Opinion Article
Refrain From Statistical Testing in Medical Research; It Does More Harm Than Good
Jos WR Twisk*
Department of Epidemiology and Data Science, Amsterdam UMC, Amsterdam, The Netherlands
Jos WR Twisk, Department of Epidemiology and Data Science, Amsterdam UMC, Amsterdam, The Netherlands
Received Date:February 23, 2024; Published Date:March 21, 2024
Abstract
Most medical researchers still believe that statistical testing is important to determine whether an estimated effect is important or not. Unfortunately, this believe is based on several misunderstandings regarding statistical significance. Therefore, the purpose of this paper is to highlight these misunderstandings and to convince medical researchers to refrain from statistical testing. The biggest problem with statistical testing is that it is based on an arbitrary cut-off value and a non-existing dichotomy. Therefore, statistical testing should not be used to evaluate the results of medical research. Instead of using statistical significance, the results should be evaluated by its clinical relevance. It is clear that refraining from statistical testing will be a challenge, but it will definitely improve the evaluation of the impact of medical research.
Keywords:Statistical significance; misinterpretations; misuse; clinical relevance
Introduction
From the moment statistical testing theory was introduced by Fisher, about 100 years ago, its use has been criticized, including by Fisher himself. However, within medical research, statistical testing is still the main method to decide whether an effect estimate is important or not. In the past years, there is a tendency among a few applied methodologists and also some scientific journals, such as the International Journal of Epidemiology, to get rid of statistical testing in medical research [1-14]. Unfortunately, most medical researchers still believe in the importance of statistical testing. This believe is, however, mostly based on several misunderstandings regarding the concept of statistical significance. The purpose of this paper is, therefore, to highlight these misunderstandings and to convince medical researchers to refrain from statistical testing.
Discussion
Misunderstandings regarding statistical significance
The biggest misunderstanding regarding statistical significance is the fact that finding a non-statistically significant result indicates that there is no effect, while finding a statistically significant result indicates that there is an effect. A comparable misunderstanding exists in the evaluation of effect estimates in randomized controlled trials (RCT). When a non-significant result is found in an RCT, the trial is evaluated as negative, and it does not matter whether the p-value is 0.06 or 0.82; negative is negative and the intervention or medication evaluated in the RCT is found to be non-effective.
A major problem with statistical significance is that it is based on a dichotomy with a highly arbitrary cut-off value. When the observed p-value ≤ 0.05, the null-hypothesis is rejected and when the p-value > 0.05, the null-hypothesis is not rejected. Rejecting the null-hypothesis indicates that there is an effect. Not rejecting the null-hypothesis indicates that there is no effect. It should be realized that there is no such dichotomy in medical research. In every study the effect estimate is not equal to zero; so, in every study there is an effect. The p-value of the observed effect gives the probability of finding that particular effect (or more away from the null-hypothesis) when actually the null-hypothesis is true. This p-value is not only related to the magnitude of the effect estimate, it is also related to the sample size and to the heterogeneity in the study population. Although the p-value itself is not a bad indicator, the problem is that an arbitrary cut-off is used to decide whether the null-hypothesis is rejected or not. It should further be realized that hypothesis testing does not say anything about the clinical significance (or clinical relevance) of an estimated effect. And although most researcher more or less will agree with the statement that clinical significance is different from statistical significance, they do not act according to this statement. Besides the fact that there is no dichotomy in evaluating effect estimates in medical research, also the fact that the cut-off value of 0.05 is totally arbitrary is problematic. It is not clear why not using a cut-off value of 0.10 or 0.01 or why not using different cut-off values for studies with different sample sizes.
Regarding reporting results of statistical analysis, the good news is that many researchers nowadays report effect estimates and corresponding 95% confidence intervals in their scientific papers. The bad news, however, is that even as many researchers use the 95% confidence interval for statistical testing only, i.e. when the value of the null-hypothesis lies not in the 95% confidence interval, the estimated effect is statistically significant, which again indicates that there is an effect. (Table 1) summarizes the misunderstandings about statistical significance.
Table 1:Misunderstandings about statistical significance.
