Research Article
The Adsorption Behavior of Copper Clusters on SiO2 and TiO2 Surfaces: A Computational Study
Shenna M Shearin1*, Michael J Taft2, Divi Venkateswarlu3 and Debasish Kuila3
1Department of Chemistry, Bennett College, USA
2Department of Chemical, Biological and Bioengineering, North Carolina Agricultural and Technical State University, USA
3Department of Chemistry, North Carolina Agricultural and Technical State University,
Shenna M Shearin, Department of Chemistry, Bennett College, USA
Received Date: March 01, 2020; Published Date: March 18, 2020
Abstract
In this study the various adsorption modes of binding of copper on SiO2 and TiO2 surfaces were investigated by advanced computational techniques. The central objective of this study was to develop a working model of metal-oxide surface-mediated copper clusters, since such catalytic matrixes have a wide-range of applications in the Methanol Steam Reform process. The structural models of the copper clusters ranging from n=2 to n=20 were created using the Birmingham Cluster genetic algorithm (BCGA) coupled with the Gupta potential based on the physiochemical parameters published by Cleri and Rosato [1]. Optimization of the copper clusters was performed using Density Functional Theory (DFT) with PBE XC functional of Pbe0 and LANL2DZ basis set of NWChem package. Adsorption binding of the Cu clusters on SiO2 and TiO2 surfaces were performed using periodic Density Functional Theory (DFT) and PBE XC functional of the Quantum Expresso package. to investigate the binding free energy, the most optimal mode of binding, and the key adsorption interactions of Cu atoms on SiO2 and TiO2 surfaces.
Keywords: Adsorption; Copper; Metal-oxide; Density functional theory; Methanol steam reform
Introduction
Depletion of petroleum based fossils fuels provoked the pursuit for generating alternative safe and environmentally clean fuels over the past decade. Hydrogen gas (H2) has long been regarded as a promising alternative to fossil fuels. For instance, fuel cells polymer electrolyte membrane fuel cells (PEMFC) are one of the primary sources as high efficiency energy converting devices. In such processes, producing safe storage of explosive hydrogen gas and circumventing the opportunities of leakage remains a challenging task. Compared to gaseous hydrogen gas storage, on-board hydrogen production by reforming liquid hydrogen carriers will be more promising for future commercialization. Steam reforming of alcoholic sources such as methanol, ethanol or glycerol have been investigated comprehensively for their potential to be converted to hydrogen. Methanol steam reforming (MSR) is considered one of the most favorable chemical processes for on-the-fly hydrogen production for several reasons [2,3]. (i) Methanol is in liquid-state at ambient condition; (ii) it has high H-to-C atom ratio; (iii) requirement of relatively low temperatures (200-400 °C) for activation of methanol and (iv) methanol is sulfur-free and can be easily produced from biomass. Various catalysts have been developed for MSR reactions.
In particular, copper (Cu) based catalysts have demonstrated the ability to produce gas with high hydrogen (H2) concentration and high selectivity for carbon dioxide (CO2) [2,3]. Metal oxide substrates exhibit a strong effect on the catalytic efficiency. Copper supported catalysts exhibited a higher selectivity for hydrogen and a lower selectivity for CO2 on silica (SiO2) than on a titania (TiO2) at 250 °C [2,3]. SiO2 has been used as a structural support matrix as it stabilizes copper particles and enhances the activity in Methanol Steam Reforming (MSR) reactions [2,3]. Additionally, experimen- tal studies reveal Cu-SiO2 catalysts exhibits a higher selectivity for hydrogen and a lower selectivity for carbon monoxide (CO) than that of Cu in the framework of TiO2 [2,3]. Understanding the nature of these reactions on an atomic level by experimental techniques is difficult due to the electronic effect of metal-oxide interactions. Furthermore, quantum mechanical methods are powerful tools that contribute useful information about the microscopic aspects of metal-oxide interactions [4-19]. In a study by Pacchioni and coworkers, Density Functional Theory (DFT) calculations were used to predict the interaction of isolated Cu atoms and small Cun clusters (2 ≥ n ≤ 5) on metal-oxide support which resulted due to the electrostatic interactions between the Cu metal and the support of a two-coordinated oxygen of the SiO2 [4]. It was also demonstrated that isolated Cu atoms deposited on TiO2 shows a preference to transfer their valence electron to the bridging oxygen sites of TiO2 with formation of a strong bond while the interaction with the Ti sites are weak [4].
