Research Article
Perceived Risk in Cloud Computing Technology Adoption in Small and Medium-Sized Accounting Firms
Jacqueline T Jamsheed*
Department of Accounting, Elms College, USA
Zora P deRham
Corresponding AuthorJacqueline T Jamsheed, Department of Accounting, Elms College, USA.
Received Date: September 05, 2023; Published Date: September 20, 2023
Abstract
This study uses data from an online survey of 116 small-and medium-sized (SME) accounting firm leaders to research perceptions of cloud computing technology adoption to meet staff demands for a remote work environment and customer requirements for digital service offerings post-COVID-19 pandemic. The perceived risk impacting cloud adoption decisions were studied using the theory of planned behavior (TPB) and technology acceptance model (TAM) as the scaffolding for the research model. The analysis was conducted using partial least squares (PLS). The findings indicate that leaders have significantly favorable views towards the adoption of cloud technology. Moreover, the research finds, through the unsupported hypotheses of risk perception, that firm leadership does not find the risk of cloud adoption a barrier to their consideration of technology. The study illustrates how SME accounting firm leadership views the adoption of cloud-enabled technologies to meet their evolving business needs, while illuminating attitudinal and intentional factors impacting technology adoption. Based on the literature review and to the best of the authors’ knowledge, this study is the only published research focusing on SME accounting firm leadership perceptions surrounding the risks associated with cloud technology adoption resulting from the post-pandemic business changes.
Keywords: Cloud technology; Small and medium-sized accounting firms; Small and medium-sized businesses (SMEs); Risk, Risk Perception; Technology Acceptance Model; Theory of Planned Behavior
Introduction
One impact of the post-COVID-19 pandemic business environment is the desire of employees, customers, and supply chains to reimagine the concept of work and the interactions that their work entails [1-4]. This reimagined landscape includes consideration of cloud enabled technologies; Large enterprises estimate that approximately 60% of their environments will be migrating in the cloud by 2025, generating $3 trillion in EBITDA value by 2030 [5]. Small and medium-sized enterprises (SMEs) must also reimagine work and are faced with an unprecedented push towards the adoption of cloud-supported technologies in support of a workforce and client base wanting to maintain many practices and processes surrounding their remote interactions, as adopted at the height of the pandemic [6-10]. In the current environment, a key to sustainable growth and continued relevance for many SMEs is the consideration of cloud enabled technologies [11]. Cloud technologies are also affordable and easy to use, making their adoption attractive to SMEs [12].
This research attempts to understand how small and mediumsized regional and local accounting firm decision-makers perceive the risks associated with cloud computing technology adoption in the context of theory of planned behavior (TPB) and technology acceptance model (TAM). Evidence was gathered via an online survey of 116 leaders representing accounting firms from various geographical locations. The analysis was conducted using partial least squares (PLS). This study aims to enrich the business and academic community’s knowledge of the enticements and barriers to technology adoption in small-and medium-sized accounting firms responding to a changing work landscape.
The results indicate that SME accounting firm leadership does not find the risk of cloud adoption a barrier to their consideration of cloud-enabled technologies due to the potential increase in job performance and productivity. The survey results also indicated a positive attitude towards the use of cloud technology owing to its perceived ease of use and usefulness. The leadership in our study also felt that they had sufficient knowledge, understanding, and support to adopt cloud technology if they decided to do so. This study on the perceived risks of cloud technology adoption was based leadership’s intention to adopt, perceptions of ease of use and overall usefulness grounded in the theory of planned behavior and the technology acceptance model.
This study makes many academic and practical contributions to literature. Based on the literature review and to the best of the authors’ knowledge, this study is the only published research focusing on SME accounting firm leadership perceptions surrounding the risks associated with cloud technology adoption as a result of the business changes brought about by the postpandemic world order. In addition, this study adds to the literature on the factors affecting smaller accounting firms’ technology adoption. Theoretically, this study builds on past research surrounding technology risk perception, the predictive power of technology acceptance and theory of planned behavior models by introducing a new setting of accounting SME leadership views on cloud technology adoption. Practically speaking, this study provides insights into the thinking and considerations of SME accounting firm leadership when making technology acquisition decisions.
