Research Article
Analysis of Pedestrian Crashes with a View Towards Mitigation by Connected Vehicle Technologies
Kiyett Brown1, Ren Moses2 and Eren Ozguven3*
1Graduate Research Assistant, Department of Civil and Environmental Engineering, FAMU-FSU College of Engineering, 2525 Pottsdamer St, Tallahassee, FL 32310, USA
2Professor, Department of Civil and Environmental Engineering, FAMU-FSU College of Engineering, 2525 Pottsdamer St, Tallahassee, FL 32310, USA
3Associate Professor, Department of Civil and Environmental Engineering, FAMU-FSU College of Engineering, 2525 Pottsdamer St, Tallahassee, FL 32310, USA
Eren Ozguven, Associate Professor, Department of Civil and Environmental Engineering, FAMU-FSU College of Engineering, 2525 Pottsdamer St,Tallahassee, FL 32310, USA.
Received Date: June 20, 2022; Published Date: August 09, 2022
Abstract
A comprehensive analysis of pedestrian crashes occurring at intersections was conducted with an underlying hypothesis that mitigation of vehicle-to-pedestrian crashes will be more challenging than mitigation of vehicle-to-vehicle crashes when connected/automated vehicles come to fruition. Pedestrian safety is important when discussing connected and autonomous vehicle (CAV) technology because, with full autonomy, the human driver is no longer making all the decisions for the vehicle. The number of crashes after CAV implementation will reduce because crashes occurring due to improper driver behaviors will be eliminated. Drivers influenced by alcohol, drugs, or medications will not be behind the wheel trying to make cognitive driving decisions. Although most of these crash types will not be eliminated until 100% CAV penetration is reached. According to the crash types and factors discussed in the dataset, the reduction of crashes could be as much as 40% resulting in over $278 million in savings for Florida. This reduction can be increased with behavioral changes in pedestrians as well. Increasing proper use of sidewalks, crosswalks, and waiting for walking phases at intersections will also improve pedestrian safety even without the implementation of CAVs. This paper describes the factors of pedestrian crashes through descriptive analysis of Florida crash data, ADAS applications to help with pedestrian detection, and the reduction of vehicle-pedestrian crashes by implementing ADAS applications leading to fully connected and autonomous technologies, specifically in urban environments.
Keywords:Pedestrians; Pedestrian safety; CAV technologies; Safety; Sensing technology; Advanced driver assistance systems
Introduction
The safety of vulnerable road users, particularly at intersections, is of paramount concern not only in today’s human-driven vehicular environment but also in the future’s connected vehicle environment because roadway, traffic, and environmental factors overwhelm human drivers’ ability to pose similar challenges to machine-based entities. Before explaining the purpose and scope of this research paper, it is important to narrate the body of knowledge related to the study of vehicle-to-pedestrian conflicts and crashes. To this end, a comprehensive literature review of both published and gray literature was undertaken and is briefly discussed below. The review centers on variables associated with a pedestrian crash as categorized in the FHWA-RD-95-163 Report [1].
According to the World Health Organization 2018 [2], the issue of traffic fatalities is steadily increasing. The report states that there were approximately 1.35 million fatalities in 2016. It highlighted vulnerable roadway users, such as pedestrians, cyclists, and motorcyclists represented 54% of the deaths in 2016. Pedestrians specifically represent 23% of the fatalities [2]. Compared to vehicles, these roadway users are vulnerable to injury or fatality due to their limited or non-existent protection, speed, and weight. The interaction between vehicles and pedestrians depends on various factors and situations.
Vehicle-pedestrian interactions occur within and outside of crosswalks with or without traffic signals, within intersections or at mid-block crossings. The occurrence of the interactions all depends on the geometry of the roadway where the pedestrian is trying to use or share the road with vehicles. Within urban environments, more vehicle-pedestrian interactions occur where signals and intersections are present. Urban environments provide a high level of “walkability” that cannot be found in other areas. It is these areas that are of interest to the research team. Pedestrian behavior is often more predictable at intersections due to pedestrian signals and designated crosswalks. However, the more predictable behavior does not limit or reduce the number of vehicle-pedestrian crashes within urban environments. It is believed that the implementation of Advance Driver Assistance Systems (ADAS) leading up to fully connected and autonomous vehicles (CAVs) will increase pedestrians’ safety, especially in urban environments.
Figure 1 shows a flowchart covering the methodological approach instituted to achieve the research objectives.
Figure 1:Research methodological flow chart.
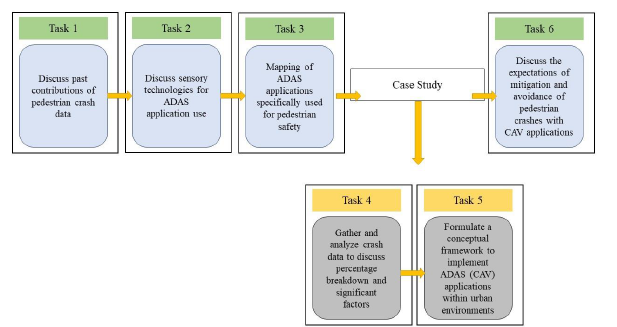
Crashes are random events, and the occurrences of these events cannot be easily predicted. A pedestrian is defined as someone walking along a roadway or in a developed neighborhood. Due to their limited or no protection, they are vulnerable roadway users and are at higher risk of fatality or severe injury if involved in a vehicle crash.
The study focuses on pedestrian crashes occurring in urbanized areas because many initiatives nationwide aim to provide safer streets for walking, especially within the urban environment [3]. The risk of crashes and injuries is increased in dense areas compared to low-density areas. US data shows that roughly 73% of pedestrian fatalities occur in urban environments. This is largely due to increased pedestrian trips and exposure [4]. To this end, an urbanized area was defined using the 2010 U.S. Census Bureau of Urbanized Areas from the Florida Department of Transportation (DOT). According to the 2010 U.S. Census Bureau, there are 30 urbanized areas in Florida based on an urbanized population greater than or equal to 50,000 people [5]. Police crash reports for all crash types involving pedestrians in 2018 were obtained for all urban environments in Florida using a crash database.
This study aimed to conduct a quantitative and qualitative analysis of pedestrian-involved crash data to investigate the challenges connected and automated vehicle technologies will operationally face in tackling ped-vehicle conflicts. Phase 1 involved acquiring and analyzing intersection pedestrian crashes. Phase 2 involved mapping pedestrian crashes as they exist today to potential connected/automated vehicle systems that can mitigate/eliminate and explore psycho-physical challenges to their implementation. Phase 3 involved a conceptual framework of case studies to connect ADAS applications of CAV technology to relatable crash factors and studies.
Researchers have recognized the need to utilize CAVs in transportation engineering. Studies have been conducted to predict the effectiveness and safety impacts that AVs will offer in the future. There are studies investigating the impacts of detecting pedestrians using video imaging with AVs, and these studies are limited to controlled open environments or simulations of the simplest scenarios. There is relatively little work in the literature which considers implementing full CAV technology and smart infrastructure to detect pedestrians in urban areas. One of the main objectives of the study will be to determine which CAV technologies will be useful in identifying pedestrians in urban environments. Conducting a study suggested in the framework within the urban environment will help evaluate how these technologies work in more difficult (realistic) scenarios. Several studies have been conducted through simulation or video processing techniques to determine the accuracy or effectiveness of detection technology. In other words, the impacts of ADAS applications leading to full CAV implementation in urban environments will be investigated to evaluate the potential reductions in pedestrian crashes fatalities that occur in Florida but can be applied to other urban settings. The proposed methodology can enable transportation engineers and car manufacturers to evaluate the impacts of implementing CAV and infrastructure technologies in urban areas to reduce pedestrian crashes and fatalities.
Therefore, the knowledge that will be obtained from the result
of this research could lead to safer environments for pedestrians.
The contributions of this study can be listed as follows:
• Identifying the impacts of ADAS applications leading to fully
connected and autonomous technologies in real-world urban
environments that will help identify pedestrians.
• Identifying the impacts of ADAS applications and CAV
implementation in real-world urban environments that will
help reduce crashes involving pedestrians with vehicles.
• Comparing the types of sensory technology needed within
infrastructure or vehicles to enhance pedestrian detection.
• Identifying the factors of pedestrian crashes that can be
eliminated by implementing ADAS applications with sensing
technologies leading to fully connected and autonomous
vehicles in the transportation network.
The rest of this paper is structured as follows. Section 2 is a summary of pedestrian crash factors, pedestrian behaviors, and driver behaviors that are involved in pedestrian crashes. Section 3 summarizes sensing technology used in ADAS applications, discussing the uses and limitations of the technologies and the current and future capabilities of technology on the pedestrian experience. Section 4 summarizes the ADAS applications of CAV technology that are specifically studied to enhance the pedestrian experience and increase pedestrian safety. Section 5 highlights descriptive statistics of vehicle-pedestrian crashes that occurred in urban environments in Florida in 2018. Section 6 will outline and discuss a conceptual framework to connect crash data and CAV technology to improve the overall pedestrian experience and increase pedestrian safety. Sections 7 and 8 will discuss the overall contributions of the research and provide recommendations for future research.
Vehicle-Pedestrian Crash Factors
In this publication, the variables linked to pedestrian crashes are roadway factors, locational factors, environmental factors, temporal factors, vehicle factors, crash characteristics, pedestrian characteristics, driver characteristics, contributing factors, and fault. A study by National Highway Traffic Safety Administration (NHTSA) in 2017 determined the pre-crash scenario parameters consisted of environmental conditions – atmospheric, lighting, and road surface, pedestrian conditions – age, gender, size, alcohol involvement, and harm functions, and other contributing factors – traffic flow and lanes, travel speed and speed limit, vision obstruction, driver alcohol involvement, and driver distraction [6].
Types of pedestrian crashes
Evaluating the types of crashes between vehicles and pedestrians will help determine what applications are available or can be made available to reduce the impacts of vehicle-pedestrian collisions. It is also helpful to know what factors cause these crashes to occur. Analyzing the significant factors will help identify appropriate ADAS applications (leading to fully connected and autonomous vehicle technologies) as countermeasures to mitigate pedestrian fatalities and injuries. Among Florida crashes, it has been researched what leading factors contributed to pedestrian crashes in 2006. This study found crashes involving vehicles and pedestrians were classified as one of nine types. These types include crossing not in a crosswalk during either the first half or the second half of crossing the roadway; crossing at an intersection; pedestrian in the road; walking along the street either with or against traffic; pedestrian exiting a disabled vehicle; vehicles turning or merging, or unique circumstances not likely to happen again [7]. Another Florida study by [8] on pedestrian safety concluded the significant factors of vehicle-pedestrian crashes to include the percentage of heavy vehicles (HGV), the speed limit of the roadway, pedestrian age, Average Annual Daily Travel (AADT), at-fault pedestrians, weather and lighting conditions, and the time-of-day (TOD). These factors and more will be considered when analyzing vehiclepedestrian crashes in urban environments in Florida.
Analyzing the physical settings, such as vehicle location, pedestrian location, roadway alignment and geometrics, lighting conditions, and surface conditions, will address the most common situations in which vehicle-pedestrian crashes occur. A study conducted by the National Highway Traffic Safety Administration (NHTSA) in 2017 determined vehicle-pedestrian crash parameters from crash data for five years (2005 – 2009), including environmental conditions – atmospheric, lighting, road surface; pedestrian conditions – age, gender, size, alcohol use; and other contributing factors – traffic flow and lanes, travel speed and speed limit, vision obstruction, driver alcohol use, and driver distraction [6]. These factors were supported by another study conducted by the Federal Highway Administration (FHWA). This additional study also added road class, control type, sidewalk presence, shoulder type, pedestrian signal, and median width and additional contributors to vehicle-pedestrian crashes [1]. W Tao, et al. [9] used machine learning techniques with the XGBoost technique to rank factors by their influence on pedestrian severity. The study concluded the top ten factors include speed limit, crash type, age, bus involvement, gender, day of the week, month, national road type, and Christmas period. Another study conducted by [10] supports the factors mentioned above of vehicle-pedestrian crashes. This study investigated vehicle-pedestrian crashes by observing crash data, questionnaires, and post-crash field data. The study determined road users’ features, road characteristics, errors, and intentional or unintentional violations were among the most significant factors causing crashes or conflicts between vehicles and pedestrians.
These types of crashes and their significant factors are again supported by a study conducted by [11]. This study investigated fatal pedestrian crashes in the United States from 2014 to 2018 and found some of the key factors to be categorized with variables such as dark with lighting conditions, vehicles traveling straight, vehicles turning, local roads, and older pedestrians. There were also many crashes where the pedestrian was walking outside the crosswalk area while the lighting conditions were dark with no additional lighting. In addition to this study, another crash analysis study conducted in San Antonio, TX by [12] also suggests the most significant factors of severe pedestrian outcomes are due to lighting conditions, road classification, speed limits, traffic control devices, collision type, age of the pedestrian, and gender of the pedestrian.
Location and roadway factors
The location of the pedestrian and the geometric and physical characteristics of the roadway or intersection can affect the safety of pedestrians and other vulnerable roadway users. The study conducted by FHWA contained crashes from six states, including urban and rural areas, within the dataset. From the data, two-thirds of the crashes occurred in urban areas. Still, pedestrian severity outcomes are more than 2.3 times more likely to be fatal in the rural environment compared to the urban environment [1,4]. This could be because urban environments contain more traffic control devices to slow traffic down. The study by [13] found that 61% of the crashes occurred in a location defined as urban compared to those described as happening in a rural environment. Again, the interactions between vehicles and pedestrians are more consistent in urban environments due to dense populations. At intersections, the roadways are mostly straight and level. Even though, in many studies, it seems as though traffic signals are positive for vehiclepedestrian interaction if an accident occurs (i.e., due to redlight running), the outcome is likely to be more severe [14]. The study conducted by FHWA classified crashes based on road class data; 42% of vehicle-pedestrian crashes occurred on local streets compared to 24% on county routes and 21% on state routes [1].