The adsorption behavior and binding interactions of Cu clusters on SiO2 and TiO2 supports are difficult to elucidate via experimental methods. This study is useful for gaining an in-depth understanding of the adsorption behavior of small Cun clusters on SiO2 and TiO2 supports and the thermodynamic properties of cluster- oxide interactions for producing optimal yields of hydrogen in the MSR process.
Methods and Design
Construction of Cun clusters
The Birmingham Cluster Genetic Algorithm (BCGA) combined with Gupta potential [20] and DFT using XC functional of Pbe0 and LANL2DZ basis set of NWChem package were employed to construct and optimize the Cu clusters [22,20,5,6]. The inter-atomic interactions provided by the Gupta potential, a many-body potential based on the Friedel’s tight-binding model [20], was used to account for the repulsive and attractive terms. In this model, the configurational energy of a cluster is written as the summation of all atoms of attractive and repulsive energy components in equation (1);

Where the Born-Mayer pair is expressed as the repulsive term Vr (i) as shown in equation (2);

The many-body attractive term Vm (i) is expressed as a and b represent the atomic species of atoms i and j, respectively, as shown in equation 3.

A, ξ, p and q are potential parameters that are usually fitted to experimental properties of bulk metals and alloys, such as cohesive energy, lattice parameters, and independent elastic constants for the reference crustal structure at 0 K; r0 denotes the bond length of the bulk material. This bond length is taken as the average between the bulk values of the pure metals in agreement with Vegard’s law [7,8]; and rij is the distance between atoms i and j. Values of the Gupta potential parameters describing Cu-Cu are extracted from the work of Cleri and Rosato [1]. Table 1 shows the parameter values used in this work.
Table 1:Gupta potential parameters for Cu metal.

Construction of SiO2 and TiO2 models
The first and most crucial step is to find the best binding mode which generates the most stable complex. All predicted models start with this step. Initial coordinates for the structural models of silica (SiO2) and titania (TiO2) were derived from Zeolite database (www. http://europe.iza-structure.org) [21]. Periodic DFT calculations were performed using the Quantum Expresso package, employing a basis set of plane waves, ultrasoft pseudopotentials and the Perdew-Burke-Ernzerhof (PBE) exchange-correlation (XC) functional [22]. The energy and density cutoffs were set as 40Ry and 400Ry, respectively.
The Brillouin zone was sampled at the gamma point. We used the Gaussian smearing method with a finite temperature width of 0.03 eV to improve convergence near the Fermi level. Figure 1 shows the dimensions of the unit cell for both surfaces. For SiO2 models, a 2x2 rectangular unit cell with dimensions of 13.80Å and 12.92Å was used in the x and y directions, respectively. The three-dimensional parameters of the unit cell for silicon–oxygen tetrahedron (SiO4, id: EDI), a three four-membered SiO2 ring include dimensions represented as a=b=6.90Å, and c=6.46Å [3]. For TiO2 models, a 2x2 rectangular unit cell with dimensions of 5.96Å and 9.26Å was used in the x and y directions, respectively. The unit cell parameters for TiO2 are a=b=4.64Å and c=2.96Å [21]. A vacuum space of 15Å was introduced to curtail replicated cell interactions.
Construction of Cu-oxide complexes
The copper clusters were arranged every 60° while separated with about 4-5Å of vacuum space between the support and cluster. The same periodic DFT potential and PBE XC functional was employed of the Quantum Expresso package [22]. Three-dimensional molecular renderings were generated using Avogadro, Chimera, and Xcrysden visualization programs. Figures 2 and 3 shows the possible orientations of Cu-7 clusters on SiO2 and TiO2 before and after optimization, respectively.