Background and Theory
Cloud adoption advantages and disadvantages
Cloud computing technology, as defined in this research, refers to general internet-based computing services rather than to a particular type of characteristic (e.g., on demand, resource pooled), model (e.g., public, private, hybrid or community), or category (e.g., SaaS, PaaS, IaaS) [13,14]. Cloud computing embodies the convergence of two major trends in information technology: efficiency and business agility, [15] which can present advantages and disadvantages to businesses that employ this technology. Advantages include cost savings/efficiency, continuous availability, reliability, agility, scalability/flexibility/elasticity, quick deployment with ease of integration, increased storage capacity, outsourced maintenance, minimized new software licensing, innovation, simultaneous user access, and environmentally friendly solutions [16-25]. Disadvantages include lack of user control due to the outsourced nature of the product, network dependency due to 100% internet usage, risk/exposure to cyber threats, concerns over third-party access to systems, and challenges linked to data and system migration [26,17,18,27,22,23].
Cloud adoption risk considerations and compensating controls
Cloud computing has attracted many as it facilitates collaborative remote work, virtual data sharing and anywhere data access. In reviewing the literature, the barriers to cloud adoption in the form of perceived risks, especially for SMEs, are high with these business continuity threats requiring consideration and attention. Some of the most cited perceived risks influencing a business’s decision on cloud adoption are: (1) network reliability/connectivity, (2) control over stored data, (3) security of stored data- where is it located and who has access to it, (4) overall cost- transition to the cloud and storage, (5) service provider ability, trustworthiness and support, (6) data back-up frequency, completeness and deletion, and (7) overall technical savvy and understanding of internal staff [28-34]. The risks surrounding compatibility with current systems, data leakage/loss during transfers, infrastructure support, and network breaks are additional systematic risks requiring attention once a move to that cloud has occurred [35-37]. An overarching factor influencing user trust placed in cloud service providers is the understandability and transparency in which policies surrounding the securing of user data and the management of data access are outlined [38].
Within the accounting community the risks associated with information system adoption are outlined citing five trust service categories (security, availably, processing integrity, confidentiality and privacy) that must be considered and addressed in each engagement (AICPA, 2022). Building on the work of the AICPA under the framework of cloud technology utilization, trust within the areas of authorization, authentication, and access management was identified as a key consumer concern [39]. Trust is seen as a crucial factor in the adoption of any technology influencing the perceived risks associated with technology acceptance and the speed at which adoption occurs [40-42]. Initial trust can also be established based on the service level agreements (SLA) provided by the cloud service provider and how these contractual agreements provide required assurances [43].
Organizations of all sizes are now realizing that cloud computing risks can at times elude governance considerations, such as internal controls assessments, as these external service providers are not considered part of the enterprise risk management and monitoring framework in addition to overstated entity reliance on vendor controls (Committee of Sponsoring Organizations of the Treadway Commission [44,45]. Increasing controls coverage designed to reduce perceived risks and increase trust surrounding the use of cloud computing in the accounting community can be achieved through improved governance with expanded general controls audit procedures covering all cloud services in their entirety, moving beyond the physical realm [46]. As SMEs are increasingly moving data to the cloud, the importance of security preparedness in the form of access controls, password management, two factor authentication and data encryption along with a detailed understanding of service providers terms of service are essential factors in protecting data and securing business survival [47]. Businesses of all sizes must also be vigilant against threats posed by employee actions, both those of the cloud provider and the company itself and ensure that there are processes in place to trace and track malicious employee behavior [48]. Although the risks and threats associated with cloud adoption are plentiful, businesses believe that with proper mitigation the mission-critical nature of the technology supports adoption of this technology [49].
Theory
A theoretical approach based on and the construct of perceived risk [50], the concepts of the Technology Acceptance Model (TAM) [51], and Theory of Planned Behavior (TPB) [52], have been utilized in this study to understand and explain the intention and behavior towards adoption of cloud technology by small and medium-sized accounting firms impacted by the global business changes of the COVID-19 pandemic. When looking at the intention to adopt a technology and concerns over risk, the perceived benefits are weighed against the perceived risks. Although cloud computing has been successful in attracting customers, it is still considered a risky technology among smaller businesses with a number of barriers hindering cloud computing adoption, such as lack of internal staff expertise, concern over data security, data migration interoperability, and lack of knowledge of the regulatory and service terms framework [36,53]. Concerns over the continuous availability of services via seamless connectivity, the transition of existing systems to the cloud, and data security [54] were also paramount concerns among smaller firms. Studies have found that privacy risk when considering the disclosure of personal information via the use of Internet platforms was outweighed by the benefits offered by the platform [55]. The influence of perceived usefulness mitigates perceived risk when considering mobile payments and internet banking [56]. The adoption of cloud technology found that perceived risk and subjective norms have a significant effect on trust intention vis-à-vis cloud adoption [57]. In the SME setting, cloud adoption-perceived risks were mainly those surrounding the reputational impact of data security breaches and denial of service issues [50].
