognisance about changing preferences, rate of technical changes and the cultural differences among users.
As user preferences are constantly changing, researchers should keep tabs on new developments to stay in tune with users’ needs and desires. Continuous assessments and surveys can be used to catch dynamic patterns in users’ preferences on which basis it would be easier for the system designers as they get information about changing user needs. The knowledge on how users handle technology and design of AI systems based on this consideration maintain sustainable user satisfaction over time, leading to the successful adoption of AI technologies in decision making.
Technological advances represent a dynamic element of the AI setting, and researchers should investigate the repercussions of inevitable technologies in individuals’ encounters. The advent of technologies such as decentralised AI or edge computing brings to board new possibilities and challenges that may determine what an AI system has been designed for, how and its general functionality. Further research may explore in-depth the impact of such budding technologies on users’ perceptual preferences and interactions with Artificial intelligence – assisted decision making. When researchers prepare to consider and resolve the ramifications of such innovations, they can be part of raising AI systems that are cutting-edge while simultaneously focusing on users.
User cultures are very important in the development of user experiences, and here, researchers acknowledge how cultural variance impacts AI adoption. Cultural preferences, values, and norms affect user expectations and their acceptance of AI technologies. Future studies should seek to understand how culture influences user engagement, reflecting users’ understandings into the design of culturally sensitive approaches. By considering and accounting for cultural subtleties, researchers can add to the progression of AI systems composed of users in different cultural foundations.
Study Limitations
Recognition of limitations that underlie synthesis of existing literature is needed for a comprehensive understanding of findings, which have been synthesised. There are several factors which can be attributed to the built in limitations of this method. First, the breadth and depth of the results of synthesis will depend on the quality and number of studies available. Differences in research methods, sample sizes, and study purposes presented within the literature may create bias and limitations. The diversity and rigor of the studies included in the synthesis influence the reliability and generalizability of the findings.
It is essential to remember that the current literature limits the synthesized insights and does not fully reflect all possible user experiences with AI-enhanced decision-making. Second, there is a significant limitation to the possibility of publication bias. Articles with statistically significant results or positive outcomes are more likely to be published than those reporting no effect or negative findings, which may remain unpublished. This bias may create a positive representation of users’ experiences. Addressing the publication bias by incorporating gray literature and unpublished studies into it should be attempted to strengthen the synthesised results. In addition, variations in research methods among the studies provide further limitations.
The comparability and uniformity of the synthesized results can be influenced by changes in data collection techniques, demographics of participants, and analytical strategies. It is important for researchers to maneuver through these methodological divergences carefully, noting that the synthesized insights are bound by limitations imposed by the nature of variety in research approaches across the literature. Lastly, the fast development of AI technologies makes synthesis difficult. AI field is a developing one, with the emergence of new innovations. The compiled findings are confined only by the literature available during the time of a review, and additional insights may accrue after this summation process. Researchers and practitioners should remain aware of the progress in AI technologies as well as continue observing users’ experiences that were influenced by AI-assisted decision-making.
Conclusion and Recommendation
Conclusion
The underlying themes that repeatedly foreground the key importance of system accuracy, the ethical mandate for transparent decision-making processes, and user interface design reveal integrative dynamics among technological, ethical and user-centered issues associated with defining the formation of a particular course of users’ experiences. The study results confirm the positive perception of users about AI-supported decision support, which is characterized by high accuracy scores. Moreover, the issue of transparency, process as a measure for increasing user trust and confidence, is ethical in conformance to moral principles, whereas design issues, which essentially refer to user interface designs, act as mediums that promote complete satisfaction among users. The integration further captures the facets of user experiences that are constantly evolving under the aegis of emerging trends, technological innovations, and cultural differences. Nevertheless, it is vital to note that there are significant limitations associated with meta-literature synthesis, such as biased findings and the factors affecting different methodologies of meta-research concerning the dynamic landscape in terms of AI technologies.
Recommendations
Based on the study conducted, different recommendations can be presented.
Prioritize Accuracy and Transparency: AI ought to be increasingly precise alongside openness in decision production. They also need to give focal points to the designers. Shortening, advanced technology integration, and ethical standards guarantee good passersby usage.