The number of lanes a pedestrian must cross plays an important role in the time required for the pedestrian to cross safely. If there is no pedestrian signal, this affects the gap needed for pedestrians to cross the entire roadway safely. In the FHWA study, 60% of the vehicle-pedestrian crashes occurred on one- or two-lane streets, and almost 90% occurred on roads with four or fewer lanes [1]. This is also confirmed in two additional studies that found that 25% and 18% of vehicle-pedestrian crashes, occurred on roadways with four or more lanes [6,15]. Roads with four or more lanes are associated with a 0.9% increase in vehicle-pedestrian crashes [16]. The researchers in Philadelphia also concluded that streets with more lanes and higher speeds correspond with an increased number of vehicle-pedestrian crashes throughout the day on weekdays and weekends [17].
The most common roadway surface of vehicle-pedestrian crashes is asphalt, and most of the crashes occur on straight-level areas of roadways. 41% of the crashes in the FHWA study occurred at intersections; traffic signals were present in 19% of the crash locations, and an additional 8% contained stop signs leaving a large percentage without stop-controlled devices [1]. The use of some control devices resulted in less severe crashes. The control devices help lower speeds because vehicles often need to stop or slow down while approaching the control device. The study containing only Florida crashes found that of the 61% urban classified crashes, about 18% of the crashes occurred at an intersection [13]. Pedestrian volumes and vehicular volumes at intersections can be important when discussing vehicle-pedestrian interaction and exposure [14-17]. An increase in pedestrian and vehicular volumes will most likely result in an increase in the frequency of pedestrian crashes [18].
A crosswalk or sidewalk is also essential when analyzing pedestrian safety. Sidewalks and crosswalks give pedestrians a distinct location to walk, usually visible to drivers and clear of vehicle interaction. However, the pedestrian must use these roadway features correctly. It was found in the FHWA study that 21% of the crashes contained marked crosswalks on the roadway and these types of crashes were exceptionally high in California [1]. NHTSA confirms this in their study, concluding that in 44% of crashes at intersections, the pedestrian was in the crosswalk [6]. The presence of a crosswalk does not mean the intersection is safer. In [18], researchers found a study concluding that on multi-lane roads that experience high traffic volumes of at least 12,000 vehicles per day, having a crosswalk alone resulted in higher pedestrian crash rates than not having a crosswalk. This suggests pedestrians are using the crosswalk, but it raises the concern about how the vehicle-pedestrian interaction occurs. Almost all (94%) of the vehicle-pedestrian crashes in the D.C. and Baltimore area included a vehicle performing a turning maneuver within an intersection instead of a driveway or entrances/exits of parking lots. And of these crashes, 45% of the vehicles were turning right compared to leftturning vehicles (55%) [19]. When programming signals in Florida, the pedestrian has the right-of-way to walk during the vehicular movement, so interactions with right-turning vehicles would likely be the driver’s fault, not yielding to the pedestrian unless the driver is turning right on red. Drivers who are waiting for an acceptable gap in traffic to perform a right-turning maneuver are less likely to be paying attention to pedestrians traversing the intersection to their right. The driver’s focus is on approaching traffic from the left. Because of this, drivers do not see pedestrians and thus a conflict, near miss, or collision occurs. A team of researchers found the right turn on red maneuvers accounts for one to three percent of all pedestrian and bicycle crashes [18]. Left turning traffic that interacts with pedestrians would be the fault of the pedestrian not waiting until the walk sign is provided. In [18], researchers concluded that the increased volume of left-turning vehicles is associated with a higher increase in pedestrian crashes compared to the increased volume of right-turning vehicles. Researchers found that changing intersection operations to include an exclusive pedestrian phase increases pedestrian safety by reducing the number of pedestrian crashes even at high pedestrian and vehicular volumes [18].
A study conducted by [20] investigated the perceived pedestrian safety of parents and their willingness to allow their young children to walk to school. The study used six different roadway conditions: sidewalks, landscape buffers, and street trees. The study concluded that the presence of a sidewalk, buffer strip and street tree affected parents’ perception of pedestrian safety. Parents perceived those pedestrian environments to be safer for walking.
Environmental and temporal factors
Temporal and environmental factors include the month and time of day, lighting, weather, and road conditions at the time of the crash. These factors affect the driver’s ability to see or stop the vehicle in time to avoid hitting a pedestrian. Being able to see the pedestrian in enough time to stop or slow down is also attributed to driver inattentiveness, but conditions of the roadway affect the stopping ability of vehicles. It is expected that glare or extreme brightness from the sun at sunrise and sunset can also contribute to the low visibility of pedestrians, signage, and traffic control devices. Findings from several studies indicate how the time of day (TOD) and lighting conditions play a role in pedestrian crashes.
Traffic volumes during the morning (AM peak) and evening (PM peak) weekday peak hours are typically more significant than the off-peak hours. Some cities will experience peak hour volume behaviors during the midday (MD peak). The times of the day that experience high traffic and high pedestrian volumes will be essential to notice and analyze. A study conducted in the Philadelphia area found that higher traffic volumes and increased pedestrian activity are linked with more vehicle-pedestrian crashes on weekdays and weekends throughout the day. The researchers also found on weekdays, there was a 10% increase in traffic volumes, and this caused a rise in expected pedestrian crashes to 0.7% with 95% confidence [17]. The study by [13] found that 60% of the crashes occurred in daylight conditions, about 34% occurred after dark, and about 6% occurred at dusk or dawn, and the time of day that had the highest percentage of crashes was the PM peak hours. FHWA concluded that 58% of the vehicle-pedestrian crashes occurred within the hours of 2:00 and 9:59 pm, 60% occurred during daylight, 23% during dark but lighted conditions, 71% during clear weather conditions, while the roadway surface was dry 83% of the time [1].
Vulnerable roadway users are negatively impacted by the lack of good lighting conditions. The fatality risk and probabilities are increased when driver visibility is low [14]. However, the most substantial negative effect is on pedestrians and their safety. This confirms that good lighting conditions of intersections and other road segments are needed, especially those facilities used heavily by pedestrians. Roadways with no street lighting at night are highly associated with pedestrian fatalities based on research. It is consistent with past claims concluding that well-lit roadways possibly reduce the severity of pedestrian-vehicle crashes. Visibility is a concerning factor in the early morning and evening hours when the sun rises and sets. The sun dramatically affects the driver’s ability to see clearly during these hours. Weather affects the driver’s ability to see, especially during snow or heavy rains. In the NHTSA study, 25,000 crashes occurred in inclement weather [6]. It also affects the driver’s ability to stop suddenly if there is a pedestrian within the direction of travel. A study conducted by [15] found that 76% of the vehicle-pedestrian crashes occurred when the weather was clear, 54% in the daylight and another 28% occurred in dark–lighted conditions. This concludes that the percentage of crashes during times of inclement weather is not high due to drivers changing to more cautious behaviors. A study conducted in D.C. and Baltimore found that there were more crash occurrences during the winter months (October to March) compared to the other months of the year (April to September) [19]. Winters in the D.C. and Baltimore areas consist of snow and ice precipitation. It is known that driving in these conditions is more challenging to stop vehicles suddenly to avoid hitting pedestrians. The weather, visibility (during blizzards), and roadway conditions affect the ability to avoid hitting pedestrians in these instances. Due to their geographical occurrence, these roadway conditions will not be studied or modeled. Such roadway conditions are not prevalent in every region, unlike clear days experienced by every region.
A Riyadh, Saudi Arabia study found that Saturday had the highest percentage of vehicle-pedestrian crashes (18%). Wednesday was the second-highest day of the week, with 16% of the vehiclepedestrian crashes. The lowest rate of vehicle-pedestrian crashes occurred on Friday with 10%. It was also found that 66% of vehiclepedestrian crashes occurred within the last third of the day (4:00 – 11:59 PM) [21].
Vehicle factors
With vehicle factors, two things are analyzed – the type of vehicle and the point of contact. The type of vehicle is essential in distinguishing between light trucks and vans (LTV) versus passenger cars or other vehicles. LTVs consist of large vans, large pickup trucks, large utility trucks, compact pickup trucks, and minivans. One study of vehicle-pedestrian crashes found that almost 70% of the vehicle-pedestrian crashes involved a passenger car, followed by 16% involving pickup trucks [1]. It was also found that almost two-thirds of the vehicles contacted the pedestrian on the front, right front, or left front portion of the vehicle. In about 12% of the crashes, the pedestrian contacted the hood, windshield, or multiple vehicle points [1]. When breaking the data into vehicle types, the number of deaths amongst pedestrians and LTVs increased by 10% compared to the previous year [22]. This study in 2004 also indicated that all categories of LTVs have a higher pedestrian risk than cars. It was also concluded by [22] that when a large van struck pedestrians, 13.3% died because of vehicle-pedestrian interaction. For vehicles, the fatality percentage was 4.5% and for SUVs was 11.5%. This information indicates that pedestrians struck by a van or SUV are almost three times and two times more likely to suffer a fatal injury compared to being struck by a car, respectively. This further suggests that the size of the vehicle matters when it comes to vehicle-pedestrian interaction.
The point of contact of the pedestrian goes together with the size of the vehicle and the speed of the vehicle. Pedestrians struck by vehicles can suffer injuries to a wide range of body parts. This is due to the differing heights of the pedestrians and the different sizes of vehicles. Speed and size affect both the probability of occurrence as well as the severity of the outcome of the collision. Pedestrians and vulnerable roadway users are affected more by the speed of the vehicle compared to collisions only involving vehicles. Pedestrians that LTV strikes are impacted differently than pedestrians hit by a passenger car. DE Lefler [22] found that in vehicles traveling at any given speed slower than 38 mph, the probability of the pedestrians experiencing a severe head, chest, and lower-body injury is higher when LTVs strike the pedestrian than in passenger cars.
Pedestrian behaviors
The improvement of pedestrian safety in the connected/ automated vehicle environment requires we additionally review current crash characteristics, pedestrian characteristics, driver characteristics, contributing factors, and fault assignment in relation to crashes occurring today, i.e., pre-connected vehicle driving environment. The exact trajectory and injuries involved in the collisions occurring between vehicles and pedestrians depend upon many factors that include pedestrian factors such as age and height; crash factors such as vehicle speed and impact angle; vehicle factors such as vehicle type. Describing the types of pedestrians involved in vehicle-pedestrian crashes will give a better understanding of the percentages of pedestrians within specific age groups. Understanding the ages of the pedestrians involved in crashes will give information concerning the heights of the individuals and their ability to make quick decisions or movements.
There are three movements that are relevant to pedestrian behavior. These movements include lateral movements, linear movements, and collision avoidance. The lateral movements include the pedestrian’s ability to change their path behavior, linear movements relate to the pedestrian’s speed and steps moving forward in a specified direction, and collision avoidance movements are due to avoiding collisions with other pedestrians [23]. Previous studies have concluded that pedestrian behaviors change when there is a crossing signal versus when there is not a crossing signal present. It was found that over 95% of pedestrians crossed the street regardless of other factors when there was the presence of a crosswalk or signalization compared to less than 50% when a crosswalk is not present [24]. The presence of crosswalks at signalized intersections almost makes the driver’s behavior irrelevant because pedestrians expect the driver to comply with the law and stop at the signal. When a pedestrian is crossing at an unsignalized intersection or mid-block, crossing the intersection can be dangerous. The gap acceptance of pedestrians differs among age and gender demographics. Older and younger pedestrians need more time to cross the street and therefore will accept longer gap times [4]. The study conducted by NHTSA concluded that 61% of the vehicles in the crashes observed were traveling in a straight path when the crash occurred. Roughly 78% of the pedestrians in the crashes had no recorded actions, improper crossing, or darting/running onto the road [6]. This can be supported by the study conducted in Riyadh as well; 22% of the vehicle-ped crashes in the study occurred near or within a crosswalk, and 36% of the pedestrians were struck while facing traffic (or walking in the opposite direction of travel) and 20% were struck while their backs were facing traffic (or walking in the direction of traffic) [21]. While 21% of the pedestrians were performing an improper crossing of a roadway or intersection and 18% of the pedestrians were recorded as darting or running into the roadway [6].
Pedestrian behaviors also change depending on traffic characteristics, road environments, and individual subjective factors. When traffic speeds and volumes change, pedestrians change their behaviors to accommodate the changes in traffic. Pedestrian behaviors differ when approaching different types of intersections as well. C Gruden, et al. [25] & C Gruden, et al. [26] conducted a survey of pedestrians’ perceived safety of roundabouts and signalized intersections using eye-tracking devices. The respondents suggested that signalization could help improve pedestrian safety, but the field test of their study showed pedestrians usually do not pay attention to the signals. This same study also investigated pedestrian reaction times when they are distracted by phone use compared to those pedestrians who do not use cell phones when crossing intersections at a roundabout. C Gruden, et al. [25] concluded the use of cell phones when trying to cross an intersection, affects the pedestrian reaction time. Eye contact is the main form of communication between pedestrians and drivers when there is no pedestrian signalization provided. This communication is weakened when either party is distracted. These distractions have further negative impacts on pedestrian safety. These findings are further supported by [27] who compared national and international studies of cognitive distractions of pedestrians through phone usage. These distractions reduce risk awareness and increase dangerous behaviors by pedestrians at intersections in urban environments. The results of this study also indicate that the distraction by cell phone usage results in pedestrians disregarding signs and leads to slower crossing speeds. These behaviors put pedestrians at a greater risk of being involved in a crash
Changed pedestrian behaviors also occur as the crossing walking distance increases or decreases. Pedestrians will also decide to take the shortest route possible when walking. The use of walking bridges and tunnels is ideal, but pedestrians are less inclined to walk upstairs to access the walking bridge when walking directly across the street is faster. These risky behaviors also change depending on the pedestrian composition. If there are children present, pedestrians tend to choose less risky behaviors even if it means increasing walking distance [4].