TAM takes attributes from core technological variables (those pertaining to system usability) and psychological variables (those pertaining to users’ characteristics) to predict user acceptance of a technology [51]. As a social psychology theory, the TPB aims to explain human behavior and behavioral intentions across different situations. TPB also posits that behavioral intention is influenced by attitude, subjective norms, and perceived behavioral control factors over a given behavior [58]. Perceived risk is a multidimensional construct that studies the linkage between an individual’s perception of potential loss in pursuit of a desired outcome [59,60]. TAM indicates that perceived ease of use (PEOU), the extent to which an individual believes that using a particular system is free of effort, and perceived usefulness (PU), the extent to which an individual believes that using a particular system would improve work performance, [61] are both significant prognosticators of behavioral intention. Perceived usefulness has been proven to be a stronger predictor of behavioral intention than perceived ease of use [62]. Perceived usefulness and perceived ease of use have a significant positive relationship with the behavioral intention to use a technology [63], with recent research showing that the TAM is linked to positive attitudes towards using cloud computing services [64].
The predictive power of TPB stems from the belief that the stronger the intention to engage in a behavior, the more likely its performance is considering three conceptually independent determinants of intention: attitude towards the behavior (ATT), subjective norm (SN), and degree of perceived behavioral control (PBC) [52].
Research Model and Hypotheses
Research Model
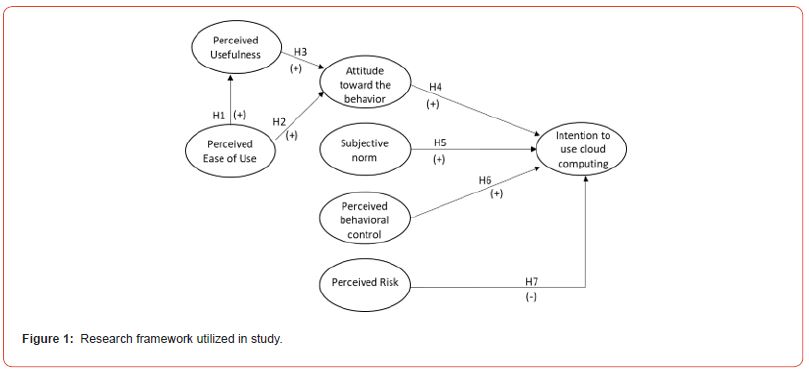
This research is an exploratory study of SME accounting firm leadership perceptions of cloud technology and their likelihood of leveraging this technology in daily operations. Utilizing past work on combining TAM and TPB, this study combined all five factors (usefulness, ease of use, attitude, subjective norms, and behavioral control), as they were shown to have a significant impact on intention to utilize new technology [65]. Furthermore, the perceived risk of technology adoption [50] completes the model by acknowledging the fact that, even though consumers’ attitudes and intentions can drive technology decision-making, risk can still exert influence over decision-making processes and impact the weight placed on other factors, such as intention, attitude, or perceived control [57]. By considering the three factors (attitude, subjective norm, and perceived behavioral control) outlined by Ajzen [58] and the two factors (perceived usefulness and perceived ease of use) outlined by Davis, (1985) the current model has been constructed. Additionally, the perceived risk (Grant, Edgar, Sukumar, & Meyer, 2014) construct was added to this model, as shown in Figure 1.
Hypotheses
As seen in TAM, both PEOU and PU represent users’ cognitive responses to using a technology, which in turn influences a user’s attitude towards a given technology, impacting their intention to use [51, 66]. Attitude (ATT) is the level at which an individual favorably or unfavorably evaluates the behavior being studied [67,68]. The users’ affective response ultimately drives their behavioral response towards the technology with all things being equal; people form intentions to perform behaviors towards which they have positive effects [61]. It is believed that SME accounting firm leadership will be more open to utilizing cloud technology options to support both internal (staff collaboration and data analytics) and external (client interface and data management) activities if they find these options easy to use and useful for accomplishing personnel support and client acquisition/retention goals. Based on these views, the following hypotheses are proposed:
1. H1- Perceived ease of use (PEOU) positively impacts decision makers’ perceptions of the usefulness of cloud computing technology.