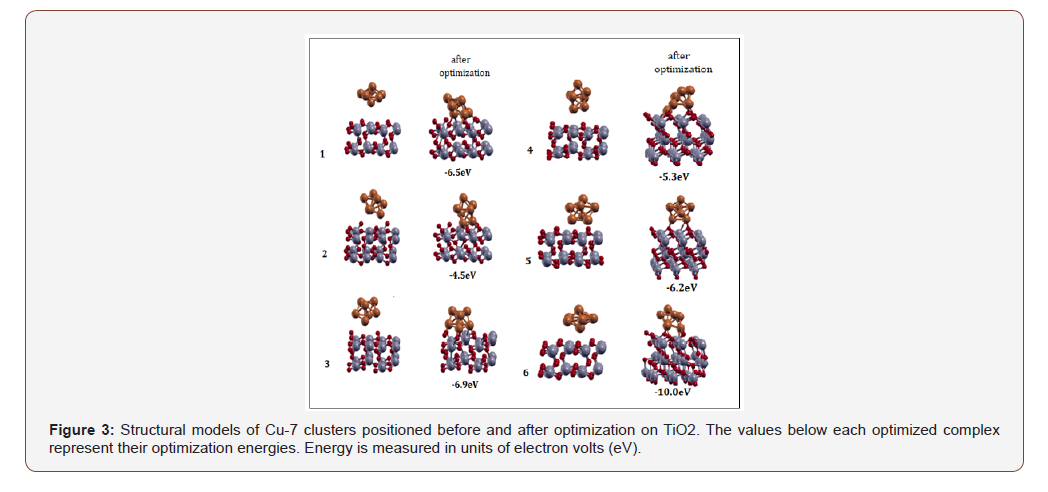
Results and Discussion
Rational for the initial selection of Cu-7 atom clusters
Many complementary computational studies have attempted to validate and elucidate the preferred configurations of Cun clusters based on mass spectra cluster analysis, ionization potentials, and electron affinities observations [14]. Calculations performed on pure copper and gold clusters as well as bimetallic Cu-Au clusters have been performed. Based on analysis of the stability function, copper clusters showed significant peaks at n= 7, 13, 19, 23, and 55 while for gold clusters there were large peaks at 7 and 13 (due to the pentagonal bipyramidal geometry) and no significant peaks were observed at n=19 or 55 as these clusters take on an amorphous structure. Instead more advanced theoretical methods such DFT have been performed to predict the structural evolution of smaller metal clusters in the size range of n= 2 to n=30. Some DFT based calculations have predicted that copper clusters in the size range of n=2 to n=6 adopt a planar geometry [2,10,21] whereas a structural transition from planar to pentagonal bipyramidal geometry occurs at n=7. This pentagonal bipyramidal shape is observed as the precursor for double-layered structures as atoms grow up to n =16 [18,19]. Therefore, a benchmark optimization study with Cun clusters ranging from n=2 to n=20 was conducted to determine the best starting point in the initial selection for the most stable, lowest energy geometric copper isomer.
Optimization of Cu clusters
DFT calculations using Pbe0 and LANL2DZ basis set of NWChem package were performed to optimize the copper clusters ranging from n=2 to n=20. Figure 4 illustrates that the Cu-7 cluster is geometrically and thermodynamically the most stable with an energy value of -5.7 eV. Based on the energy diagram, as the cluster size increases, the energy decreases. Cu-7 is identified as having the lowest-energy structure. This is consistent with several theoretical studies [4,8-19]. A description of the possible modes of binding for Cu7 is shown in Figure 5. It appears that Cu-7 can adopt two possible configurations, horizontal (left) and vertical (right). The structure of Cu-7 adopts a pentagonal bipyramidal geometry. The binding modes and corresponding energies for each Cu-oxide model were further investigated.


The adsorption energy (Es) was calculated from the minimized ground state energies of all component structures as shown in equation 4:

Ecomplex is the Cu cluster interaction with the metal-oxide surface; Esubstrate and Ecluster represent the pure metal-oxide surface and pure Cu cluster, respectively. Table 2 shows that Cu- SiO2complexes appears to be twice as energetically stable than Cu- TiO2.
Table 2:Adsorption energies of Cu-7 clusters in the framework of SiO2 and TiO2.

Analysis of adsorption interactions in Cu based SiO2 and TiO2 complexes
Figure 6 illustrates the intermolecular interactions of Cu-7 cluster on SiO2 and TiO2. It appears that Cu-7 interacts strongly with O and weakly with Si and Ti atoms on both surfaces. Ti-Cu has a covalent bond length of approximately 2.66 Å, longer than Cu-O, which extends to only 1.85 Å. There appears to be more Cu-O interactions in the framework of SiO2 which makes for a stronger network of intermolecular forces. The weak interactions appear to help retain the symmetry of Cu-7 cluster in the framework of TiO2. On the contrary, Cu-7 cluster symmetry is greatly reduced on SiO2 surface perhaps due more electrostatic Cu-O interactions. It is deemed that the surface area and pore size of SiO2 may also contribute to a more distorted shape of Cu-7 cluster.