Young pedestrians have different crossing behaviors compared to adults and this behavior also differs when there are adults present compared to when adults are not present. MS Riaz [28] conducted a study of child pedestrian crossing behaviors at a crosswalk in front of their school in Belgium. The study found that over half of the crossings in the study contained one or more behaviors that placed children in unsafe scenarios. The most unsafe behavior recorded was children not stopping at the curb before crossing the intersection. Not looking for oncoming traffic before and during the crossing maneuver was the second most unsafe behavior performed by primary school children. When adults were holding the hands of the children as they approached the intersection led to safer behaviors by the children. The children walked safely across the intersection and were less distracted when accompanied by an adult. These findings can be expounded on by [29] where children were recorded at crosswalks near primary schools in urban environments at locations prone to vehicle-pedestrian crashes involving children. This study concluded that the crossing speed of young pedestrians decreases while walking in a group. It also concluded that the age of children, adult supervision, and the use of cell phones influence the walking behaviors of children.
It has been recommended that some CAV applications work better among adults versus children due to their height or inability to see pedestrians due to view obstructions such as parked vehicles along the roadways. Younger pedestrians are more vulnerable to the severity of pedestrian crashes. Their bodies are smaller and more fragile compared to young and middle-aged adults. Within the FHWA study, 30% of the pedestrians were children under 15 years of age, and 50% were under 25 years old; however, less than 10% of the pedestrians involved were 65 years old or older [1]. Their study determined that young people were overrepresented in the crash data, and the elderly population was underrepresented based on population percentages when the study was conducted. At the study time, only 22% of the population was 15 years old or younger, and 36% of the population was under 25 years old. M Vehicles [13] conducted a study of Florida crashes and 33% of crashes involved pedestrians under 18 years old. Over-representation of younger and elderly populations will differ depending on the study region. Younger pedestrians within [4] study discussed children being involved in pedestrian crashes due to the lack of sensory or cognitive ability to manage modern traffic complexities. In Florida, elderly populations will be more significant in the state’s southern areas than in the northern or panhandle areas.
It is also known that male pedestrians and female pedestrians behave differently at intersections. Over 60% of the pedestrians involved in vehicle-pedestrian crashes in the FHWA study were males. The race of either the pedestrian or driver is not always known or reported within crash data, but the race of pedestrians was available for the crashes in the study by [1] containing crashes from Florida and North Carolina. There was a disproportionately higher percentage of black pedestrians in the crash data. And an additional study by [13] found that white males were involved in pedestrian crashes 40% of the time. However, the study looking at pedestrian crashes conducted in Washington D.C., and Baltimore found that 62% of the pedestrians were female in Washington and 52% in Baltimore [19]. A study conducted in Iran investigated the differing behaviors amongst Persian pedestrians. The study concluded that there are significant differences in pedestrian behaviors across age, gender, and income levels. Their findings also suggest that young, males are riskier in their behavior compared to older and female pedestrians concluding that pedestrians with risky pedestrian behaviors also have risky behaviors as drivers [30]. This would suggest differences among males, females, races, and ethnicity in pedestrian behaviors depending on the region. It has not been determined how pedestrians have different behaviors across racial demographics, so the race of pedestrians will not be studied or commented on heavily within this study. It will be noted if this information is provided within the crash data provided for this study.
Due to the lack of protection, pedestrian crashes are more likely to result in injury or fatality. These roadway users are the most vulnerable. These users do not have an outer layer of protection to shield them from feeling the brunt of a collision. During the study conducted that looked at vehicle-pedestrian crashes in the Washington D.C. and Baltimore areas, researchers found that only about 2% of crashes resulted in fatalities [19]. Within the FHWA study, there are several noteworthy findings. These include: 6% of the crashes resulted in a fatality, and 27% resulted in a severe injury; one out of every five pedestrians killed in a crash was 65 years old or older; pedestrians were impaired from alcohol or drugs in 15% of the crashes; alcohol involvement was most found in pedestrians between 25 and 44 years old [1]. S Das, et al. [31] conducted a study using supervised association rules to identify pedestrian crashes and fatality patterns. The study concluded two significant associations among pedestrians involved in pedestrianvehicle crashes; (1) impaired pedestrians are involved in crashes even when roadways and facilities are well-lit, and (2) middle-aged male pedestrians (35 – 54) were more significant in numbers when grouping the impaired pedestrians. The percentage of fatalities within these two studies seems small. However, one fatality is too many when addressing the number of deaths and their socioeconomic impacts.
Pedestrian factors that contribute to pedestrian-vehicle crashes include when the pedestrian decides to cross, the orientation of the pedestrian, the number of pedestrians crossing at the time, and the pedestrian’s appearance [32]. The study conducted in Riyadh found that 46% of vehicle-pedestrian crashes were attributed to pedestrian behavior. In the crashes studied it was concluded that 65% of the pedestrians were not paying attention and were crossing the roadway without being in a crosswalk. In addition to this 11% of the crashes in the dataset consisted of children playing in the roadway [21]. Clothing of pedestrians plays a key role in the visibility of the pedestrians, especially at night and the decision of where the pedestrian crosses influences driver behavior.
Driver behaviors
Drivers of different age groups have differing driving behaviors. However, a common behavior that causes crashes is the driver’s inattentiveness. NHTSA reported that nearly 80% of vehicle crashes and 65% of near misses involve drivers that are not paying attention at least three seconds before the incident [33]. Younger drivers are less experienced and may drive cautiously compared to drivers between 23 – 64 years old. Older drivers may also drive more cautiously because their cognitive skills and reaction times are slower, while drivers between 25 – 64 are more aggressive drivers. Older drivers are less likely to be distracted by texting or cell phone use than drivers of the younger demographics. Older drivers also take longer to perform specific driving tasks like turning maneuvers. These differences in driving behaviors play a role in pedestrians’ ability to cross or walk along the road safely. FHWA found that 27% of drivers involved in a vehicle-pedestrian crash were between the ages of 16 and 24 years old versus 29% of this same age demographic involved in all vehicle crashes [1]. This demographic of drivers is not only a significant portion of all crashes that occur on the roadway, but they are also involved in many pedestrian crashes. This significant involvement could be due to a lack of experience or increased distractions while performing driving tasks. Drivers at least 25 years or older in the D.C. and Baltimore area involved in vehicle-pedestrian crashes were 70% and 65% male, respectively [19] Supporting the claim of drivers over 25 years old have more aggressive driving behaviors. Another study conducted in Riyadh; Saudi Arabia found that drivers under 18 were responsible for 11% of all pedestrian crashes in the dataset. It was also found that drivers between 18 and 30 were responsible for 57% of the crashes, and 12% of the drivers were considered illiterate [21]. A smaller percentage of drivers under 18 are involved in vehicle-pedestrian crashes, supporting more cautious driving behaviors.
Compared to pedestrians, drivers are shielded by vehicles they are in from injuries or fatalities during vehicle-pedestrian crashes. Very few drivers experience any injuries because of the collision. Drivers’ alcohol use among vehicle-pedestrian crashes is less than 6% in the dataset studied by [1]. These drivers would fall into the 12 – 64-year-old group compared to other age groups discussed in this section. The study conducted in Riyadh found that drivers under the age of 18 were responsible for 11% of all pedestrian crashes in the dataset. It also found that drivers aged between 18 and 30 were responsible for 57% of the crashes [21]. The eye level of the driver is a crucial factor when determining the driver’s stopping capability.
A study conducted in the Cape Coast Metropolis, Ghana investigated driver compliance and pedestrian safety at zebra crossings. The study investigated crashes from 2007 to 2016 and found that 35.4% of the crashes resulted in pedestrians being hospitalized and 15.2% resulted in fatalities [34]. The study also found that over 70% of the crashes occurred at places other than an intersection and there were more than 23 zebra crossings present in the metropolis area. Even though there were zebra crossings present the study concluded that there is a very low compliance rate among the drivers. This driver behavior can be supported by another study conducted in Poland by [35] that concluded drivers frequently exceeded the speed limit near pedestrian crossings. This type of behavior from drivers placed pedestrians in unsafe scenarios and can be limited or avoided with the implementation of uniform cautious behaviors of CAVs.
Contributing factors
Contributing factors can include pedestrian, vehicle, roadway, or environmental factors that contribute to the cause of the crash. FHWA concluded that two-thirds of pedestrians were responsible for at least one contributing factor. The most common pedestrian contributing factors include running into the road, failing to yield to traffic, alcohol impairment, walking between parked vehicles, or walking in the wrong direction. Of the contributing factors listed, running into the road has the highest percentage with 15% [1]. An impaired pedestrian is an essential factor in the frequency of pedestrian crashes. Pedestrians under the influence of alcohol or other drugs tend to behave more dangerous than sober pedestrians [31]. In the Riyadh study, 46% of the vehicle-pedestrian crashes were attributed to pedestrians’ behavior. It was concluded that 65% of the pedestrians were not paying attention and were crossing the roadway outside of the crosswalk, and 11% of the crashes were attributed to children playing in the roadway [21]. A study by [13] found that in 34% of the crashes, pedestrians did not make any improper actions that led to the crash. In comparison, 13% noted the pedestrian did not yield to the right-of-way, and 2% were alcohol or drug impaired. In addition, the study conducted in San Antonio, TX concluded that failure to yield to the right of way and driver distractions were primary contributing factors in vehiclepedestrian crashes. The study also concluded that incapacitating and fatal outcomes are more likely to occur when the pedestrians’ behavior is the primary cause of the crash [12]. An international study conducted in Bangladesh supports but also expands on these risky behaviors of pedestrians. The study concludes that crossing in an oblique manner, running patterns and cell phone usage while crossing were the riskiest behaviors performed by pedestrians [36].
Roadway or environmental factors also include glare or vision obstruction. Still, the majority (75%) of the crashes in the dataset do not have any road or environmental factors listed as contributing factors to the vehicle-pedestrian crash [1]. However, in the NHTSA study, 13% of the vehicle-pedestrian crashes include an object obstructing the driver’s view as a contributing factor. Although 45% of the crashes do not have a driver contributing factor, some factors listed have contributed to vehicle-pedestrian crashes. Driver factors include but are not limited to hit-and-run (16%), failing to yield to pedestrians (15%), improper backing (5%), exceeding the speed limit (4%), alcohol impairment (4%), and distracted driver (4%). The study conducted by [15] confirms the other studies’ findings. They found that 51% of the drivers involved in vehiclepedestrian crashes are not distracted, and 43% of the crashes did not have recorded information about the driver being distracted.
The above roadway, traffic, and environmental factors are not likely to go away as connected and automated vehicles are implemented and therefore there is a need for field experimentation and simulation analysis of how to overcome the challenges they pose in the machine-based driving environment to mitigate pedestrian fatalities and achieve “Zero fatality” vision of connected-automated vehicles implementation.
Sensing Technologies
Intelligent Transportation Systems (ITS) aims to apply information technology, communications, sensor technology, and the internet to transportation systems to improve travel, safety, and other performance measures within the roadway system. Integrating sensor-based technologies and connected vehicle communications is needed to enable CAVs. This environment can include other vehicles (vehicle-to-vehicle or V2V), infrastructure (vehicle-to-infrastructure or V2I), pedestrians (vehicle-topedestrian or V2P), and networks (vehicle-to-network or V2N), or everything (vehicle-to-everything or V2X). Future technologies of ITS include roadside units, onboard units, and wireless communication devices. Roadside Units (RSUs) help provide wireless communication from the infrastructure to On-Board Units (OBUs) located inside vehicles. The RSUs and OBUs use 5.9 GHz Directed Short-Range Communication (DSRC) to communicate between infrastructure and vehicles with low latency. The RSUs are placed at each intersection connected to the traffic controller. The RSU can deliver information from the Traffic Management Center to the OBU located inside vehicles. The RSU also includes information about the intersection geometry and relays this information to the OBU displayed on the screen in the vehicle. This allows the driver to see the geometrics of the intersection as the driver is approaching the specified location. Increasing RSUs’ ability to detect and monitor pedestrian behavior at intersections will provide drivers with information about the location of pedestrians and their possible intentions. The OBUs not only receive information but can also send information to the RSU (infrastructure). The OBU can then send location as well as approaching speed.
To integrate these sensory technologies with connected vehicle communications, DSRC will play a vital role because it is currently the only short-range wireless alternative that provides low latency, high reliability, security, and privacy [37]. One of the trivial problems for the vehicle is to perceive its surroundings, pedestrians included, in real-time and make real-time decisions. Receiving information from the surrounding environment requires CAVs to depend on advanced sensing technologies and devices [38]. Sensors play an important role in autonomous vehicle safety. The safety of the vehicle relies on the onboard computing system. This system depends on the sensors’ ability to ‘see’ the surrounding environment and make decisions. The automotive industry has been increasing sensory technology to perform equal to and potentially better than human drivers. But recent crashes among AVs show the existing sensors cannot reliably detect other vehicles, pedestrians, or bicyclists [39]. Table 1 compares the different types of sensory technology that can detect pedestrians [3,37,40]. This table displays the advantages, disadvantages, user types, and typical uses related to pedestrian detection. Some of these sensory technologies are currently manufactured into vehicles, and other sensor technologies will need to be incorporated into the roadway.
Table 1:Comparison of sensory technologies.