2. H2- Perceived ease of use (PEOU) positively impacts decision maker’s attitude towards cloud computing technology.
3. H3- Perceived usefulness (PU) positively impacts decision makers’ attitudes towards cloud computing technology.
4. H4- Positive attitude (ATT) towards cloud computing positively influences intention to adopt cloud computing technology.
The TPB [58], an extension of the theory of reasoned action (TRA) (Ajzen & Fishbein, 1980), appears to provide predictive guidance on individual attitudes towards performing a behavior via the impact of behavioral intentions and behavioral influencers. The constructs of subjective norms (SN) and perceived behavioral control (PBC) incorporate aspects of human behavior to ascertain intentions [69]. Perceived social pressure also impacts planned behavior, with a positive relationship between subjective norms and intention [70,66]. This study explores the linkages between subjective norms and perceived behavioral control in SME accounting firms’ leadership intentions towards adopting cloud technology. In the aftermath of the COVID-19 pandemic, the norms surrounding the use of cloud-supported technologies have shifted, and the following hypotheses are postulated:
1. H5- Subjective norms (SN) positively influences intention to adopt cloud computing technology.
2. H6: Perceived behavioral control (PBC) positively influences the decision maker’s intention to adopt cloud computing technology.
The effects of perceived risk on technology adoption decisions as they relate to the external factors of perceived ease of use and perceived usefulness have been examined to determine the connection and direction of this connection. The study by [50] states that risk in the SME setting is a subjective factor that is impacted by many exterior influencers, such as industry, age of leadership, and staff technological savvy. Technology adoption is also influenced by leadership style, which is a high-touch client model in many SMEs, with these relationships being a part of their success formula [71,72]. Increased reliance on technology to support staff and client interactions could be viewed as risky by accounting SMEs; therefore, the following hypothesis is postulated:
1. H7- Perceived risk (R) negatively influences the decisionmaker’s intention to adopt cloud computing technology.
Materials and Methods
Sample and Procedure
The empirical section of this study was conducted using a questionnaire survey modifying the previously validated scales for perceived usefulness, perceived ease of use, [61,73] attitude, subjective norms, perceived behavioral control, [52,70,68,74,65] perceived risk, [56] and intention to use [52]. The survey was sent to members of the BDO Seidman Alliance, a nationwide association of independently owned local and regional accounting, consulting, and service businesses across the United States, (BDO Alliance, n.d.) which comprises decision makers of small and medium-sized accounting firms. Based on the targeted nature of the underlying population in this study and the P-values, a usable questionnaire sample population of 116 was deemed acceptable [75,76]. The survey response rate was 16%, with a usable response rate of 14%, which was considered acceptable due to the high quality of respondents and the timing of the survey (COVID-19 generated business uncertainty and disruption), both being factors and key features in the study [77,78].
The respondents consisted of 69 percent males and 31 percent females. The majority of respondents were certified public accountants (78%). The ages of the respondents were evenly distributed, with the majority (34%) being between the ages of 51 and 60. The majority of respondents were either senior partners (28%) or owners (24%) of the accounting firm that they worked for. The largest number of respondents (32%) worked in firms with an employee count between 26 and 50. See Table 1 for additional details regarding the makeup and distribution of survey respondents.
Table 1:Summary Statistics of the Make-up and Distribution of Respondents.
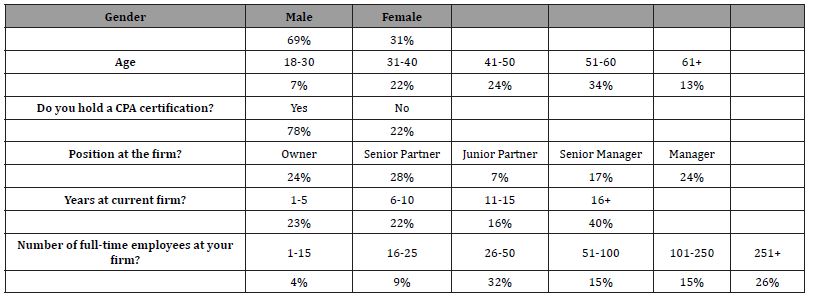
Methodology
Partial least squares structural equation modeling (PLS-SEM) was used to test the hypotheses. In addition to accommodating smaller sample sizes, this methodology has two distinct advantages over ordinary least squares (OLS) regression [79-83]. First, PLS-SEM makes no assumptions regarding the distribution characteristics of the underlying variables. Second, OLS regression does not easily facilitate the inclusion of interaction terms in a model without orthogonality as a design condition. Finally, the majority of the literature discovered during research on the TPB and TAM frameworks revealed the extensive use of the PLS-SEM methodology. Thus, this author sought to preserve the comparability of the results from this study with those attained in prior research.
