Review Article
Resilience and Uncertainty in Engineering Decisions
Daniel A Vallero*
Department of Civil and Environmental Engineering, Pratt School of Engineering, Duke University, USA
Daniel A Vallero, Adjunct Professor, Duke University, Durham, North Carolina, 27709, USA.
Received Date: August 14, 2019; Published Date: August 21, 2019
Abstract
The safety, health and welfare of the public hold prominence in engineering. No matter the discipline, the public is the engineer’s ultimate and most important client. Decisions regarding types and levels of environmental and public health protection are usually driven and constrained according to the amount of risk removed or added by an action or policy. Risk assessment documents contain the scientific and technical information that underpins these risk-based decisions. A retrospective risk assessment, such as one imbedded in a root cause analysis, attempts to quantify the amount of risk introduced by a previous action. Conversely, a prospective risk assessment predicts the types and amounts of risk that is being and could be added or prevented by an action.
Keywords: Risk; Reliability; Resilience; Precautionary principle; Reliability function; Failure rate; Uncertainty; Pollutant; Treatment technology
Introduction
The safety, health and welfare of the public hold prominence in engineering [1-4]. No matter the discipline, the public is the engineer’s ultimate and most important client. Decisions regarding types and levels of environmental and public health protection are usually driven and constrained according to the amount of risk removed or added by an action or policy [5-16]. Risk assessment documents contain the scientific and technical information that underpins these risk-based decisions. A retrospective risk assessment, such as one imbedded in a root cause analysis, attempts to quantify the amount of risk introduced by a previous action. Conversely, a prospective risk assessment predicts the types and amounts of risk that is being and could be added or prevented by an action [17]. Risk assessments require scientific and engineering data, i.e. evidence-based risk assessment. In the U.S., evidence-based, prospective risk assessment often places the onus on the regulatory body, e.g. a federal or state agency, to whether the risk introduced by an action is acceptable. Usually, these data are requested by the regulator from the applicant for the action, e.g. a permit or notice, but the onus for the action is for the regulatory agency to show that the action would be unsafe or otherwise unacceptable. The precautionary approach, on the other hand, requires that the applicant provide sufficient evidence that the action is sufficiently safe. That is, the onus of proof is on the applicant, not the regulator. Precaution is in order when data needed to properly quantify risks are lacking or insufficient to make an informed decision. Thus, precaution is usually applied to an action with health, safety and environmental impacts that could be sufficiently severe and irreversible [18-20]. The most obvious examples of engineering precaution are those related to bioengineering, given the large uncertainties introduced by genetic manipulations and synthetic biology, especially related to downstream risks. However, there are also numerous civil and structural engineering scenarios in which precaution is appropriate, e.g. hazardous waste site cleanup [21,22], protection of drinking water aquifers [23-25], and construction stability [26].
Intentionally or intuitively, engineers use risk metrics to determine the success or failure of a project. Indeed, engineers are called upon to reduce an existing or potential risk. Ideally, risk reduction is a systematic process whereby the decision to take an action to reduce the risk also incorporates engineering reliability and resilience. Reliability is the probability that the system will perform its intended function for a specified condition, including quality and time [27,28]. Reliability is the flip side of risk, i.e. instead of the likelihood of an adverse and undesirable outcome, reliability expresses the likelihood of a desired outcome. Since both are probabilities, their values range between zero and unity, often expressed as a percentage ranging from 0% to 100%. Complete reliability, i.e. 100%, and conversely, 0% risk are the ideals [29]. Reliability is the extent to which the engineer, the public and other clients have confidence of success. This confidence is often a function of the length of time that a system, process or item operates. A device will be reported to fail at some rate, e.g. x number of failures per hours of operation. A pump is needed to measure particulate matter (PM) in an active system. For example, the pump is expected to fail, on average, once every 1000 hours of operation. If the town engineer uses 10 pumps in the field for 11,000 hours, the average failure rate would indicate one likely failure during that operation time. Thus, the design may not be sufficiently reliable. If the failure is unacceptable, e.g. to meet statistical significance, the engineer would need to procure at least one replacement pump. Thus, reliability is the mathematical expression of success. Reliability is the probability that something that is in operation at time 0 (t0) will still be operating until the designed life (time t = (tt)). The probability of a failure per unit time is the hazard rate or failure density [f(t)] This is a function of the likelihood of an occurrence of failure, but not a function of the severity of the failure [30]. The likelihood of a failure at a given time interval is found by integrating the hazard rate over a defined time interval:
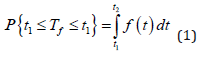
where Tf = time of failure.