Invest in User-Centric Design: The designers must constantly adjust the user interface designs as aesthetics, intuitive operation, and easy navigation should be the focus of amending these factors. It is also evident that the iterative application of user testing and feedback use mechanisms makes it possible to adapt UI designs to different needs. When going beyond the traditional use of three-dimensional technologies in order to integrate emerging technologies that include augmented reality and natural language processing, the user interfaces will undoubtedly be approached on a more advanced level.
Stay Vigilant to Emerging Trends: Policymakers, developers and researchers should proactively resolve new trends and variations while anticipating unknown technologies. Future research should focus on understanding the impact of new technologies, such as decentralised AI and edge computing, which are unfolding on the quality of user experiences.
Mitigate Limitations Through Rigorous Research: The process itself is part of the limitation researchers should also be aware of and try to limit. Reducing publication bias, considering different methodological approaches, and looking at changing AI situations may assist us in setting our signals on the right course.
Encourage Cross-Disciplinary Collaboration: As it involves different fields, collaboration among the developers of artificial intelligence systems, policymakers, and researchers is necessary for AI-assisted decision-making. The interdisciplinary initiatives may reduce AI technologies to responsible and user-user attribution by development, implementation, and control.
In a recommendation for future research, having thus synthesized the findings, there is a need to look into ethical components associated with AI-based decision support systems, especially in safeguarding them from bias and discrimination, which merits separate analyses. By comparing longitudinal data, it is possible to see how user experience across time changes. And yet, further research could examine how contextual differences, like organizational culture or social norms, influence user satisfaction.
Acknowledgments
I am grateful to Shaqra University for their constant support throughout the research process and the development of this publication. The university’s resources and academic environment played a vital role in shaping the results of this work. I am thankful for the opportunity to make a significant contribution to the academic community and acknowledge Shaqra University’s crucial role in facilitating and empowering this endeavor. The author would like to thank the Deanship of Scientific Research at Shaqra University for supporting this work.
Conflict of Interest
No conflict of interest.
References
- Shehzad MK, Rose L, Butt MM, Kovács IZ, Assaad M, et al. (2022) Artificial intelligence for 6G networks: Technology advancement and standardisation. IEEE Vehicular Technology Magazine 17(3): 16-25.
- Duan Y, Edwards JS, Dwivedi YK (2019) Artificial intelligence for decision making in the era of Big Data–evolution, challenges and research agenda. International journal of information management 48: 63-71.
- Delipetrev B, Tsinaraki C, Kostic U (2020) Historical evolution of artificial intelligence.
- Ouyang F, Jiao P (2021) Artificial intelligence in education: The three paradigms. Computers and Education: Artificial Intelligence 2: 100020.
- Cronholm S, Göbel H (2022) Design Principles for Human-Centered Artificial Intelligence. In European Conference on Information Systems (ECIS), Timisoara, Romania pp. 18-24.
- Cirqueira D, Helfert M, Bezbradica M (2021) Towards design principles for user-centric explainable AI in fraud detection. In International Conference on Human-Computer Interaction pp. 21-40.
- Varghese M, Raj S, Venkatesh V (2022) Influence of AI in human lives.
- Gabriel I (2020) Artificial intelligence, values, and alignment. Minds and machines 30(3): 411-437.
- Haleem A, Javaid M, Qadri MA, Singh RP, Suman R (2022) Artificial intelligence (AI) applications for marketing: A literature-based study. International Journal of Intelligent Networks.
- Semerádová T, Weinlich P, Semerádová T, Weinlich P (2020) Factors influencing user experience. Website Quality and Shopping Behavior: Quantitative and Qualitative Evidence pp. 29-62.
- Deng S, Fang Y, Liu Y, Li H (2015) Understanding the Factors Influencing User Experience of Social Question and Answer Services. Information Research: An International Electronic Journal 20(4): n4.
- Nagaraj SK, Bryant A (2016) Factors in building transparent, usable and comprehensive user privacy policy system. In 11th International Conference on Cyber Warfare and Security: ICCWS2016. Academic Conferences and publishing limited pp. 253.