Results
Measurement Model
To ensure the reliability and validity of the constructs, confirmatory factor analysis was conducted. Convergent validity and discriminant validity were measured using confirmatory factor analysis (factor loading), average variance extracted (AVE), and composite reliability (CR). The measurement tool consists of seven items with a total of 26 factors, with all but one factor above the ideal cutoff criterion of 0.7 [84]. The one factor below 0.7 is at 0.673 which is very close to the 0.7 mark and seen in many studies as a factor loading that is acceptable [85-88], while some have considered factor loading cut-offs of 0.4 and above for their confirmatory factor analysis work [89]. The average variance extracted (AVE) ranged from 0.588 to 0.933, all above the minimum limit of 0.5, to ensure convergent validity of the constructs [90]. Composite reliability (CR) considers indicators with different loadings and is a better indicator of internal consistency when using PLS [91]. CR (the degree to which the construct indicators expressed the latent construct) exceeded the recommended value of 0.7 [84]. The high alpha value was due to the earlier questions designed as part of the TPB and TAM studies.
Discriminant validity differentiates the measure of one construct from another and is seen by examining the correlation between the measures of potential overlapping constructs [90]. This model shows the differentiation in measurement of one construct to another, with all falling well below any overlap threshold and the constructs of intention and attitude, even though close, meeting the threshold requirements. According to Compeau, Higgins and Huff (1999), the average variance shared between each construct and its measure (diagonal values) should be greater than the variance shared between the constructs and other constructs; As seen in Table 2, this model meets these criteria.
Table 2:Overview of Findings Discriminant Validity Findings.
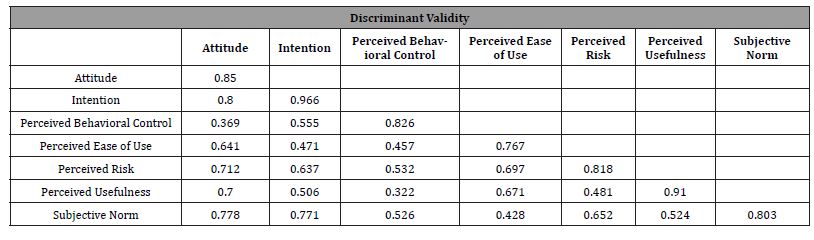
To provide additional discriminant validity detection, the heterotrait-monotrait ratio (HTMT) was used, [92] as shown in Table 3. The resulting HTMT values in this study were below the 0.85 threshold [93,94].
Table 3:Overview of heterotrait-monotrait Ratio (HTMT) Findings.
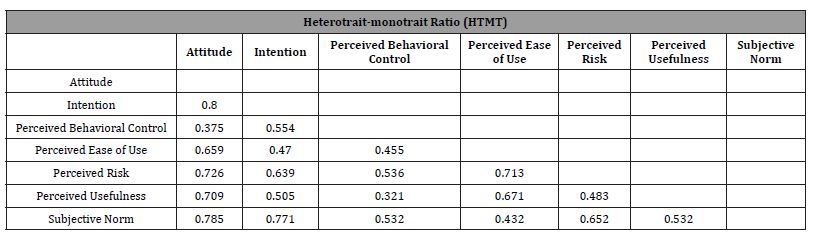
Structural Model
Upon completion of the required measurement tests, the data were examined for model fit. To ensure that there were no multicollinearity issues among the independent factors (PEOU, PU, SN, PBC, PR) and the dependent factors (ATT and INT), variance inflation factors (VIF) were tested. As seen in Table 4, the lateral collinearity measured with the VIF values was under 3.3, indicating no potential collinearity problems [95]. In addition, the structural model is assessed by examining the R2, beta, and via bootstrapping reporting on the corresponding t-values [84].