Thus, the reliability function R(t), of a system at time t is the cumulative probability that the system has not failed in the time interval from t0 to tt:

It is not a question of “if”, but “when” an engineered system will fail [31]. The optimal time may be extended, e.g. of a pollution control device, by increasing tt to making the device more resilient under conditions that would otherwise lead to failure. For example, proper engineering design of a fabric filter system should work well, not only during “normal” conditions, but resist failure during hostile conditions, e.g. a time-period of highly corrosive particulate matter (PM) loading. In addition to design, the structure and materials may also contribute to failure, e.g. filter material is based on less corrosive pollutant loads than those actual emissions, decreasing Tf [31]. This example illustrates the importance of resilience [32-34]. The less resilient a system, the more sensitive a system is to perturbations and the more that the engineer must control that system to make it sufficiently reliable. A very resilient system can withstand a wide array of insult. A system that lacks resilience is a sensitive and vulnerable system, needing controls to remain operational when conditions change.
Resilience Metrics
Thus, the risk and reliability specifications of engineered systems must incorporate resilience, i.e. able to return to the original form or state after being bent, compressed, or stretched or the ability to recover return to the desired state readily following the application from some form of stress [33-35]. Engineers have traditionally required designs to be sufficiently resilient, whether they used this term explicitly. As mentioned, the specifications of a technology or item included tolerance ranges as well as operational lifetime. For example, an item may perform its function for 10 years, so long as temperature, pressure, corrosiveness and other environmental and operational factors fall within specified tolerances. Thus, the designer sought ways not only to lengthen the lifetime, but also to broaden the tolerances, i.e. make the item more resilient. To achieve this, the item must become [36]:
1. more robust by widening the upper-to-lower performance limits;
2. more resistant to and recoverable from disruptions;
3. reconfigurable by incorporating adaptive properties; and,
4. more adaptable to changing conditions.
Thus, resilient systems include a “learning” process, whereby the operator can be attentive and recall changes and disruptions to anticipate future stresses [36,37]. The engineer can be supported in these anticipatory and cognitive processes by artificial intelligence and machine learning.
Put another way, resilient systems have wide tolerances, whereas an overly sensitive system is easily harmed outside of very narrow tolerance ranges. For example, the town’s wastewater treatment plant should have much greater resilience than most university laboratory experiments, since real-world conditions vary during the design life of the facility. Nature provides an example, i.e. an ecologically resilient habitat can withstand large ranges of moisture, temperature and pollutant loading. For example, atmospheric deposition of a pollutant onto a biologically diverse system may cause little impact and/or require a relatively short time to recover. Conversely, the same deposition onto a non-resilient, less diverse system, e.g. monoculture crop, could be devastating. The stressors are often synergistic, e.g. perhaps drought conditions could be withstood, were it not for the addition of the deposited pollutant [38]. Likewise, an environmental treatment technology that builds in resilience is in less need of intricate engineering controls against insult than a non-resilient system [32,39,40].
Risk and Precaution
In evidence-based risk assessments, the onus is on the environmental or public health regulator to show that an action or agent is unsafe. This is the predominant perspective of most U.S. health and environmental regulations, i.e. it is up to the agency to stop an action, such as a new chemical being used in a product, only if it has sufficient information to support this decision. However, the agency usually may require the applicant to provide such information. Environmental engineers commonly employ chemical or biological risk assessments to address [41]:
1. The amount of a potentially harmful agent in each environmental medium or compartment, e.g. in the soil, water, air, carpet, walls, etc.;