- Pratama MAT, Cahyadi AT (2020) Effect of user interface and user experience on application sales. In IOP Conference Series: Materials Science and Engineering 879(1): 012133.
- Zhang Y, Liao QV, Bellamy RK (2020) Effect of confidence and explanation on accuracy and trust calibration in AI-assisted decision making. In Proceedings of the 2020 conference on fairness, accountability, and transparency, 295-305.
- Tajmir SH, Lee H, Shailam R, Gale HI, Nguyen JC, et al. (2019) Artificial intelligence-assisted interpretation of bone age radiographs improves accuracy and decreases variability. Skeletal radiology 48(2): 275-283.
- Lee D, Yoon SN (2021) Application of artificial intelligence-based technologies in the healthcare industry: Opportunities and challenges. International Journal of Environmental Research and Public Health 18(1): 271.
- Balasubramaniam N, Kauppinen M, Hiekkanen K, Kujala S (2022) Transparency and explainability of AI systems: ethical guidelines in practice. In International Working Conference on Requirements Engineering: Foundation for Software Quality, (3-18).
- Larsson S, Heintz F (2020) Transparency in artificial intelligence. Internet Policy Review 9(2).
- Andrada G, Clowes RW, Smart PR (2023) Varieties of transparency: Exploring agency within AI systems. AI & society 38(4): 1321-1331.
- Felzmann H, Villaronga EF, Lutz C, Tamò-Larrieux A (2019) Transparency you can trust: Transparency requirements for artificial intelligence between legal norms and contextual concerns. Big Data & Society 6(1).
- Han X, Huang D, Eun-Lee S, Hoon-Yang J (2023) Artificial Intelligence-Oriented User Interface Design and Human Behavior Recognition based on Human–Computer Nature Interaction. International Journal of Humanoid Robotics 20(06): 2250020.
- Stige Å, Zamani ED, Mikalef P, Zhu Y (2023) Artificial intelligence (AI) for user experience (UX) design: a systematic literature review and future research agenda. Information Technology & People.
- Bader V, Kaiser S (2019) Algorithmic decision-making? The user interface and its role for human involvement in decisions supported by artificial intelligence. Organization 26(5): 655-672.
- Dudley JJ, Kristensson PO (2018) A review of user interface design for interactive machine learning. ACM Transactions on Interactive Intelligent Systems (TiiS) 8(2): 1-37.
- Levy J, Lu Y, Montivero M, Ramwala O, McFadden J, et al. (2023) Artificial Intelligence, Bioinformatics, and Pathology: Emerging Trends Part I-an Introduction to Machine Learning Technologies. Advances in Molecular Pathology.
- Sharma P, Kaur N (2022) Emerging trends in artificial intelligence-based banking Services: application of UTAUT model towards individual acceptance NeuroQuantology 20(12): 2855.
- Shahzad U, Asl MG, Panait M, Sarker T, Apostu SA (2023) Emerging interaction of artificial intelligence with basic materials and oil & gas companies: A comparative look at the Islamic vs. conventional markets. Resources Policy 80: 103197.
- Gill SS, Xu M, Ottaviani C, Patros P, Bahsoon R, et al. (2022) AI for next generation computing: Emerging trends and future directions. Internet of Things 19: 100514.
- Nguyen A, Meyer NNJ, Dawe J, Shi M, Androutsos W, et al. (2024) AI improves accuracy, agreement and efficiency of pathologists for Ki67 assessments in breast cancer. Scientific Reports 14(1): 1283.
- Conant EF, Toledano AY, Periaswamy S, Fotin SV, Go J, et al. (2019) Improving accuracy and efficiency with concurrent use of artificial intelligence for digital breast tomosynthesis. Radiology: Artificial Intelligence 1(4): e180096.
-
Marran Aldossari*. Enhancing User Experiences in Ai-Driven Decision-Making. On Journ of Robotics & Autom. 3(1): 2024. OJRAT.MS.ID.000552.
AI; Decision-making; AI-aided decision-making; User experiences; HCI
-
This work is licensed under a Creative Commons Attribution-NonCommercial 4.0 International License.