Validation of Cu-oxide complexes based on experimental observations
The significant structural changes induced by SiO2 on Cu7 cluster explains the large energy gap between the two systems. Based on experimental results provided by Dr. Kuila and coworkers (Table 3), Cu- SiO2 catalysts are less selective for CO than Cu- TiO2 catalysts. Based on these observations, the greater number of Cu-O interactions, larger pore size, and larger surface area could contribute to lower selectivity for the adsorption sites of CO on SiO2 versus Cu- TiO2 catalysts. To explore the energy profile of the reaction pathway for Cu in the framework of both surfaces is beyond the scope of this study.
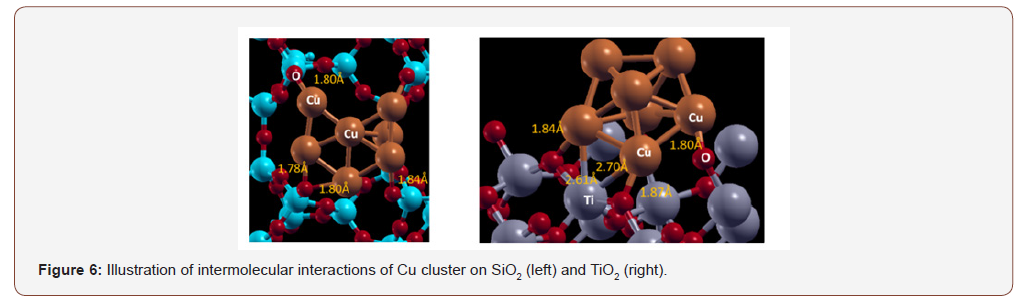
Table 3:Experimental data of the Methanol Steam Reforming pathways of Copper on SiO2 and TiO2.
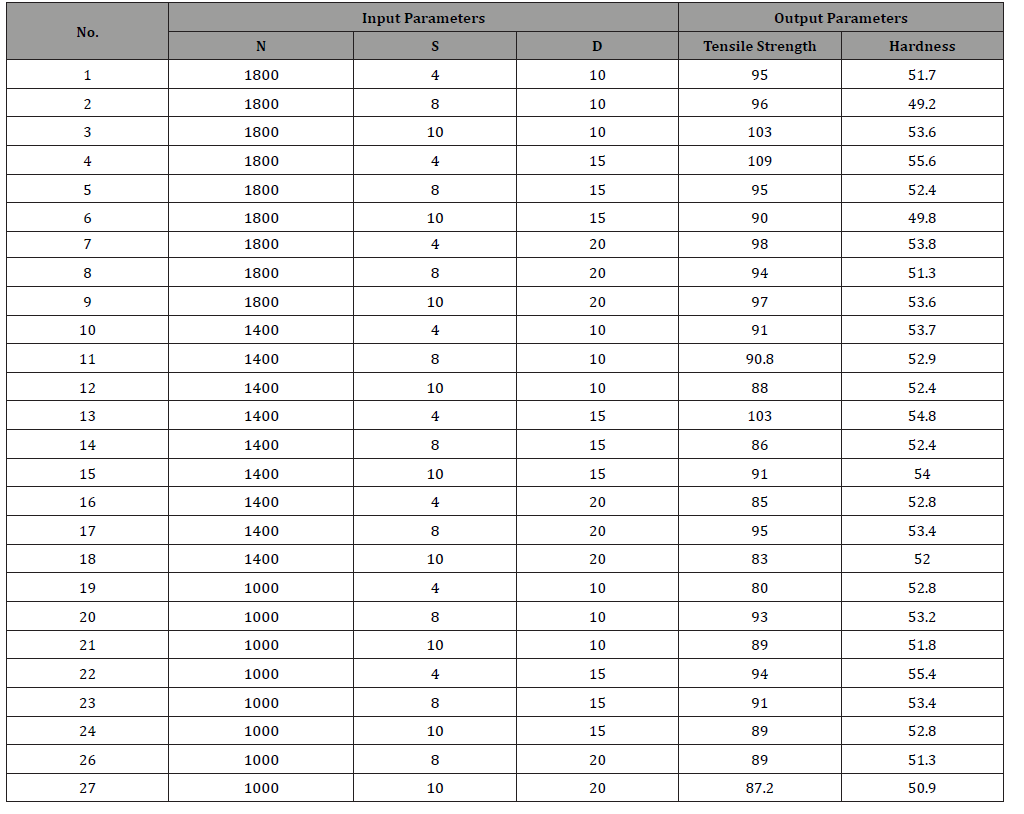
Conclusion
The working models provide insight regarding the structural profile of Cu clusters on SiO2 and TiO2. Our data shows the atomic composition of Cu in the framework of SiO2 and TiO2 is governed by intermolecular forces which play key roles in the thermo-stability of the Cu-oxide clusters [2,3]. BCGA and DFT methods were used to predict and compare the most stable geometry and binding affinity for Cu7 cluster while on the surface of SiO2 and TiO2. Our results predict that the binding of Cu7 cluster horizontally on the SiO2 surface is the most favorable. It was observed that copper clusters on SiO2 undergo a significant structural change from pentagonal bipyramidal to a planar geometry while the cluster geometry on TiO2 is retained. We conclude that Cu-SiO2 catalysts exhibit more electrostatic interactions of Cu-O bonds as well as elongation of Cu-Cu bonds, and wider surface area contribute to a more stable metal complex which is in strong correlation with experimental and theoretical data [2,3,23]. Experimental results show that Cu- SiO2 catalysts exhibits a higher selectivity for hydrogen and a lower selectivity for carbon monoxide than Cu clusters in the framework of TiO2 perhaps due to more Cu-O covalent interactions which are known to enhance structural stability [23-32].
Acknowledgement