2. The potential exposure to the agent, i.e. the amount of contact with a receptor in each medium and compartment; and,
3. The potential harm, e.g. toxicity, of the agent.
Nations vary in their environmental protection strategies and approaches. For large-scale and irreversible damage, many nations apply precaution, i.e. any requested action is denied unless the applicant can provide sufficient information showing that the action or product is safe [18-20,42,43]. Unlike traditional risk assessments, which assume governance holds the burden of proof that something is harmless, the precautionary onus assumes that the burden of proof is entirely on those who anticipate the new action and if “reasonable suspicion” arises of a severe and potentially irreversible outcome, the action as proposed should be denied. This requires an objective, well-structured, comprehensive analysis of alternatives to provide a needed service, including a “noaction” if any of the alternatives are worse than doing nothing new. Increasingly, evidence-based risk assessments are being augmented or even supplanted by precaution, especially if the decision involves a reasonable likelihood that an adverse effect is severe and irreversible [44]. The precautionary approach also calls for systems thinking and sustainable solutions, which are being supported by emerging screening models and tools, including life cycle analysis (LCA) and multi-criteria decision analysis (MCDA). The key is the ability to consider numerous variables from various information sources. For untested and data-poor agents and processes expert elicitation is employed [45], i.e. gathering insights from a swath of the scientific community on newly emerging or otherwise poorly understood challenges to air quality. At the local level, an engineer may elicit expertise within an organization, through teams, and with stakeholder meetings. The approach will vary by type of design and project, but also for different stages of the same design and project [46-48].
Among the important gaps in data and tools needed for riskbased and precaution-based decision making is more reliable means of estimating and predicting exposures to stressors. For example, human health characterization factors (CFs) in LCAs have benefited from improved hazard, especially toxicity, information [49]. Given that health risk is a function of hazard and exposure, both traditional risk assessment and LCA’s human health CF must be based on reliable exposure predictions [50]. For instance, materials or chemicals used in early life stages could result in the formation and release of pollutants at some later stage, e.g. eliminating wastes before they reach the household or changing chemical synthesis or product manufacturing approaches in early stages that prevent a future problem [51].
Uncertainty
An engineer never enjoys 100% certainty about a design. Indeed, engineers have numerous working definitions of risk other than the product of hazard and exposure to that hazard. Another is that risk is the effect of uncertainty on the engineer’s ability to meet specified objectives. In the latter definition, the “effect” is a deviation from the “expected” outcome [34]. The outcome’s acceptability varies by who is expecting it. The engineer may be satisfied with a treatment technology based it its efficiency in removing a pollutant, whereas policy makers and the public may care most about the costs of the overall treatment facility. This does not mean the public accepts less environmental quality. In fact, they correctly assume that the engineer will ensure that the environment and public health are protected. The engineer should not expect the public to appreciate every drink of clean water but should expect that the public will not and should not accept any water, air or other environmental quality that does not meet accepted standards. Engineering decisions fall into two broad categories [52,53]. The first is a decision under risk; the second, a decision under uncertainty. This is but another example of a twist on the term “risk”. When outcomes cannot be known or are only incompletely known, the decision is said to be under risk. Optimizing for best approaches is less about selecting the “good” versus “bad” options, but about the “good enough, with fewer ancillary negative outcomes” versus “not good enough, with too many unacceptable outcomes”. It is impossible to identify every contributing event for all but the simplest decisions; so, such event trees are often very broad assimilations of possible outcomes. Even if there is a wealth of information about possible outcomes, these outcomes occurred in the past, which is never identically repeated. Engineering uncertainty results from temporal, spatial and operational variability and the lack of knowledge [54]. Perhaps, more than most scientific venues, the factors that lead to environmental risk are highly variable, given the large number of habitats, sources of pollution, diseases in populations, geographic diversity, and many other environmental circumstances. Additional uncertainties result from measurement imprecision, voids and gaps in observations, practical obstacles, lack of consistency in measured and modelled results, and the inability to understand gathered information, i.e. structural uncertainty [55].
Conclusion
The engineer of the 21st century is expected to provide designs and projects that reduce risks with acceptable reliability and resilience. Engineering success is a function of the amount of risk that has been reduced or avoided. Thus, the success of environmental engineering requires vigilance and preparation for uncertainties about future hazards and potential exposures to these hazards. Thus, the engineer is accountable to ensure the designs and operations provide for the public’s safety, health and welfare. This is achieved by managing and reducing risks, using approaches that are reliable and resilient in the face of uncertain and changing operational and environmental conditions.
Acknowledgement
None.
Conflict of Interest
No conflict of interest.
References
- National Society of Professional Engineers (2018) NSPE Code of Ethics for Engineers.
- Hoke T (2016) A Question of Ethics: The Obligation to ‘Hold Paramount ‘the Safety of the Public. Civil Engineering Magazine Archive 86(3): 38-39.