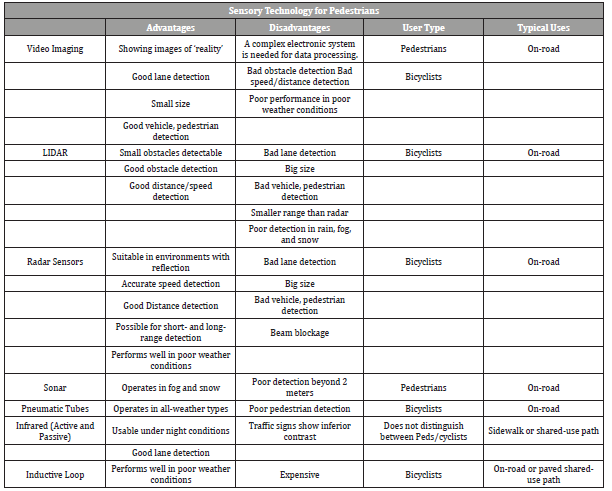
The sensing technology used with vehicles must be able to perceive its surrounding environment in real-time and under allweather and lighting conditions. The technology must view other vehicles, traffic signs/signals, and roadway geometrics while maintaining the optimal route [38]. Figure 2 shows the need for multiple sensors to achieve this state-of-the-art level for sensing the surroundings of CAVs in the most complex environment such as urban areas. For example, cameras provide a digital image for the region, requiring a complex processing algorithm to obtain information from the 2D image. However, cameras’ challenges include their sensitivity to the low intensity of light and capturing images within very bright and dark areas [41].
Figure 2 not only shows where each sensory technology is placed within the vehicle, but also shows the range of sensing technologies. This depicts how multiple sensing technologies can work together to inform the vehicle of its surroundings. A Papadoulis, et al. [42] evaluated the safety impact of competing traffic scenarios that occur in urban environments where interactions are more complicated will answer when the safety benefits of CAVs will be maximized. During more complex scenarios, occlusion amongst pedestrians is not uniform, and the objects that cause occlusion vary but exploring this could help improve the performance of detecting pedestrians. The use of vehicle sensory technology and video imaging algorithms have difficulty incorporating motion features with a moving camera, and motion is an essential cue for pedestrian perception [43].
Figure 2:Major ADAS sensor types and vehicle positions [41].
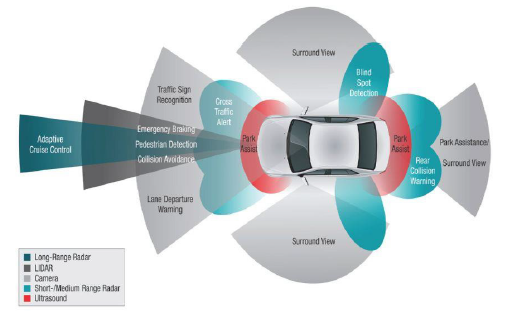
Radar sensing technology has the advantage of being less affected by weather conditions. However, the emitted signal must be restricted to a narrow beam to achieve a good angular resolution [38]. But an essential advantage of radar is the sensors’ ability to determine the relative speed of detected objects. Because of this, most sensing technology devices are better detectors when they use a combination of camera, radar, and even LIDAR sensing technology.
Typical pedestrian accident prevention systems involve using onboard sensors to detect pedestrians. The system is designed to prompt the driver to act when there is a potential collision with a pedestrian. The system can also work if the driver fails to perform using ADAS applications such as automatic emergency braking. Any safety feature implemented by ADAS applications must accurately receive information about the surrounding environments of the vehicle. However, some may find this type of system distracting, especially if the system warns the driver every time there is a pedestrian within a 100 ft radius of the vehicle. In this case, the driver may turn off the system, making the supportive technology useless [44].
A review of engineering measures that can be implemented to reduce pedestrian-vehicle crashes suggests using automatic pedestrian detection at walk signals and in-pavement flashing lights to warn or alert drivers of pedestrians crossing [45]. To reduce the uncertainty within these sensory technologies, it has been suggested to improve the development of sensor fusion algorithms, use more sensors, or incorporate V2V and V2I communications. The addition of V2V and V2I communication will help to reduce the uncertainty with localization [40]. However, the sensor fusion approach also has data reliability and range limitations. No data can be collected when the object in question is not visible to sensors or if the line of sight is obstructed, especially regarding communication between vehicles and infrastructure.
A wireless vehicular communication system (V2CV) can be viewed as an automotive sensor that will enhance sensing technologies in new ADAS applications. V2CV will enable drivers to exchange information about their own vehicles in addition to their surrounding environments. The onboard sensors provide information using cameras, LIDAR, and RADAR to estimate the vehicle’s location, relative speed, and direction. But V2CV communications will include data about the vehicle’s yaw rate, steering angle, and speed, but can also extend communication capabilities to infrastructure and vulnerable roadway users equipped with wireless communications [33]. Just like with any sensing technologies previously mentioned V2CV communications have positives and negatives. It is believed the implementation of V2CV technologies will enhance the perception capabilities of detection in places where the line of sight for onboard sensors is obscured. V2CV communications are not affected by weather or lighting and provide are a more accurate account of the location of the vehicle and the target. Negative aspects of V2CV include delayed or lost communication of radio frequency due to environmental blockage or increased usage such as congested urban environments. Vehicles must be equipped with communication devices to exchange and receive safety messages. Also, the accuracy of positioning is heavily reliant on the global navigation satellite systems signals, so, these signals can be lost in tunnels and under an overpass [33]. Figure 3 describes the positives and negatives of both V2CV communications and onboard sensors discussed in Table 1.
Figure 3:Positive and negative characteristics of V2CV and on-board sensors [33].
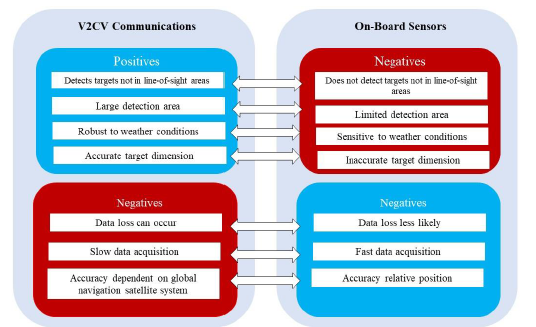
Sensor fusion is needed in the future to receive the most benefits from ADAS applications to better equip CAVs. Sensor fusion integrates data gathered from multiple sensors to reduce the detection uncertainties and overcomes the disadvantages of individual sensors’ operation independently. Sensor fusion will also help develop consistency in models that can perceive surroundings accurately in various and complex environmental conditions. Sensor fusion techniques in addition to algorithms have been studied together over the years to produce better detection quality. Some of the algorithms used with sensory fusion include but are not limited to Residual Networks (ResNet), Single-Shot Multibox Detector (SSD), CenterNet, or Kalman filtering [46]. There are three approaches to combining sensory data from various technologies: high-level, low-level, and mid-level fusion. High-level fusion fuses data together that is collected from each sensor independently. It is mostly adopted due to the lower level of complexity, but it also can provide inadequate information [46]. Low-level fusion fuses data at its lowest level – raw data and mid-level (feature level) fusion are in between high and low-level fusion. Just as sensing technologies have their strengths and weakness, there are also pros and cons to sensor fusion. Table 2 outlines the pros and cons of each level of sensor fusion.
Table 2:Comparative overview of sensor fusion approaches [46].
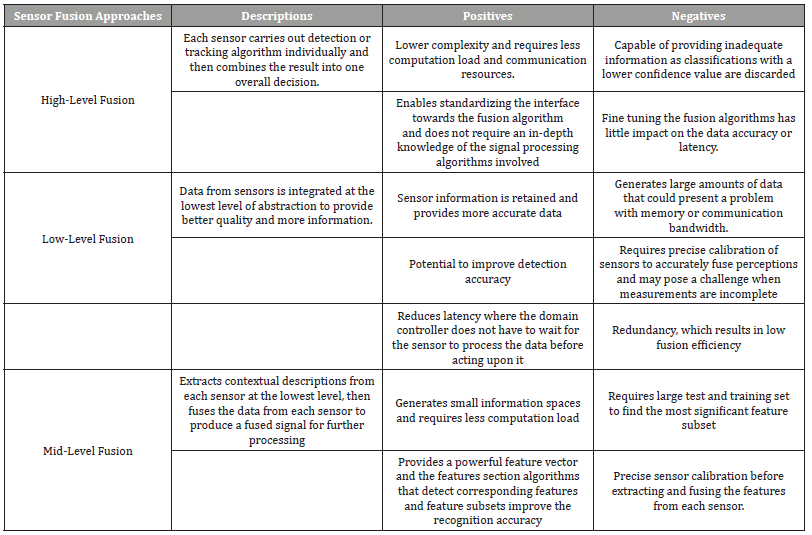
M Baek, et al. [33] proposed an approach for designing a warning system based on fusion sensors and wireless vehicular communications which are considered high-level fusion. The study used Kalman filtering which is a recursive algorithm that keeps track of the state estimate. The researcher of that study used wireless communication and fusion sensors to obtain information about the host vehicle. The study demonstrated that the proposed system enhanced driver and pedestrian safety through perception performance and proper collision warnings. This was based on the time-to-collision estimates from the trajectory predictions and risk assessment steps. From this data, the driver received an audible warning if there was a possible collision [33].
With all the advancements in the technology to reach the point of provided support and assistance to drivers through sensing technologies, there are still some shortcomings of the technology. There are still difficulties in the detection accuracy of certain pedestrians. Mainly youth and elderly pedestrians. NY Abdullah, et al. [47] and M Brandao [48] conducted studies on detection accuracy of pedestrian across age groups. The studies found gaps in the detection performance in pedestrians that were from 0 – 10 years old and older than 55 years old. Improving this technology would help to increase the safety of pedestrians and the actions of vehicles within the control logic algorithms.
ADAS Application for Pedestrians
Advanced Driver Assistance Systems (ADAS) is a vehicular control system that uses environmental inputs through sensor technology to improve driving comfortability and safety by aiding drivers in recognizing and reacting to traffic situations that are potentially dangerous. Studies have been conducted on singular ADAS applications on certain types of pedestrian crashes. Table 3 lists ADAS applications, the percent reduction of crashes, and the types of pedestrian crashes studied. These studies consist of simulations based on real vehicle-pedestrian interaction. Each of these studies deals with interactions that are possible in the real driving environment, but they do not include every weather possibility that can occur while driving. The studies do not discuss the differences in pedestrian behaviors and do not account for differing pedestrian behaviors within the studies.
Table 3:Mapping of ADAS applications for pedestrian crashes.
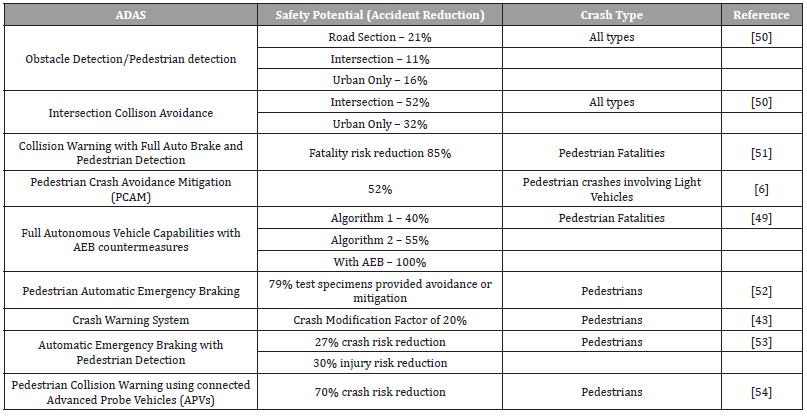
Testing fully autonomous vehicles in the field with pedestrian safety as the focus has not been done yet. However, [49] used two algorithms (rules for AVs to follow) to test the capabilities of avoiding or mitigating pedestrian fatalities. Algorithm 1 used the simple traffic rules to determine what percent of crashes can be avoided and Algorithm 2 used the simple traffic rules plus additional rules to allow the AV to drive cautiously in pedestrian scenarios. It was found to mitigate all possible fatalities the use of AEB will need to be added to the AV capabilities. The additional use of AEB with Algorithm 2 could potentially avoid 100% of pedestrian fatalities. When the AV follows simple traffic rules, only 40% of fatalities can be avoided. The study however assumed 100% sensing capabilities without considering adverse weather conditions. These limitations will be taken into consideration in the following discussion section of this paper.
The study conducted by [50] included crashes that occurred on both rural and urban highways. The percent reduction was split between crashes that occurred near or within the intersection and crashes that occurred on-road sections. The results of the study found various percent increases in safety due to the implementation of ADAS application. The ADAS applications in use and most aligned with this study are the use of obstacle detection/ pedestrian detection and intersection collision avoidance based on the analysis of conflicts. For this study, we are interested in the effects of ADAS applications in the urban environment. The study found the application of obstacle/pedestrian detection will have a potential effect of decreasing the crash frequency by 16% on urban highways and the application of intersection collision avoidance will have a potential effect of 32% on urban highways.