The author gives upmost thanks to Dr. Roy L Johnston for providing the Birmingham Genetic Cluster Algorithm and Dr. Richard Abrokwah for his expertise in Steam Reforming Pathways.
Conflict of Interest
No conflict of interest.
References
- Cleri F, Rosato VV (1993) Tight-binding potentials for transition metals and alloys. Phys Rev B Condens Matter 48(1): 22-23.
- Takahashi K, H Kobayashi, N Takezawa (1985) on the difference in reaction pathways of steam reforming of methanol over copper-silica and platinum-silica catalysts. Chemistry Letters 14(6): 759-762.
- Takezawa N, N Iwasa (1997) Steam reforming and dehydrogenation of methanol: Difference in the catalytic functions of copper and group VIII metals. Catalysis Today 36(1): 45-56.
- Pacchioni G, Lopez N, Illas F (1999) Cu atoms and clusters on regular and defect sites of the SiO2 Electronic structure and properties from first principle calculations. Faraday Discuss 114: 209-222.
- Roy L Johnston (2003) Evolving better nanoparticles: Genetic algorithms for optimising cluster geometries. Dalton Trans 22: 4193-4207.
- M Valiev, EJ Bylaska, N Govind, K Kowalski, TP Straatsma, et al. (2010) NWChem: a comprehensive and scalable open-source solution for large scale molecular simulations. Comput Phys Commun 181(9): 1477-1489.
- Rama Krishna K, Vijaya Kumar K, Ravindernathgupta C, Dachepalli Ravinder (1921) Magnetic Properties of Ni-Zn Ferrites by Citrate Gel Method. L Vegard Z Phys 2(3): 149-154.
- (1928) L Vegard: Z Cryst 67: 239.
- Shin K, Kim DH, Yeo SC, Lee HM (2012) Structural stability of AgCu bimetallic nanoparticles and their application as a catalyst: A DFT study. Catal. Today 185(1): 94-98.
- Guvelioglu GH, Ma P, He X, Forrey RC, Cheng H (2005) Evolution of small copper clusters and dissociative chemisorption of hydrogen. Phys Rev Lett 94(2): 026103.
- Itoh M, Kumar V, Adschiri T, Kawazoe Y (2009) Comprehensive study of sodium, copper, and silver clusters over a wide range of sizes 2 <= N <= 75. J Chem Phys 131(17): 174510.
- Lecoultre S, Rydlo A, Félix C, Buttet J, Gilb S, et al. (2011) Optical absorption of small copper clusters in neon: Cu-n, (n=1-9). J Chem Phys. 134(7): 074303.