- Walker HW (2016) Moral Foundations of the Engineering Profession. ASEE Mid-Atlantic Section Conference, USA.
- Vesilind PA, Gunn AS (2010) Hold Paramount: The Engineer’s Responsibility to Society. Cengage Learning.
- Abt E, Rodricks JV, Levy JI, Zeise L, Burke TA (2010) Science and decisions: Advancing risk assessment. Risk Analysis 30(7): 1028-1036.
- Public Health Assessment Guidance Manual (2005).
- Council (2002) Environmental Health risk assessment: guidelines for assessing human health risks from environmental hazards. Department of Health and Ageing and EnHealth, USA.
- Egeghy PP, Sheldon LS, Isaacs KK, Özkaynak H, Goldsmith MR, et al. (2016) Computational exposure science: an emerging discipline to support 21st-century risk assessment. Environmental Health Perspectives 124(6): 697.
- Environmental Health Australia (2012) Environmental Health Risk Assessment: Guidelines for Assessing Human Health Risks from Environmental Hazards. Canberra, ACT: Commonwealth of Australia.
- Faber MH, Stewart MG (2003) Risk assessment for civil engineering facilities: critical overview and discussion. Reliability engineering & system safety 80(2): 173-184.
- Morgan MG (1981) Risk assessment: Choosing and managing technology-induced risk: How much risk should we choose to live with? How should we assess and manage the risks we face? IEEE Spectrum, 18(12): 53-60.
- NAoS National Research Council (1983) Risk assessment in the federal government: managing the process. Washington, DC: National Academy Press, USA.
- NAoS National Research Council (2009) Science and decisions: advancing risk assessment.
- Russell M, Gruber M (1987) Risk assessment in environmental policymaking. Science 236(4799): 286-290.
- Suter II GW (2016) Ecological risk assessment. CRC press, USA.
- Environmental health criteria 214: Human exposure assessment (2000).
- Diamond J, Altenburger R, Coors A, Dyer SD, Focazio M, et al. (2018) Use of prospective and retrospective risk assessment methods that simplify chemical mixtures associated with treated domestic wastewater discharges. Environmental toxicology and chemistry 37(3): 690-702.
- Persson E (2016) What are the core ideas behind the Precautionary Principle? Science of The Total Environment 557: 134-141.
- Singh C (2016) The Precautionary Principle and Environment Protection.
- Science & Environmental Health Network (1998) Wingspread Conference on the Precautionary Principle.
- Colombano S, Brunet J, Gilbert D (2015) French public policy for contaminated sites and land management. Book of abstracts 1: 86.
- Sam K, Coulon F, Prpich G (2017) Management of petroleum hydrocarbon contaminated sites in Nigeria: Current challenges and future direction. Land use policy 64: 133-144.
- Correljé D François, Verbeke T (2007) Integrating water management and principles of policy: towards an EU framework? Journal of cleaner production 15(16): 1499-1506.
- M Fuerhacker (2009) EU water framework directive and Stockholm convention. Environmental Science and Pollution Research 16(1): 92-97.
- M Fürhacker (2008) The Water Framework Directive–can we reach the target? Water Science and Technology 57(1): 9-17.
- Al-Kodmany K (2018) The sustainability of tall building developments: A conceptual framework. Buildings 8(1): 7.
- Vandoorne R, Gräbe PJ (2018) Stochastic modelling for the maintenance of life cycle cost of rails using Monte Carlo simulation. Proceedings of the Institution of Mechanical Engineers, Part F: Journal of Rail and Rapid Transit 232(4): 1240-1251.
- Connor PO, Kleyner A (2012) Practical reliability engineering. John Wiley & Sons.
- Vallero DA, Peirce JJ (2003) Engineering the risks of hazardous wastes. Burlington, MA: Butterworth-Heinemann p.306.
- Vallero DA (2014) Excerpted and revised from: Fundamentals of air pollution. Fifth edition. ed. Waltham, MA: Elsevier Academic Press p. 999.
- Vallero DA (2019) Air Pollution Calculations: Quantifying Pollutant Formation, Transport, Transformation, Fate and Risks. Elsevier.
- Elmqvist T, Carl F , Magnus N, Garry P, Jan B, et al. (2003) Response diversity, ecosystem change, and resilience. Frontiers in Ecology and the Environment 1(9): 488-494.