Table 4:Overview of Variance Inflation Factors (VIF) Findings.
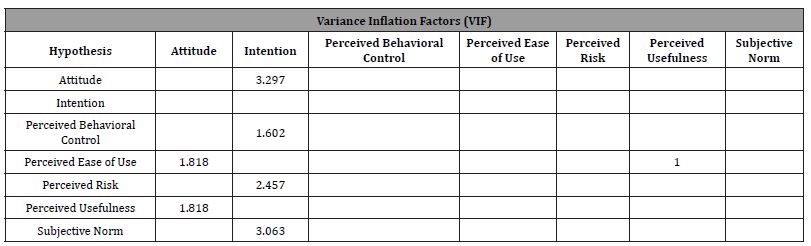
Hypothesis and Model Testing
In addition to confirming the relationships among the constructs, the relevance of significant relationships should be assessed via effect sizes (f2), predictive relevance (Q2), and significance (P Value). Effect size (f2) was used to assess the impact of a predictor construct on an endogenous construct. Predictive relevance or Stone-Geisser’s value (Q2) shows how well observed values are reconstructed by the model and its parameters. First, the relationships between the model variables are examined. Perceived ease of use positively impacted perceived usefulness (β = 0.671; p < 0.05) and attitude (β = 0.311, p < 0.05), while perceived usefulness positively impacted attitude (β = 0.492, p <0.05) and attitude positively affected intention (β = 0.560, p < 0.05), supporting Hypotheses H1, H2, H3 and H4. Subjective norm and perceived behavioral control positively affected intention (β = 0.234, p < 0.05) and (β = 0.251, p < 0.05), supporting Hypotheses H5 and H6. Perceived risk negatively impacted the intention to utilize cloud computing, and H7 was not supported (β = -0.047, p = 0.686). Perceived ease of use of cloud technology explains 45% (R2 = 0.450) of the variance in perceived usefulness, and perceived usefulness and perceived ease of use explain 54.4% (R2 = 0.544) of the variance in attitude. Attitude, subjective norms, perceived behavioral control, and perceived risk explained 73.7% (R2 = 0.737) of the variance associated with the intention to utilize cloud technology (Table 5).
Table 5:Summary of Hypotheses Results.

Next, the effect size (f2) was examined. P value can indicate an effect; however, the p value does not reveal the size of the effect [96]. To understand the effect size, (f2) is considered. Based on the guidelines provided by Cohen (1988), the acceptable effect sizes of (f2) values are 0.35, 0.15, and 0.02, which are considered substantial, medium, and small, respectively. In this model, all relationships, with the exception of risk, had substantial or medium effect sizes. After viewing the R2 and f2 effect sizes, the predictive power of the model was examined using the predictive power-reuse technique (Q2) via the blindfolding procedure suggested by [97]. If the Q2 values are greater than 0, the model is assured to have predictive relevance (Figure 2), and all endogenous variables show acceptable predictive relevance [98].
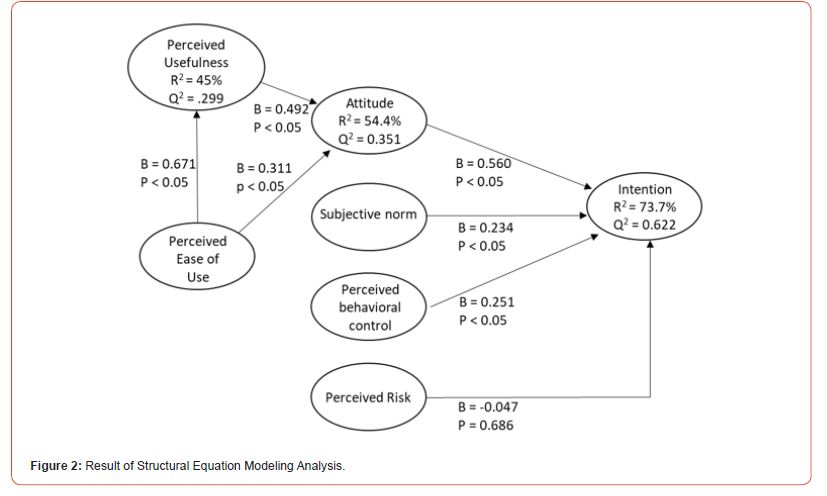
Conclusion and Limitations
This study examines small-and medium-sized accounting leadership perspectives towards cloud-enabled computing technology adoption as a means of meeting customer requirements for digital service offerings and staff demands for a remote work environment in the face of changes post-pandemic. Even though risks are present when considering cloud adoption, perception of these risks was not found to be significant with attitudes and intentions being critical factors affecting the use and integration of cloud-enabled technologies. Leaders in small and medium-sized accounting businesses who have a positive attitude towards cloud technology and find these technologies useful tend to be more open to the adoption of cloud platforms. Based on a review of the literature, this study is the first to study the relationship between perceptions of risk and views on usefulness, ease of use, perceived behavioral control, subjective norm, and attitude on the intention of leadership in small and medium-sized accounting firms to adopt cloud technology.