Automatic Emergency Braking (AEB) is used within many ADAS applications to avoid or mitigate vehicle-pedestrian crashes. Currently, AEB applications are in use to avoid rear-end collisions, but AEB can be used in all types of crashes that can occur on the roadway including vehicle-pedestrian crashes. There are several studies discussed within this section of the paper that focus on the advantages of AEB in mitigating or reducing pedestrian crashes. Collision warning with full auto brake and pedestrian detection (CWAB-PD) is one example of AEB being used in ADAS applications for the purpose of increasing pedestrian safety. Collision warning with full auto brake and pedestrian detection has been studied over the years, but [51] uses the third generation of the Volvo system that is implemented to help drivers avoid collisions. The third generation provides fully automatic braking up to 10 m/s2, warning and brake support as well as automatic emergency braking in pedestrian crashes, and automatic collision warning [51]. The use of automatic emergency braking is to avoid or mitigate crashes in cases where the driver is unable to react in a timely manner. In this study, the collision warning with full auto brake and pedestrian detection provides a warning and brake support when there is a risk of a crash. If the driver does not intervene after the warning, braking support is automatically applied to help slow the car down. In this instance, the crash may not be avoidable, but the speed of the vehicle will be reduced significantly. Mitigation, in this case, is obtained by decreasing the speed of the vehicle when the driver does not react in time and thereby decreasing the severity of the vehiclepedestrian crash. When the speeds of vehicles are reduced from 50 to 25 km/h pedestrian fatality risk factors are reduced by 85% [51]. The Pedestrian Crash Avoidance Mitigation (PCAM) also uses an automatic braking system along with forward-looking detection sensors such as RADAR and/or cameras to detect pedestrians in front of a forward-moving vehicle [6]. PCAM also uses the braking system to reduce the speed of approaching vehicles to either avoid or mitigate vehicle-pedestrian crashes and it was found the application has a 52% effectiveness rate. These safety benefits are expressed in terms of pedestrian injuries avoided or mitigated. The final study discussed in this section about the use of AEB involves testing different vehicles (specimen) that have pedestrian automatic emergency braking capabilities. This study uses P – AEB which consists of three functionalities; FCW to warn the driver of a conflicting situation, Crash Imminent Braking (CIB) to apply brakes if the driver ignores the warning, and Dynamic Braking Support (DBS) to provide brake support in situations where the driver does not provide enough braking force. Each vehicle in the study was equipped with system capabilities and focused on four testing scenarios. Scenario 1 consists of an adult running in the path of the vehicle’s far side with an impact point in the middle (50%) of the width of the vehicle (CPFA – 50). The second and third scenarios are an adult walking crossing the path of the vehicle from the near side of the vehicle with an impact location at the passenger side of the vehicle (25%) width and driver side (75%) of the vehicle (CPNA – 25/75). And the last scenario involved a child running from behind to near side road-dies obstructing vehicles with an impact location in the middle of the vehicle (50%) width (CPNC – 50) [52]. This study found that more crashes were mitigated within the CPNA – 75 scenario. Since only 79% of the vehicles showed mitigation or reduction results, that means there were four vehicles that did not mitigate or reduce pedestrian crashes even though they were said to have P – AEB technology. JB Cicchino [53] also uses AEB but incorporates pedestrian detection to assess the effects of pedestrian risk. The study uses vehicles with the ADAS application and compared them to vehicles where the ADAS application was optional. The results of the study show that pedestrian crash risk and injury risk decreased by 27% and 30%, respectively. This study supports the claims that AEB with pedestrian detecting will reduce the number of pedestrian crashes. This study did however not test the vehicles in dark or traveling in high-speed conditions, but the effectiveness of the application is significant.
A study conducted by [55] evaluated the effectiveness of ADAS application in addressing interactions with transit vehicles and pedestrians. A crash warning system was used in the study to mitigate and avoid pedestrian crashes. The transit vehicles were equipped with Mobileye cameras with an image processing algorithm. The study consists of four cameras being placed for front and side sensing of pedestrians and bicyclists and three displays for visual and audible alerting of pedestrians or cyclists. The study resulted in increased reaction times to pedestrians when warnings were provided to the transit drivers. These increased reaction times led to improved driver behaviors such as yielding to pedestrians or bicyclists. These actions resulted in a crash modification factor of 20% being assigned to pedestrian warning systems. K Matsuo, et al. [54] also uses a crash warning ADAS application in their study in addition to connected advanced probe vehicles. The study proposed a two-step Empirical Bayes (EB) estimation based on the safety performance function model. Based on the data collected from the APV, the vehicle-pedestrian crash count models were estimated using the actual pedestrian collision warning rates and the EB estimates of incident rate. The model with the EB estimates of the pedestrian collision warning incidence rate revealed that vehiclepedestrian crash risk decreased by almost 70% compared to the model without the pedestrian collision warning incidence rate. This helps support the claim that the APV data used in the warning information can improve the estimation in determining the effect of a traffic safety treatment.
Florida Vehicle-Pedestrian Crash Data
It has been previously discussed that increased exposure of volumes of pedestrians increases the frequency of pedestrian crashes that a site experience. However, there is a challenge in obtaining pedestrian exposure data for all sites due to agencies lacking data collection processes. This section will however discuss and analyze the crash data obtained from the Signal four Analytics database. The results of the data analysis of the crashes are given below. The data shows the percentages of each category that was evaluated in analyzing vehicle-pedestrian crashes. As stated above the crash data is from 2018 and was obtained from the State of Florida Department of Transportation database. The subset of crashes analyzed contains only crashes that involve pedestrians. There is a total of 3684 crashes in the entire dataset.
With crashes involving vehicle-pedestrian interaction, it is often the result in injury or fatality given the speed and composition of the vehicle compared to the pedestrian. The crashes were then analyzed based on common events. These events include things such as driver or pedestrian behavior, common roadway characteristics, or common pre-crash movements. They were also analyzed based on the common crash types based on severity, roadway identifiers, and point of contact. A significant number of crashes provided information about responsible parties as well as behaviors that caused the crash. The responsible party or contributing factor is any action, decision, or event that led directly to the crash. These factors could be assigned to the driver or the pedestrian involved in the crash. Having identified the responsible party is important in determining the counteractions that can take place in the future to mitigate the crash or reduce the severity of the crash.
The crashes obtained were broken down by categories based on their common events and types and presented as percentages of each category. The categories include crash severity, driver/ pedestrian characteristics, roadway identifiers, weather conditions, roadway conditions, time of year and time of day, etc. This information gives us details of the conditions and events prior to the crash.
The data in Table 4 are the details of the severity outcomes of the dataset. The data shows approximately 75% of the crashes result in injury (possible, incapacitating, or non-incapacitating), 19% result in property damage only (PDO), and 6% result in fatality. The complete breakdown of percentages is seen in Table 4 below.
Table 4:Severity outcomes of vehicle-pedestrian crashes in Florida urban environments in 2018.
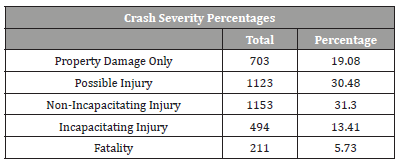
It is imperative with future technologies of connected and autonomous vehicles that the number of injuries and fatalities decrease. To decrease the number of injuries and fatalities caused by pedestrian–vehicle interaction, it is imperative to know how these interactions occur. Table 5 displays the percentages of interactions between pedestrians and vehicles. The breakdown of these interactions is important to help understand the actions of the pedestrian and driver before the crash occurred. Sideswipe crashes between vehicles and pedestrians occur when pedestrians are walking along the roadway either in the same direction or opposite direction of traffic. These crashes would be more likely to occur in places where there is no sidewalk for the pedestrian to traverse or the pedestrian is walking too close to the roadway. Rear to side crashes or rear to rear crashes is most likely to occur within a parking lot. Vehicles backing out of parking spaces are not fully aware of the pedestrians in their surroundings. Front-to-side crashes could occur in multiple settings. Having a pedestrian dart out into traffic within a midblock or within an intersection could be categorized as a front-to-side crash. Also, having a vehicle make a right turn at an intersection while the pedestrian has the right of way results in a crash being categorized as front-to-side crash or an angle crash. Pedestrians are known to cross when they do not have the right-of-way, for instance, when vehicles are at an exclusive left-turn lane within the intersection. Vehicles, in this case, have the right of way and pedestrian signals are more than likely programmed to hold the “Don’t Walk” sign during this phase. It is known that pedestrians will get impatient and will cross when they feel it is safe to cross although it is not safe to cross. The dataset did not have a complete list of reported crash types. Almost 37% of the crashes did not have a crash type, however, when implementing CAV technology, it is imperative to know what types of crashes can occur to better understand where sensory technology needs to be located to detect pedestrians. Although this information is not obtained from the dataset collected, it is still useful in knowing what ADAS applications are necessary to reduce vehicle-ped crashes.
Table 5:Manner of collision amongst pedestrians and vehicles in Florida urban environments in 2018.
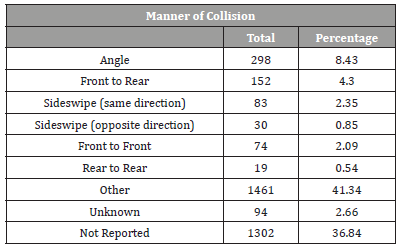
Understanding environmental factors that can contribute to crashes is helpful in knowing what sensory technologies can be used within those situations to improve the visibility and safety of pedestrians. The tables below portray the percentages of environmental factors that can contribute to vehicle-pedestrian crashes. Knowing weather conditions and lighting of crashes goes with the time of year these crashes occur. Table 6(a) – 6(c) are all related to the environmental factors that contribute to vehiclepedestrian crashes in Florida in 2018.
Table 6a:Environmental factors of vehicle-pedestrian crashes in Florida urban environments in 2018.
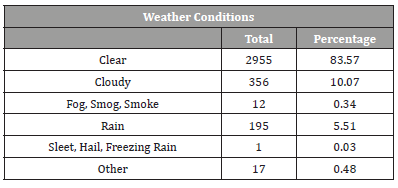
Table 6b:Environmental factors of vehicle-pedestrian crashes in Florida urban environments in 2018.
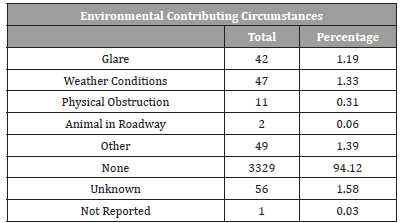
Table 6c:Environmental factors of vehicle-pedestrian crashes in Florida urban environments in 2018.
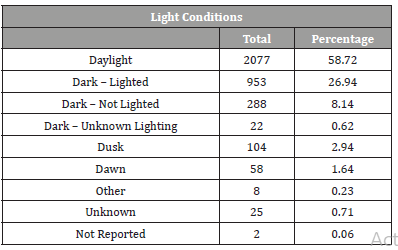
It is seen that approximately 84% of the crashes are occurring on clear days. Current sensory technologies can detect pedestrians on clear days so these crashes will be further investigated to determine if occlusion is a factor contributing to the crash.
Just as the weather conditions are important when analyzing crash data, it is also imperative to know if there are other environmental factors that contribute to the crash. These environmental factors found within the dataset include but are not limited to glare, physical obstruction, or weather conditions. The dataset shows that 94% of the crashes do not have any environmental factors that contribute to the crashes. It will be further analyzed if ADAS applications will be able to reduce the percentage of crashes due to glaring, weather conditions, physical obstruction, and animals within the roadway.
The lighting conditions at the time of the vehicle-ped crash are important to know as well. If the crash occurred on a roadway that is not lit very well, the CAV will have to have better vision than the average driver under those same lighting conditions. The dataset shows that almost 60% of the crashes occurred with the lighting conditions are daylight. Almost 30% of the crashes occurring in the dataset are under dark conditions with lighting and almost 10% under dark, non-lighted conditions. The time of day and lighting conditions go hand in hand when discussing the occurrence of crashes. Since most of the crashes occur while it is daylight, the time of day of occurrences should correspond to the daytime hours. It can be seen from the dataset that most crashes are occurring during daylight hours between 6:00 AM and 7:00 PM. There is a slight edge of crash percentages happening during the PM peak hours of 25% compared to 24% of crashes occurring during the night-midnight hours. Knowing this information will again be useful in knowing how the CAV technology will operate during times of the day when visibility is limited due to lighting.
The road system of the crashes will help to identify which department or municipality operates and maintains the roadway surface. It will be important in the CAV technology that pavement markings are clear and visible. It is also proven that clear distinct pavement markings for the edge of pavement, crosswalks, and stop bars at intersections provide crash modification factors that will lead to a reduction of crashes in the future based on crash history. Table 7 shows the number of crashes and the percentages that occur on each roadway type.
Table 7:Road system identifier for vehicle-pedestrian crashes in Florida urban environments in 2018.
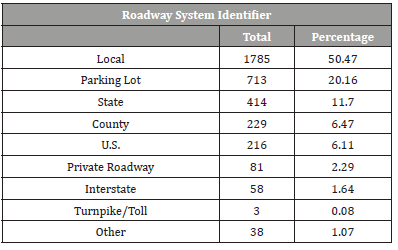
The dataset shows that more than 50% of vehicle-pedestrian crashes occur on local roads. The local roadway system is largely comprised of neighborhood streets. These are roads that are relatively used to handle local traffic. It is very challenging in these types of roadways to provide a high level of safety while also maintaining sufficient access to neighborhood services and facilities. The speed limits on these roadways are usually between 20 and 45 mph and make up most roads in the United States.
Pedestrians across different demographics behave differently. As stated in the literature review, pedestrians located in “college towns” behave very differently from other locations. And with vehicle-pedestrian crashes, there can be more than one pedestrian involved in the crash. The classification of the severity of the crash does not describe how each pedestrian is affected by the crash. Table 8 considers each pedestrian involved in the crashes within the dataset and classifies the severity of the injuries sustained by each.
Table 8:Severity of injuries sustained by pedestrians in vehiclepedestrian crashes in Florida in 2018.
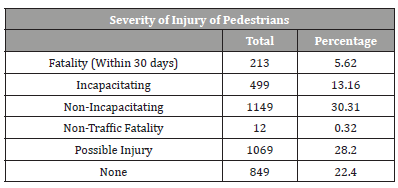
Pedestrians that interact with vehicles often sustain major injuries. Within this data set, almost 6% of the pedestrians involved in the crashes were fatalities within 30 days of the crash, and 13% of pedestrians sustained incapacitating injuries. About 22% of the pedestrians did not sustain any injury at all.