- Hollnagel E, Woods DD, Leveson N (2007) Resilience engineering: Concepts and precepts. Ashgate Publishing.
- Solomon JD (2017) Communicating Reliability, Risk and Resiliency to Decision Makers.
- Solomon JD, Vallero DA (2016) From Our Partners – Communicating Risk and Resiliency: Special Considerations for Rare Events.
- Heinimann HR (2016) A generic framework for resilience assessment. An edited collection of authored pieces comparing, contrasting, and integrating risk and resilience with an emphasis on ways to measure resilience, p. 90.
- Helm P (2015) Risk and resilience: strategies for security. Civil Engineering and Environmental Systems 32(1-2): 100-118.
- Paoletti E, Schaub M, Matyssek R, Wieser G, Augustaitis A, et al. (2010) Advances of air pollution science: from forest decline to multiple-stress effects on forest ecosystem services. Environmental Pollution 158(6): 1986-1989.
- Fiksel J, Goodman I, Hecht A (2014) Resilience: navigating toward a sustainable future. Jour. Solutions 5(5): 38-47.
- Reja MY, Brody SD, Highfield WE, Newman GD (2017) Understanding the notion between resiliency and recovery through a spatial-temporal analysis of section 404 wetland alteration permits before and after hurricane Ike. World Acad Sci Eng Technol Int J Environ Chem Ecol Geo Geophys Eng 11(4): 348-355.
- EPA/100/B-04/001, US Environmental Protection Agency (2004) An examination of EPA risk assessment principles and practices.
- Turvey CG, Mojduszka EM, Pray CE (2005) The Precautionary Principle, the Law of Unintended Consequences, and Biotechnology presented at the Agricultural Biotechnology: Ten Years After, Ravello, Italy.
- Harremoës P, David Gee, Malcolm MacGarvin, Andy Stirling, Jane Keys, et ( 2001) Late lessons from early warnings: the precautionary principle 1896-2000. Office for Official Publications of the European Communities.
- United Nations Environment Programme (1992) Rio declaration on environment and development. ed. Nairobi, Kenya, 1992.
- Wood MD, Plourde K, Larkin S, Egeghy PP, Williams AJ, et al. (2018) Advances on a Decision Analytic Approach to Exposure-Based Chemical Prioritization. Risk Anal.
- Wu W, Holger RM, Graeme CD, Rosemary L, Kathryn B, et al. (2016) Including stakeholder input in formulating and solving real-world optimisation problems: Generic framework and case study. Environmental modelling & software 79: 197-213.
- Bottomley PA, Doyle JR (2001) A comparison of three weight elicitation methods: good, better, and best. Omega 29(6): 553-560.
- US EPA (2011) Expert Elicitation White Paper. Science and Technology Policy Council, Washington, USA.
- Schulte PA, Lauralynn TM, Donna SH, Andrea HO, Gary SD, et al. (2013) Occupational safety and health, green chemistry, and sustainability: a review of areas of convergence. Environmental Health 12(1): 1.
- Vallero DA (2016) Air Pollution Monitoring Changes to Accompany the Transition from a Control to a Systems Focus. Sustainability 8(12): 1216, 2016.
- Gauthier M, Fung M, Panko J, Kingsbury T, Perez AL, et al. (2015) Chemical assessment state of the science: Evaluation of 32 decision‐support tools used to screen and prioritize chemicals. Integrated environmental assessment and management 11(2): 242-255.
- Peterson M (2009) An introduction to decision theory. Cambridge University Press, UK.
- Resnik MD (1987) Choices: An introduction to decision theory. U of Minnesota Press, USA.
- Van Asselt MB (2000) Perspectives on uncertainty and risk. in Perspectives on Uncertainty and Risk: Springer pp. 407-417.
- Vallero DA (2017) Translating Diverse Environmental Data into Reliable Information: How to Coordinate Evidence from Different Sources. Academic Press, USA.
-
Daniel A Vallero. Resilience and Uncertainty in Engineering Decisions. Cur Trends Civil & Struct Eng. 3(4): 2019. CTCSE. MS.ID.000568.
-
Risk, Reliability, Resilience, Precautionary principle, Reliability function, Failure rate, Uncertainty, Pollutant, Treatment technology, Risk assessment
-
This work is licensed under a Creative Commons Attribution-NonCommercial 4.0 International License.