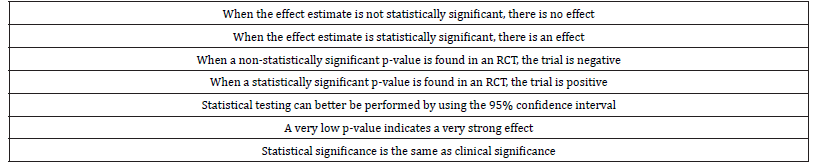
Why statistical testing is still used
The biggest advantage of using statistical significance is that its use is simple and convenient. Everybody uses the same cut-off value to decide whether an estimated effect in a study is important or not. It is seen as an objective scientific indicator so there is no discussion. By using this objective cut-off value, and the believe that statistical significance is more or less the same as clinical relevance, researchers do not have to think much about the clinical relevance of the estimated effect anymore. One of the problems is that a lot of reviewers and journal editors are not aware of the misunderstandings regarding statistical significance and they very often state that in the discussion of a scientific paper, only the statistically significant findings have to be discussed. Because publication is one of the key goals for researchers they are more or less forced to use statistical testing in their research papers.
Misuse of statistical testing: a few examples
A typical example of the misuse of statistical testing is when both crude and adjusted results are reported. In adjusted models, more parameters are estimated so in general the standard errors of the effect estimates are bigger and therefore the p-values are higher. It sometimes happens that a p-value is ≤ 0.05 before adjustment and > 0.05 after adjustment although the effect estimate of the variable of interest is not changed at all. Even in these situations, researchers conclude that after adjustment the result is not statistically significant anymore, and therefore they conclude that after adjustment, actually there is no effect.
Another example is about papers reporting results of RCT’s. In these papers, the results section always start with a table containing descriptive information and in most of those papers this descriptive information is accompanied by p-values from a statistical test comparing baseline values of all kind of variables between the intervention and the control group. In fact, reviewers sometimes ask for the results of the statistical testing, and although this statistical testing does not do much harm, it is often used in a wrong way. What most researchers do is that when the results of an RCT need to be adjusted for certain confounders, they only adjust for baseline variables that differ significantly between the two groups. A typical misunderstanding [15]. It is true that possible confounders have to be associated with the determinant (i.e., they have to differ between the two groups), but this difference does not have to be statistically significant. Furthermore, in this argumentation it is always ignored that the possible confounder must also be associated with the outcome. In fact, when there is only a small (non-significant) difference in baseline value of a particular variable between the two groups, but when that variable is strongly associated with the outcome, this variable will probably be an important confounder.
The last example is performing a meta-analysis. A metaanalysis is the golden standard on which evidence-based medicine is founded. Although this is totally correct, meta-analyses are often used in a wrong way. The general idea of performing a metaanalysis is to increase the sample size in order to obtain a more reliable estimate of the magnitude of a certain effect. However, also results of meta-analysis are often evaluated whether or not the pooled effect estimate is statistically significant and of course, the results of a meta-analysis are often statistically significant. Not because there is a strong effect, but because the sample size is big and therefore almost all estimated effects will be statistically significant.
In what kind of situations, statistical testing can still be used
Statistical testing can play a role when a choice has to be made in statistical modelling. For instance, when in a regression model it has to be decided whether a higher order polynomial (e.g., a quadratic relationship) should be preferred above a lower order polynomial (e.g., a linear relationship) a significant p-value can be used as decision criterion. Also, when it has to be decided whether stratified results should be reported when an interaction term is added to a regression model, statistical significance can play a role in that decision. However, regarding interaction terms, mostly a slightly higher p-value than 0.05 is used as a cut-off value. Finally, when it is necessary to perform variable selection in multivariable regression models, for instance when building a prediction model, statistical significance can be used as selection criterion. However, when a variable selection is performed for building a prediction model it is often advised to use a much higher p-value than 0.05 as cut-off value [16].