Within the data set, there are younger pedestrians compared to the ages of drivers being limited to 15 years old due to state driving laws. Table 9 shows the breakdown of age groups among the pedestrians involved in the crashes.
Table 9:Age groups of pedestrians in vehicle-pedestrian crashes in Florida in 2018.
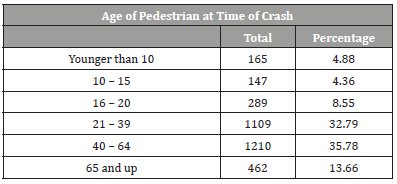
According to Table 9, pedestrians from 21 – 64 make up almost 69% of the pedestrians in the dataset. It is also important to notice that the third-largest percentage group is the older population with almost 14% of the pedestrians involved in the crashes. The age of 21 was used as a reference point due to the alcohol age limit. And according to the data presented in the crash reports, 5% of the pedestrians were suspected of alcohol use at the time of the crash. This is compared to having less than 1% of the pedestrians suspected of drug use at the time of the crash. Also, female pedestrians consist of 42% of the data and males 58%.
It is important to know what the pedestrian was doing before the crash. This information coupled with the pedestrian’s contributing factors gives insight into the ability to avoid crashes if CAV technology is present in the vehicles. In the dataset, pedestrians are classified as walking adjacent to the roadway (3%), crossing the roadway (50%), in the roadway (7%), waiting to cross (2%), walking on the sidewalk (4%), walking along the roadway (against/ with traffic) (5%), no action (7%), or unknown actions (22%) before the crash. These are not actions that contributed to the crash but just a visualization of where the pedestrians are in reference to vehicles along the roadway. The actions of the pedestrian(s) that contributed to the crash include darting/dashing in the road (10%), failure to obey traffic signs, signals, or officer (2%), failure to yield right-of-way (8%), in roadway improperly (7%), not visible to driver (1%), inattentive (1%), no improper action (54%) or unknown actions (15%).
It is also known that drivers behave differently within age groups and sexual orientation. Drivers who have quicker reflexes will tend to be more aggressive than those drivers who have difficulty with vision or slower cognitive abilities. The driving tendencies and actions from older populations will differ drastically from drivers between the ages of 26 – 40 years old. The breakdown of the ages of the drivers in this data set can be seen in Table 10.
Table 10:Ages of drivers in vehicle-pedestrian crashes in Florida in 2018.
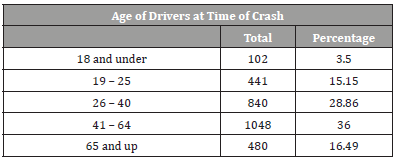
Table 10 shows that the largest percentage of drivers involved in the vehicle-pedestrian crashes in 2018 in Florida were between the ages of 41 and 64 (36%). This age group is followed by 26 – 40 (29%), then the older population of 65 and Up (16%), and then the 19 – 25 age group with 15% of the crashes. The sex of the driver at the time of the crashes was 41% female and 59% male. There are many causes of crashes, and distracted driving is among the most prevalent reasons, especially since the technological advancement of cell phones and the use of social media and texting. Other activities keep drivers distracted in vehicles like applying make-up, eating, or changing the radio station. Roughly 20% of the crashes were due to distracted drivers in the dataset. Of the distracted drivers, the most prevalent distracting activities include inattentiveness, external distractions, distractions inside the vehicle, or electronic devices (texting). In addition, with distractions, there are physical ailments that may cause the driver to lose control of the vehicle or distract the driver. 93% of the drivers appeared to be normal when the crash occurred. However, some drivers appeared the be under the influence of medications, drugs, or alcohol, ill, asleep, tired, or fatigued. These conditions hinder the driver’s cognitive abilities and decisions.
Drivers’ actions can sometimes cause the crash to occur as well. The driver’s actions before the crash are important to understand what the driver was experiencing before the crash occurred. In 51% of the crashes, the driver did not perform any actions that contributed to the crash. However, in the remaining 49% of the crashes, the driver’s actions before the crash contributed to the crash. These behaviors include failing to yield to right-of-way (13%), operating the vehicle in a careless or negligent manner (14%), improper backing (6%), disregarding traffic signs, speeding, improper passing, improper turn, failing to keep proper lane, ran off road, ran stop sign/light, swerved, or operating the vehicle erratically or aggressively (remaining 16% combined).
Other studies have shown that other factors such as alcohol, drugs, and distracted driving are also contributing factors in crashes. When analyzing the data of the crashes from FDOT (Florida Department of Transportation), 98% of the crashes were not considered drug-related, 93% of the crashes were not considered alcohol-related and 92% of the crashes were not considered distracted related. These statistics would indicate the drivers were sober and aware of their surroundings more than 90% of the time a crash occurred between pedestrians and vehicles.
Conceptual Case Study Framework
The main objective of this framework is to set in place research steps that can take place to study the impacts of connected and autonomous vehicle technology on pedestrian safety using the following approaches: (1) analyzing recent crash data, (2) identifying CAV applications needed to improve pedestrian detection, and (3) investigating the relationship between CAV applications and pedestrian fatalities. The conceptual flowchart of the research method is shown in Figure 4.
Figure 4:The conceptual framework of research methodology.
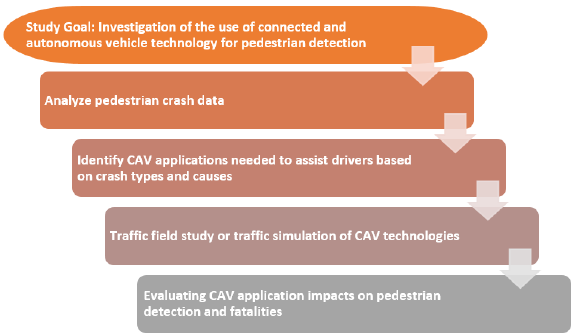
Pedestrian crashes mapping/topology
The first step of the methodology will be to gather recent crash data. This paper has collected Florida pedestrian crash data in 2018 from a crash database. The crashes have been analyzed in section 5 based on common factors and events. The crash data collected from Florida or additional vehicle-pedestrian crash data can be further analyzed within a more detailed case study by using statistical methods to identify the significant factors of the crashes. This study uses significant factors found within the literature review to further analyze the crashes within the collected dataset. The crashes can consist of urban environments like the ones suggested by the Florida Department of Transportation (FDOT) or the researcher can focus on a set of intersections that have the following criteria: 1) be in an urban environment, 2) have high pedestrian volumes, 3) have high vehicular volumes, 4) not be in downtown or central business district, and 5) have a high frequency of pedestrian crashes.
ADAS applications leading to CAV technologies relevant to Pedestrian Safety
Connected and Autonomous Vehicles have the potential to benefit the transportation sector by providing safer and smarter transportation networks. Connectivity is a very important aspect of creating this safer environment. Communication between vehicles, infrastructure, and pedestrians will be essential and knowing what information needs to be communicated between these entities is important. To determine which ADAS applications and CAV technologies will be helpful in identifying potential vehiclepedestrian crashes data will need to be gained from all parties involved.
Data required for pedestrian ADAS applications include:
• type of traffic control for the leg of crossing (intersections –
signalized)
• number of through vehicle lanes on the main street (total
through lanes in both directions)
• 85th percentile traffic speed on the main street
• average daily traffic of the main street
• predominant development type surrounding the intersection
(commercial or non-commercial)
The data gained from this information will help identify which ADAS applications and CAV technologies will be most helpful for each type of crash or each cause of crashes.
Field study
Conducting a field study is one way of evaluating the effectiveness of ADAS applications with smart infrastructure or sensor fusion. Smart infrastructure can consist of sensory technologies such as cameras to detect the presence of pedestrians and track their crossing intention. This technology can also consist of road-side units (RSUs), onboard units (OBUs), and other roadside equipment needed to retain and portray information to drivers. The OBUs can be used to give warnings to drivers about possible pedestrian interaction based on time-to-collision (TTC) information obtained from the cameras and RSUs. The TTC threshold can be used as guidance on when it is appropriate to give drivers warnings of possible collisions with pedestrians. This TTC will be used to only alert drivers when TTC is less than or equal to this threshold. If the TTC is greater than the allowable threshold no alert will be given to the driver. This will limit the amount of distraction to the driver when unnecessary and deter the driver from disabling the application. This part of the study will depend upon the availability of hardware to install at the intersection and the cost of installing the smart infrastructure. It will also depend upon the researchers’ coding capabilities or testing and implementing sensory fusion or algorithms into the vehicles to test ADAS applications and CAV technologies.
Simulation analysis
Under the circumstance that a field study is not applicable simulation analysis can be conducted. All the information gathered to conduct the field study can be used to simulate the real-world environment. The microscopic multi-modal traffic flow simulation software packages such as VISSIM can be used to simulate the traffic scenarios. The Component Object Model (COM) interface in VISSIM can be used to model the V2I technologies. A python code can be written in VISSIM to model a connected vehicle or the preset autonomous vehicle parameters can be adjusted to obtain the connective abilities. Within PTV VISSIM software it can evaluate the behavior of any level of autonomy. It has the capability to create a comprehensive virtual testing environment that can represent realworld traffic conditions and infrastructure. This simulation also allows for different penetration rates of connected and autonomous vehicles. Evaluating different levels of penetration will give a true picture of how connected and autonomous vehicles will help reduce crashes over time. Microsimulation techniques can also be used to model, predict, and analyze pedestrian behavior including those behaviors in urban environments [4].
Evaluate reductions in pedestrian crashes
Upon completing the data analysis and applying ADAS applications leading to CAV technologies within the real-world environment, evaluating the possible reduction in pedestrian crashes will be the last process of the case study. This step will incorporate all information gained from each previous step to evaluate which types of crashes have the potential to be avoided based on the number of connected and autonomous vehicles in the field. This step will also include further statistical applications to show potential reductions in crashes involving pedestrians. Statistical methods inform us of how the implementation works as a reaction and insight into future countermeasures or implementation outcomes. Statistical methods measure performance, indicate what factors influence performance, or predict future results.
Empirical bayes:Analyzing data using Empirical Bayes (EB) method was applied when studying the effect of adding a two-way left-turn lane to an undivided cross-section in Ohio. The University of Dayton conducted this study to estimate the crash reduction after implementing the treatment on the intersections. The two-way left turns were implemented on arterial streets between intersections where left turns affect through-lane traffic [56]. This study was conducted to add further knowledge to an Iowa study that reported an improvement after implementing a two-way left-turn lane.
Locations receiving treatment were selected based on their prior safety performance. Sites of this nature tend to regress towards the mean. Using a statistical methodology that overcomes this tendency is necessary. EB estimation incorporates information about crash rates from similar sites, reduces the impact of regression-tothe- mean analysis. The main idea of EB is the expected number of crashes occurring at a location can be estimated based on the collection of data from similar sites.
The EB method uses the negative binomial regression model to calculate the parameter values for each coefficient. These parameters are used to predict the expected number of crashes at each specified location based on the variables used in the model. These predictions are then compared to the observed number of crashes at each site after implementing treatment. This will determine the crash reduction factor (CRF). The CRF is determined by using the ratio of crashes with improvements to collisions without modification. This method is based on the variables considered significant in the negative binomial model [56]. For this study, the AADT was the only variable used to determine the number of crashes to expect at the selected locations [56].
Negative binomial:In understanding risk factors associated with pedestrian fatalities, a study conducted a statistical analysis using negative binomial models. This study used negative binomial to account for the over-dispersion in the data that often occurs when analyzing crash frequency data [57]. The factors of interest with this study were the type of road median, land use, traffic and pedestrian volumes, adequate lighting, crosswalk, road width, and network planning. The study found that many factors influence pedestrian safety: road infrastructure, built environments, traffic exposure, traffic operations, illegal crossing of pedestrians, red light violations, and alcohol consumption of pedestrians. All the factors are relevant in improving pedestrian safety. The Negative Binomial model referenced was used to predict crash frequency [57]. The study showed a positive association between inadequate sight to distance and pedestrian fatalities. It was also noted that there was improved pedestrian safety at intersections that had marked pedestrian crosswalks, wider sidewalks, and adequate pedestrian phasing.
[58] identified safety surrogate measures for pedestrian exposure at intersections using a two-step process with Tobit and generalized linear models for predicting pedestrian trips and negative binomial and zero-inflated negative binomial models for pedestrian crashes. The Tobit model best identified exposurerelevant factors, and the negative binomial model best predicted the number of pedestrian crashes. It was concluded that these two models could be used to estimate pedestrians’ safety performance functions even when there is no sufficient pedestrian trip data available.
Multinomial logit model:Crossing the road or intersection is an essential task for pedestrians. This action can become complex depending on the volume and geometric characteristics at each intersection. In India, a study was conducted on pedestrian behavior at intersections. This study used a questionnaire to describe their crossing behavior at mid-blocks and intersections. The study took these results and used a multinomial logit (MNL) model to understand the relationship between demographical variables and crossing behavior [59].
Multinomial Logit models are used when optional choices for one or more category is available. This model was derived from the idea that the unobserved factors are uncorrelated and have the same variance for all possible options. Also, there are as many equations for this type of model as there are alternatives in the set. To make parameter estimation possible, the parameter of at least one of the discrete outcomes must be normalized to zero. The coefficients of the parameters are estimated through an iterative maximum likelihood method [59]. In this study and model, a chisquared test for independents was also used to determine the significance of the variables and the model.