- Nunez S, Johnston RL (2010) Structures and Chemical Ordering of Small Cu-Ag Clusters. Journal of Physical Chemistry C 114(31): 13255-13266.
- Winter BJ, EK Parks, SJ Riley (1991) Copper Clusters - the Interplay between Electronic and Geometrical Structure. J Chem Phys 94(12): 8618-8621.
- Winter BJ, EK Parks, SJ Riley (1991) Chemical-Identification of Icosahedral Structure for Cobalt and Nickel Clusters. Zeitschrift Fur Physik D Atoms Molecules and Clusters 19(1-4): 375-380.
- Ziashahabi A, T Ghodselahi, MH Saani (2013) Localized Surface Plasmon Resonance properties of copper nano-clusters: A theoretical study of size dependence. Journal of Physics and Chemistry of Solids 74(7): 929-933.
- Weissker, HC, C Mottet (2011) Optical properties of pure and core-shell noble-metal nanoclusters from TDDFT: The influence of the atomic structure. Physical Review B 84(16): 165443.
- Reinhard D, BD Hall, P Berthoud, S Valkealahti, R Monot (1997) Size-dependent icosahedral-to-fcc structure change confirmed in unsupported nanometer-sized copper clusters. Physical Review Letters 79(8): 1459-1462.
- Knickelbein MB (1992) Electronic Shell Structure in the Ionization-Potentials of Copper Clusters. Chemical Physics Letters 192(1): 129-134.
- Gupta R (1981) Lattice relaxation at a metal surface. Phys Rev B 23: 6265-6270.
- Ch Baerlocher, LB McCusker (2017) Database of Zeolite Structures.
- Giannozzi P, Baroni S, Bonini N, Calandra M, Car R, et al. (2009) QUANTUM ESPRESSO: a modular and open-source software project for quantum simulations of materials. Journal of Physics-Condensed Matter 21(39): 395502.
- Debasish Kuila (2015) Methanol Steam Reforming pathways of Copper on SiO2 and TiO2. American Chemical Society, USA.
- Grigoryan VG, D Alamanova, M Springborg (2006) Structure and energetics of Cu-N clusters with (2 ≤ N ≤ 150): An embedded-atom-method study. Physical Review B 73(11).
- Darby S, Thomas V Mortimer-Jones, Roy L Johnston, Christopher Roberts (2002) Theoretical study of Cu-Au nanoalloy clusters using a genetic algorithm. Journal of Chemical Physics 116(4): 1536-1550.
- Erkoc S, R Shaltaf (1999) Monte Carlo computer simulation of copper clusters. Physical Review A 60(4): 3053-3057.
- Yang M, Jackson KA, Koehler C, Frauenheim T, Jellinek J (2006) Structure and shape variations in intermediate-size copper clusters. Journal of Chemical Physics 124(2): 024308.
- Jiang M, Zeng Q, Zhang T, Yang M, Jackson KA (2012) Icosahedral to double-icosahedral shape transition of copper clusters. Journal of Chemical Physics 136(10): 104501.
- Barcaro G, Sementa L, Negreiros E, Fortunelli A (2013) Computational and Theoretical Chemistry 1021: 222-228.
- Giannozzi P, Baroni S, Bonini N, Calandra M, Car R, et al. (2009) QUANTUM ESPRESSO: a modular and open-source software project for quantum simulations of materials. J Phys Condens Matter 21(39): 395502.
- Baletto F, Ferrando R (2005) Structural properties of nanoclusters: Energetic, thermodynamic, and kinetic effects. Rev Mod Phys 77: 371-423.
- PA Deshpande (2016) Computational investigation of Cu7 as a model biomimetic CO2 capture catalyst. Chemical Engineering Science 145: 294-298.
-
Shenna M Shearin, Michael J Taft, Divi Venkateswarlu, Debasish Kuila. The Adsorption Behavior of Copper Clusters on SiO2 and TiO2 Surfaces: A Computational Study. Mod Concept Material Sci. 2(5): 2020. MCMS.MS.ID.000550.
-
DLVO, Coal, Rheology, Particle size, Energy barrier, Interactions, Surface area, pH, Viscosity, Porosity
-
This work is licensed under a Creative Commons Attribution-NonCommercial 4.0 International License.