This research also adds to the body of knowledge surrounding SME adoption of cloud computing technologies by focusing on SME accounting firm leadership perspectives in a post-pandemic environment. SME accounting firms are influenced by their leadership; the technology acceptance model and the theory of planned behavior, which focus on individual factors in technology acceptance, were utilized. This study contributes to the literature by studying the interplay of risk perception with the predictive power of TAM and TPB models in the new setting of cloud adoption. The findings show that all the TAM variables (perceived usefulness and perceived ease of use) significantly influenced the intention to adopt cloud technology. These findings support the original TAM and the findings of other studies on technology adoption [99-101,62]. This study found that the TPB variables (attitude, subjective norm, and planned behavioral control) significantly influenced attitudes towards the adoption of cloud technology, which is supported by other studies on attitude [102,65]. The findings showed that perceived risk did not have a significant influence on the intention to adopt cloud technology. These findings run counter to studies indicating that perceived risk has a negative influence on the intention to adopt new technology [103,57,56]. The results show that when it comes to embracing the cloud in today’s environment, respondents are not concerned about the risks surrounding the adoption of this technology when considering the benefits.
Lastly, this study provides a methodological contribution to the body of knowledge by enhancing the existing risk perception, TAM and TPB scales to capture the impacts of cloud computing and by utilizing partial least squares-based structural equation modeling. Following the extensive literature on risk perception, TAM, and TPB scales, an updated measurement scale was developed, resulting in seven dimensions and 26 items, to capture the multifaceted perspectives on the adoption of cloud technology within the setting of small and medium-sized accounting firms. As cloud computing is becoming a key success factor in the accounting industry, acceptance among firms that this technology must be a part of their assurance business strategy moving forward [104]; Having a targeted measurement tool for use in future research can be beneficial. The use of a PLS approach adds to the body of work that supports the argument that this method of structural equation modeling can be used more often because of its ability to model latent variables using multiple regression in settings with small-tomedium sample sizes [91,84,105].
This study was conducted using a survey of small-and mediumsized accounting firm leadership when the business impacts of the COVID-19 pandemic became visible [106]. It should be noted that this study may not be applicable to all SMEs as the survey respondents and the overall scope of this work is limited to SME accounting firms [107]. Limitations of this work pertain to survey timing and provide opportunities for future research. As the immediate reverberations of the pandemic fade, a post-hoc study on the adoption of cloud-enabled technologies by smalland medium-sized accounting firms could be suitable [108,109]. Suggested topics for future research can focus on SME accounting firm leadership satisfaction with cloud technology choices in the post-pandemic era or leadership experience regarding employee satisfaction, firm productivity, and customer acceptance after moving to the cloud.
Acknowledgement
None.
Conflict of Interest
No conflict of interest.
References
-
Jacqueline T Jamsheed* and Zora P deRham. Perceived Risk in Cloud Computing Technology Adoption in Small and Medium-Sized Accounting Firms. Iris J of Eco & Buss Manag. 1(5): 2023. IJEBM.MS.ID.000522.
-
Cloud technology, Small and medium-sized accounting firms, Small and medium-sized businesses (SMEs), Risk, Risk Perception, Technology Acceptance Model, Theory of Planned Behavior, Employees, Customers, Sufficient knowledge, Understanding, Savings, Efficiency, Continuous availability, Reliability, Agility, Scalability, Flexibility, Elasticity, Quick deployment, Increased storage capacity, Outsourced maintenance, Minimized new software licensing, Innovation, Simultaneous user access, Environmentally friendly solutions
-
This work is licensed under a Creative Commons Attribution-NonCommercial 4.0 International License.