An MNL model was used in [60] to study behavioral changes in pedestrians for a study assessment at signalized intersections. The study used MNL to estimate the probability of pedestrians making sudden changes in their directions or speeds at intersections under different influencing factors. The study helped them develop suggestions to understand the pedestrian maneuvers in detail at intersections with a viewpoint of safety.
Discussion
ADAS applications are designed to support the driver in their driving tasks and each additional application reduces the driver’s stress, anxiety, and errors while improving comfortability and decision making. It is the additional applications that will slowly lead to fully connected and autonomous vehicles being added to the modes of transportation. The sections above discussed the types of vehicle-pedestrian crashes that have occurred in past research, the vehicle and driver behaviors that attribute to those crashes, how current and future sensing technology can be used to provide ADAS applications and future connected and autonomous features to improve pedestrian safety. Sections 5 and 6 include a descriptive analysis of vehicle-pedestrian crashes in the state of Florida in 2018 with a conceptual framework to test the connections and implications of connected and autonomous vehicles within real, urban environments. This section of the paper will aim at connecting the sections to further discuss how these crashes in Florida and other states can be mitigated or avoided with the use of connected vehicles.
First knowing some vehicle-pedestrian crashes occur due to reckless driver behaviors such as driving impaired, failing to stop at a stop sign or excessive speeding. Crashes due to these factors can be eliminated with the implementation of fully connected and autonomous vehicles. Drivers will no longer be placed in these situations to make these poor decisions. We know there are many crashes in general that occur each year due to driver impairment. And based on the literature review this is a driver behavior that is a contributing factor in vehicle-pedestrian crashes. The limited driver support of ADAS in these situations may not provide enough driver support to eliminate these types of crashes completely. If the ADAS application warns the driver of a pedestrian in the roadway, the driver still must process that warning, decide what action to take to avoid the pedestrian, and then act. Other ADAS applications will offer braking support if the driver does not respond in enough time, but this support may not eliminate the crash completely if the driver is speeding excessively. The vehicle may slow down enough to not cause a fatality, but the collision may still occur.
As the penetration rate of full CAVs increases, there are types of vehicle-pedestrian crashes that will be eliminated. These include crashes caused by distracted drivers, drivers under the influence of alcohol, drugs, or medications, and drivers who fall asleep, are tired, fatigued, or ill. These crash types make up 27% of the crash data of Florida vehicle-pedestrian crashes. With a 100% penetration rate, these crashes will not occur at all.
Other crash types that can be either avoided or mitigated as the CAV penetration rate increases deal with the programming or aggressiveness of the CAV. The crashes that occurred due to drivers failing to yield to the right of way of pedestrians, careless or negligent maneuvers, drivers who have ignored traffic signs, were speeding, performing improper passing, and turning maneuvers, drivers not staying in their lane, or running stop signs and red lights can all possibly be eliminated. It is understood the operations of CAVs will follow the rules of the roadway and traffic devices, this includes reducing the likelihood of speeding. These types of crashes consist of 43% of the crashes in the dataset.
The dataset of crashes obtained from the state of Florida contains about 6% of fatal crashes. Two studies particularly focused on pedestrian fatalities discussed concluded an 85% reduction in fatality risk and a 100% reduction with the use of fully autonomous vehicle capabilities (algorithm) with the use of AEB. Assuming the vehicles have 100% detectability sensing technologies and all the fatalities that occurred in 2018 happened during daylight without adverse weather conditions, the 6% of fatalities could be reduced to 0%. However, from the discussion within the literature review, we know and understand adverse weather conditions are a factor in attributing to vehicle-pedestrian crashes. Within the dataset, 1% of the crashes occurred in adverse weather and resulted in a fatality. This percent of crashes has not been included within the simulations of studies performed with ADAS applications. The reduction of fatalities within adverse weather is still unknown and will require further research. However, reducing the fatality risk by 85% is very likely considering the likelihood of a fully connected and autonomous vehicle’s ability to respond quickly and reduce speed. It is not concluded that all fatality crashes will be mitigated or avoided, however, it can be concluded that most the fatality crashes can be reduced to an injury crash (incapacitating, nonincapacitating, or possible injury). Again, this all depends on the vehicle’s sensing technology is100% accurate.
The use of AEB and sensing technology can lead to reductions in pedestrian crashes and some of the crashes can be mitigated or avoided. If the vehicles involved in the crashes analyzed in this study have the added ADAS technologies discussed in Table 3, a percent of the crashes observed will be eliminated from the study. The additional ADAS applications will either warn the driver of a conflicting situation, apply brakes for the driver to reduce the speed, or provide brake support for the driver to reduce the speed at a quicker rate. The implementation of fully connected and autonomous vehicles will increase this reduction even more due to the programmed behaviors of the vehicles. Figure 5 below separates factors based on whether the drivers were distracted or not distracted and the percentage of crashes that occurred based on these factors. Since ADAS applications studied in Table 3 will provide warnings of conflicting situations involving pedestrians, it is important to know whether these drivers were aware of their surroundings or not. Figure 5 provides information about the percentage of crashes that occurred when the drivers were distracted versus non-distracted drivers. The crashes involving distracted drivers consist of 8% of the entire dataset. Figure 5 also shows the severity of crashes caused by distracted versus nondistracted drivers and if they were in adverse weather conditions. These weather conditions consist of rain, sleet, hail, freezing rain, smog, fog, or smoke conditions.
Figure 5:Percentages of crashes due to distracted vs non-distracted drivers.
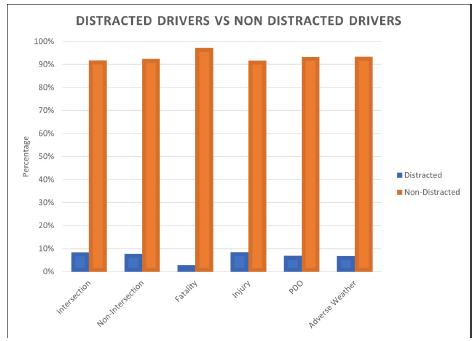
Since 8% of the crashes are attributed to a distracted driver, the warning issued by the vehicle making the driver aware of the conflicting situation could result in either the crash severity being reduced, or the crash being avoided. Depending on the initial speed of the vehicle, 8% of crashes can be avoided altogether. These crashes are more likely to occur on local roads than any other road system due to the limitations of this study being focused on urbanized regions in Florida. On local roads, the highest speed limit would be approximately 45mph. If all 8% of the distracted drivers were traveling 45mph while striking the pedestrian it is most likely the crash would result in a fatality or capacitating injury. Within the dataset, 3% of the crashes caused by distracted drivers resulted in a fatality. From this, one could conclude that the distracted driver was traveling at least 45 mph approximately half of the time when involved in vehicle-pedestrian crashes. If there was a warning given to the driver, automatic braking, or braking support, the percentage of fatalities caused by distracted drivers could be reduced. It is supported in [61] that the reduction of speed leads to a significant reduction in pedestrian fatalities. If the vehicles are fully connected and autonomous, distracted drivers would not exist and these vehicles would abide the posted speed limit, and these crashes could be avoided altogether. The perception-reaction time of the CAV will be faster than the human if the information provided to the vehicles through sensing technology is low latency. The same could be said for the 18% of injuries caused by distracted drivers and 15% of property damage only crashes also caused by distracted drivers. Until full CAVs are implemented, reducing the speed of the vehicle, and making the driver more aware of their surroundings is one way to help reduce the severity of crashes among pedestrians.
The other 92% of vehicle-pedestrian crashes that are not attributed to distracted drivers will be reduced as well based on the studies discussed in Table 5. Since the remaining crashes to discuss in the dataset involve drivers who are aware of their surroundings, it will imply that the ADAS application will either apply the brakes for the driver to allow speed reduction or provide additional brake support to the driver to increase the rate of decreased acceleration. Figure 5 also breaks down the same factors used for non-distracted drivers as distracted drivers.
Although the dataset containing crashes is from urban environments in Florida, there are crashes that do not occur within intersections. When discussing non-intersection versus intersection crashes this also includes each severity type of crash. The additional brake support will either reduce the severity or mitigate the crash by slowing the vehicle down faster than the driver can do on their own based on their comfortability level. In the dataset, approximately 30% of the crashes occurred within an intersection. Of these crashes, 14% occur within an intersection and are not due to a distracted driver. For this subset of crashes within the dataset, the Intersection Collision Avoidance ADAS application can help reduce the percentage of crashes that occur within intersections when drivers are not distracted. This application uses sensing technology to provide additional information to the driver about the surrounding environment as the driver approaches the intersection. Providing the driver with additional information will allow them to make better decisions when approaching the intersection. Of the crashes occurring at intersections, crashes occurring at four-way intersections have the highest percentage (22%) and 92% of those crashes are not related to a distracted driver. This does not give a detailed breakdown of the severity of the crashes or the crashes that occurred within adverse versus non-adverse weather conditions that can still occur, but the overall number of crashes can be reduced with the implementation of the ADAS applications, and this reduction can be increased with the implementation of fully equipped CAVs.
The provided crash data does not indicate the vehicle type involved in the crash, but traffic data would conclude most vehicles involved in the vehicle-pedestrian crashes occurring within the urban environments would consist of light vehicles because of the percentage of light vehicles compared to heavy vehicles that use the roadways within urban environments. A vehicle is considered a heavy vehicle if it is a single-unit truck, bus, recreational vehicle, or tractor-trailer truck. Heavy vehicle interaction with pedestrians within urban environments would be a very small percentage of the crashes within the dataset. Assuming 95% interaction of light vehicles within the Florida dataset would suggest that 5% of the crashes involving a heavy vehicle can possibly still happen even though light vehicles will be fully equipped with connective technology. These crashes will range in severity just as the crashes involving light vehicles. But the study conducted on light vehicle interaction with pedestrians will suggest the percentage of crashes involving light vehicles and pedestrians will be reduced by 52% with the implementation of the Pedestrian Crash Avoidance Mitigation application. This 52% reduction will stem from all severity, crash types, distracted, non-distracted, non-adverse weather conditions, and adverse weather conditions. It is the overlapping of these factors that will lead to an increased reduction in crashes if all ADAS applications are implemented within vehicles and increased safety aspects if vehicles are fully connected and autonomous.
Table 11 below outlines the percent reductions discussed. This table provides a breakdown of crash types that can be reduced with the implementation of ADAS applications leading up to fully connected and autonomous vehicles. From the discussion above all crashes due to distracted drivers can be reduced due to warnings and brake support from ADAS applications. The table includes using a 32% reduction in crashes caused by non-distracted drivers from Table 4 using Intersection Collision Avoidance ADAS application in vehicles. This ADAS application was tested in urban environments and concluded an overall reduction of crashes and not a reduction of risk. The authors assume all crashes are reduced with equal percentages to determine the number of crashes that are avoided. The table also includes information about the costs of crashes in Florida using the KABCO scale.
Table 11:Potential crash reduction and savings in Florida based on KABCO scale.
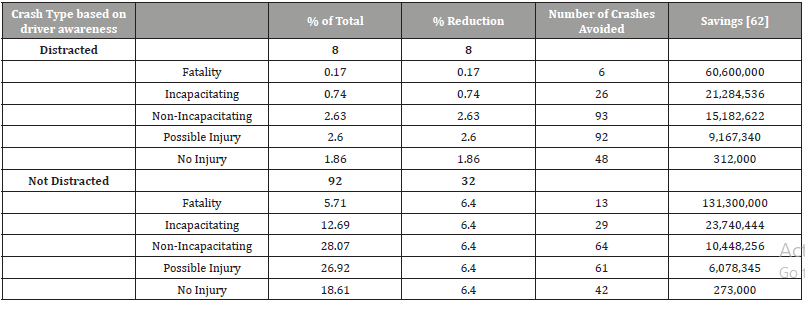
The table indicates the potential reduction of crashes and savings Florida can experience with the implementation of ADAS applications. Based on the costs provided from [62] each fatality in the state of Florida costs the state a little over $10 million, each incapacitating injury costs over $800,000, each non-incapacitating injury costs over $100,000, each possible injury is just under $100,00, and each no injury (property damage only) costs $6,500. With the implementation of one ADAS application, Florida can save a total of over $278 million. These reductions and savings can be increased when fully connected and autonomous vehicles are introduced into the transportation network. These vehicles will be equipped with many ADAS applications that will make driving decisions and maneuvers for humans.
Conclusion
Pedestrian crashes have been prevalent in previous studies and these types of crashes often result in injury or fatality. In this study, we are focused on the pedestrian crashes that occur in the urban environments of Florida. Florida was chosen as the state of reference due to its consistently high number or rate of pedestrian crashes. Pedestrian-vehicle crashes are prevalent in the United States, specifically in Florida. Florida ranks high when comparing pedestrian injuries and fatalities in the United States. The residency of the researchers and access to crash databases played a major factor in choosing the study site for this research. Pedestrian-vehicle crashes are among the most difficult crash interactions to map and categorize due to the many differing pedestrian behaviors that occur. No two pedestrians behave the same and each pedestrian-vehicle interaction is different. However, crashes occurring in Florida are what gave this study a basis for the types of pedestrian crashes that occur and the causes of these crashes. The ADAS applications discussed in this paper can be applied to other pedestrian crash data within the United States or on international roadways. There are many factors that play into the causes of these crash types around the state. Factors include driver and pedestrian characteristics and behaviors, environmental factors, roadway conditions, geometric characteristics, or drug and alcohol involvement. The use of ADAS applications provides drivers with additional information and driver support to make a better decision on the roadways. With 100% sensing efficiency of sensing technologies and algorithms, fully connected and autonomous vehicles can add to the reduction of crashes involving pedestrians. These ADAS applications used for pedestrian interactions will either mitigate or avoid vehicle-pedestrian crashes not only in the state of Florida but throughout the nation.
Although the increased ADAS technology and eventually fully connected and autonomous vehicles will improve pedestrian safety, it is not the only way to improve pedestrian safety in urban environments. There are many countermeasures available such as pedestrian bridges or raised intersections that can also increase pedestrian safety in urban environments. This study is focused on the impact of ADAS applications that will enable us to have fully connected and autonomous vehicles in the United States. It is recommended that these ADAS applications should be implemented in vehicles as a single system or multi-system to help mitigate or avoid future vehicle-pedestrian crashes. These applications should also be continued to be studied in future research with realworld environments and include adverse weather conditions and differing pedestrian behaviors. It is also recommended to further study the implementation of ADAS applications in addition to other pedestrian safety programs to see the full impact of increased pedestrian safety in real-world environments.
Author Contributions
The authors confirm their contribution to the paper as follows: study conception and design: K. Brown, R. Moses, E. Ozguven; data collection: K. Brown; analysis and interpretation of results: K. Brown; draft manuscript preparation: K. Brown, R. Moses, E. Ozguven. All authors reviewed the results and approved the final version of the manuscript.
Acknowledgement and Funding Resources
U.S. Department of Education GAANN Program: GAANN: Meeting the Needs of the Nation’s Infrastructure through Civil Engineering at Florida A&M University (Award #: P200A180074).
Conflict of Interest
No conflict of interest.
References
- L Hunter, W Stutts, C Pein, E Cox (1996) Pedestrian and Bicycle Crash Types of the Early 1990’S. pp. 149-152.
- WH Organization (2018) Global Status Report On Road Safety 2018. Geneva, Switzerland.
- B Davis, K Voros, S Piper (2016) Innovation in Bicycle and Pedestrian Counts.
- G Tiwari (2020) Progress in pedestrian safety research. International Journal of Injury Control and Safety Promotion 27(1): 35-43.
- FDOT (2022) FLORIDA DEPARTMENT OF TRANSPORTATION URBANIZED AREA POPULATION ESTIMATES 2020 Urbanized Areas.
- M Yanagisawa, E Swanson, WG Najm (2014) Target Crashes and Safety Benefits Estimation Methodology For Pedestrian Crash Avoidance / Mitigation Systems (Report No. DOT HS 811 998), pp. 103.
- LK Spainhour, IA Wootton, JO Sobanjo, PA Brady (2006) Causative factors and trends in Florida pedestrian crashes. Transportation Research Record 1982: 90-98.
- K Haleem, P Alluri, A Gan (2015) Analyzing pedestrian crash injury severity at signalized and non-signalized locations. Accident Analysis and Prevention 81: 14-23.
- W Tao, Mahdi Aghaabbasi, Mujahid Ali, Abdulrazak H Almaliki, Rosilawati Zainol, et al. (2022) An Advanced Machine Learning Approach to Predicting Pedestrian Fatality Caused by Road Crashes: A Step toward Sustainable Pedestrian Safety. Sustainability (Switzerland) 14(4).
- A Sheykhfard, F Haghighi, E Papadimitriou, P van Gelder (2021) Review and assessment of different perspectives of vehicle-pedestrian conflicts and crashes: Passive and active analysis approaches. Journal of Traffic and Transportation Engineering (English Edition) 8(5): 681-702.
- S Das, R Tamakloe, H Zubaidi, I Obaid, A Alnedawi (2021) Fatal pedestrian crashes at intersections: Trend mining using association rules. Accident Analysis and Prevention 160: 106306.
- K Billah, HO Sharif, S Dessouky (2021) Analysis of pedestrian-motor vehicle crashes in San Antonio, Texas. Sustainability (Switzerland) 13(12).
- M Vehicles (1994) Descriptive Analysis of Crashes Involving Pedestrians in Florida, 1990 – 1994. 98: 1990-1994.
- P Olszewski, P Szagała, D Rabczenko, A Zielińska (2019) Investigating safety of vulnerable road users in selected EU countries. Journal of Safety Research 68: 49-57.
- S Das, X Sun (2015) Factor association with multiple correspondence analysis in vehicle-pedestrian crashes. Transportation Research Record 2519: 95-103.
- E Dumbaugh, W Li (2011) Designing for the safety of pedestrians, cyclists, and motorists in urban environments. Journal of the American Planning Association 77(1): 69-88.
- E Guerra, X Dong, L Lin, Y Guo (2020) Temporal Analysis of Predictors of Pedestrian Crashes. Transportation Research Record, 2674(8): 252-263.
- National Academies of Sciences Engineering and Medicine, Pedestrian Safety Prediction Methodology. Washington, D.C.: Transportation Research Board.
- DF Preusser, JK Wells, AF Williams, HB Weinstein (2002) Pedestrian crashes in Washington, DC and Baltimore. 34: 703-710.
- BS Kweon, J Rosenblatt-Naderi, CD Ellis, WH Shin, BH Danies (2021) The effects of pedestrian environments on walking behaviors and perception of pedestrian safety. Sustainability (Switzerland) 13(16).
- N Al-Shammari, S Bendak, S Al-Gadhi (2009) In-depth analysis of pedestrian crashes in Riyadh. Traffic Injury Prevention 10(6): 552-559.
- DE Lefler, HC Gabler (2004) The fatality and injury risk of light truck impacts with pedestrians in the United States. Accident Analysis and Prevention 36(2): 295-304.
- X Li, X Yan, X Li, J Wang (2012) Using cellular automata to investigate pedestrian conflicts with vehicles in crosswalk at signalized intersection. Discrete Dynamics in Nature and Society.
- A Rasouli, I Kotseruba, JK Tsotsos (2018) Understanding Pedestrian Behavior in Complex Traffic Scenes. IEEE Transactions on Intelligent Vehicles 3(1): 61-70.
- C Gruden, I Ištoka Otković, M Šraml (2021) Pedestrian safety at roundabouts: Their crossing and glance behavior in the interaction with vehicular traffic. Accident Analysis and Prevention 159.
- C Gruden, II Otković, M Šraml (2021) Safety analysis of young pedestrian behavior at signalized intersections: An eye-tracking study. Sustainability (Switzerland) 13(8).
- T Campisi, II Otković, S Šurdonja, A Deluka-Tibljaš (2022) Impact Of Social and Technological Distraction on Pedestrian Crossing Behaviour: A Case Study in Enna, Sicily. in Transportation Research Procedia 60: 100-107.
- MS Riaz, Ariane Cuenen, Evelien Polders, Muhammad Bilal Akram, Moustafa Houda, et al. (2022) Child Pedestrian Safety: Study of Street-Crossing Behaviour of Primary School Children with Adult Supervision,” Sustainability (Switzerland) 14(3).
- A Deluka-Tibljaš, II Otković, T Campisi, S Šurdonja (2021) Comparative analyses of parameters influencing children pedestrian behavior in conflict zones of urban intersections. Safety 7(1).
- A Esmaili, K Aghabayk, N Parishad, AN Stephens (2021) Investigating the interaction between pedestrian behaviors and crashes through validation of a pedestrian behavior questionnaire (PBQ). Accident Analysis and Prevention 153: 106050.
- S Das, A Dutta, R Avelar, K Dixon, X Sun, et al. (2019) Supervised association rules mining on pedestrian crashes in urban areas: identifying patterns for appropriate countermeasures. International Journal of Urban Sciences 23(1): 30-48.
- A Katz, D Zaidel, A Elgrishi (1975) An experimental study of driver and pedestrian interaction during the crossing conflict. Human Factors 17(5): 514-527.
- M Baek, D Jeong, D Choi, S Lee (2020) Vehicle trajectory prediction and collision warning via fusion of multisensors and wireless vehicular communications. Sensors (Switzerland) 20(1).
- A William, OT Kolawole, OA Comfort, A Francis (2021) Driver compliance and pedestrian safety at zebra crossings in the Cape Coast Metropolis, Ghana. Urban, Planning and Transport Research 9(1): 464-478.
- M Budzynski, A Gobis, L Guminska, L Jelinski, M Kiec, et al. (2021) Assessment of the influence of road infrastructure parameters on the behaviour of drivers and pedestrians in pedestrian crossing areas. Energies (Basel) 14(12).
- NM Zafri, AI Rony, MH Rahman, N Adri (2022) Comparative risk assessment of pedestrian groups and their road-crossing behaviours at intersections in Dhaka, Bangladesh. International Journal of Crashworthiness 27(2): 581-590.
- D Bajpayee (2015) A Comparative study about Autonomous Vehicle. 2015 International Conference on Innovations in Information, Embedded and Communication Systems (ICIIECS), pp. 1-6.
- C Ilas, M Ieee (2013) Electronic Sensing Technologies for Autonomous Ground Vehicles : A Review. 2013 8TH INTERNATIONAL SYMPOSIUM ON ADVANCED TOPICS IN ELECTRICAL ENGINEERING (ATEE), pp. 1-6.
- C Yan, W Xu, J Liu (2017) Can you trust autonomous vehicles: contactless attacks against sensors of self-driving vehicle,” 28th Modern Artificial Intelligence and Cognitive Science Conference, MAICS, pp. 189-190.
- J Van Brummelen, MO Brien, D Gruyer, H Najjaran (2018) Autonomous vehicle perception: The technology of today and tomorrow. Transportation Research Part C: Emerging Technologies 89: 384-406.
- A Narayanan (2018) How Does Advanced Driver Assistance Systems (ADAS) Save Lives and Cars?
- A Papadoulis, M Quddus, M Imprialou (2018) Evaluating the safety impact of connected and autonomous vehicles on motorways. Accident Analysis and Prevention 124: 12-22.
- C Wojek, B Schiele, P Perona (2012) Pedestrian Detection : An Evaluation of the State of the Art. 34(4): 743-761.
- K Tateiwa, K Yamada (2015) Estimating driver awareness of pedestrians in crosswalk in the path of right or left turns at an intersection from vehicle behavior. IEEE Intelligent Vehicles Symposium, Proceedings, pp. 952-957.
- RA Retting, SA Ferguson, AT McCartt (2003) A Review of Evidence-Based Traffic Engineering Measures Designed to Reduce Pedestrian-Motor Vehicle Crashes. American Journal of Public Health 93(9): 1456-1463.
- DJ Yeong, G Velasco-hernandez, J Barry, J Walsh (2021) Sensor and sensor fusion technology in autonomous vehicles: A review. Sensors 21(6): 1-37.
- NY Abdullah, MT Ghazal, N Waisi (2021) Pedestrian age estimation based on deep learning,” Indonesian Journal of Electrical Engineering and Computer Science 22(3): 1548-1555.
- M Brandao (2019) Age and gender bias in pedestrian detection algorithms.
- M Detwiller, HC Gabler (2017) Potential reduction in pedestrian collisions with an autonomous vehicle. 25th International Enhanced Safety of Vehicles (ESV) Conference, pp. 5-8.
- WJR Louwerse, SP Hoogendoorn (2004) ADAS safety impacts on rural and urban highways. IEEE Intelligent Vehicles Symposium, Proceedings, pp. 887-890.
- E Coelingh, A Eidehall, M Bengtsson (2010) Collision warning with full auto brake and pedestrian detection - A practical example of automatic emergency braking. IEEE Conference on Intelligent Transportation Systems, Proceedings, ITSC, pp. 155-160.
- E Meloche, D Charlebois, B Anctil, G Pierre, A Saleh (2019) ADAS Testing in Canada: Could Partial Automation Make Our Roads Safer. in 26th International Technical Conference on the Enhanced Safety of Vehicles (ESV): Technology: Enabling a Safer Tomorrow, pp. 1-17.
- JB Cicchino (2022) Effects of automatic emergency braking systems on pedestrian crash risk.
- K Matsuo, N Chigai, MI Chattha, N Sugiki (2022) Vulnerable road user safety evaluation using probe vehicle data with collision warning information. Accident Analysis and Prevention, 165.
- M Hadi, MA Islam, S Afreen, T Wang (2021) Evaluation of an advanced driver-assistance system to reduce pedestrian and rear-end crashes of transit vehicles. in Transportation Research Record 2675(11): 1301-1309.
- P Hovey, M Chowdhury, Y Zhou, R Fries (2010) Evaluating the safety performance of adding a two-way left-turn lane to an undivided cross-section. Journal of Public Works and Infrastructure 2(4): 363-373.
- D Mukherjee, S Mitra (2020) Identification of Pedestrian Risk Factors Using Negative Binomial Model. Transportation in Developing Economies 6(1).
- J Lee, M Abdel-Aty, I Shah (2019) Evaluation of surrogate measures for pedestrian trips at intersections and crash modeling. Accident Analysis and Prevention 130L 91-98.
- G Noida, N Delhi (2019) Pedestrians Crossing Behavior in Delhi, India. Journal of the Eastern Asia Society for Transportation Studies 13: 831-840.
- WKM Alhajyaseen, M Iryo-Asano (2017) Studying critical pedestrian behavioral changes for the safety assessment at signalized crosswalks. Safety Science 91: 351-360.
- R Goel (2021) A new model to estimate pedestrian deaths from speed-related interventions,” Traffic Injury Prevention, 22(4): 330-335, 2021.
- T Harmon, G Bahar, F Gross (2018) FHWA Safety Program Crash Costs for Highway Safety Analysis.
-
Kiyett Brown, Ren Moses, Eren Ozguven. Analysis of Pedestrian Crashes with a View Towards Mitigation by Connected Vehicle Technologies. Glob J Eng Sci. 9(5): 2022. GJES.MS.ID.000724.
-
Pedestrians, Pedestrian safety, CAV technologies, Safety, Sensing technology, Advanced driver assistance systems
-
This work is licensed under a Creative Commons Attribution-NonCommercial 4.0 International License.