What will also change when statistical testing is not used anymore
First of all, the discussion about one-sided versus two-sided statistical testing is not an issue anymore. Although, also when statistical testing is found to be important, the discussion about one-sided versus two-sided testing is basically a non-discussion. This is because for one-sided testing a cut-off value of 0.025 should be used instead of the cut-off value of 0.05. It is striking to see that in real life practice, one-sided testing is mostly used when the onesided p-values are just below 0.05 (i.e., between 0.025 and 0.05). Because only in those situations, the two-sided p-value is not statistically significant. Another intriguing issue in testing theory is multiple testing. The moment more than one analysis is performed, the significance level must be adjusted for the fact that multiple statistical tests are performed. From a theoretical point of view, this is correct, however, when refraining from statistical testing, the whole discussion about multiple testing is not an issue anymore.
What is the alternative for statistical testing
The alternative for statistical testing is not to use some kind of alternative statistical measure [17,18], but to evaluate the effect estimates observed in a particular study by its clinical relevance. This is often not easy, but basically that is what should be done in a discussion section of a scientific paper. Besides that, the uncertainty of the effect estimates (which is given by the 95% confidence intervals) should be discussed. Furthermore, when several outcome variables are analyzed in the same study (which is often the case), one should evaluate the patterns in the observed results instead of focusing only on significant results with or without adjusting for multiple testing.
Conclusion
Because statistical testing is based on an arbitrary cut-off value and a non-existing dichotomy, it should not be used to evaluate the results of medical research. Instead of using statistical significance, the results should be evaluated by its clinical relevance. It is clear that refraining from statistical testing will be a challenge, but it will definitely improve the evaluation of the impact of medical research.
Acknowledgement
None.
Conflict of Interest
No conflict of interest.
References
- Chalmers I (1985) Proposal to outlaw the term “negative trial “. BMJ 290(6473): 1002.
- Rothman KJ (1986) Significance questing. Annals of internal medicine 105: 445-447.
- Goodman SN, Berlin JA (1994) The use of predicted confidence intervals when planning experiments and the misuse of power when interpreting results. Annals of Internal Medicine 121(3): 200-206.
- Altman D, Bland JM (1996) Absence of evidence is not evidence of absence. Australian Veterinary Journal 74: 311.
- Schmidt FL (1996) Statistical significance testing and cumulative knowledge in Psychology: Implications for training of researchers. Psychological Methods 1(2): 115-129.
- Krantz DH (1999) The Null Hypothesis testing controversy in psychology. Journal of the American Statistical Association 44: 1372-1381.
- Sterne JAC, Davey Smith G (2001) Sifting the evidence - what’s wrong with significance tests? BMJ 322(7280): 226-231.
- Haller H, Krauss S (2002) Misinterpretation of significance: A problem students share with their teachers. Methods of Psychological Research Online 7(1): 1-20.
- Greenland S, Senn SJ, Rothman KJ, Carlin JB, Poole C, et al. (2016) Statistical tests, P values, confidence intervals, and power: a guide to misinterpretations. European Journal of Epidemiology 31: 337-350.
- Amrhein V. Korner-Nievergelt F, Roth T (2017) The earth is flat (p > 0:05): significance thresholds and the crisis of unreplicable research. Peer Journal 5: e3544.
- Amrhein V, Greenland S, McShane B (2019) Retire statistical significance. Nature 567(7748):305-307.
- McShane BB, Gal D, Gelman A, Robert C, Tackett JL (2019) Abandon Statistical Significance. The American Statistician 73(sup1): 235-245.
- Wasserstein RL, Schirm AL, Lazar NA (2019) Moving to a World Beyond “p < 0.05”. The American Statistician 73(sup1): 1-19.
- Amrhein V, Greenland S (2022) Discuss practical importance of results based on interval estimates and p-value functions, not only on point estimates and null p-values. Journal of Information Technology 37(3): 316-320.
- Boer MR de, Waterlander WE, Kuijper LDJ, Steenhuis IHM, Twisk JWR (2015) Testing for baseline differences in randomized controlled trials: an unhealthy research behavior that is hard to eradicate. International Journal of Behavioral Nutrition and Physical Activity 12: 4.
- Harrell, Jr. Frank E (2015) Regression Modeling Strategies with Applications to Linear Models, Logistic and Ordinal Regression, and Survival Analysis. Second Edition. Springer International Publishing AG Switzerland.
- Greenland S, Mansournia MA, Joffe M (2022) To curb research misreporting, replace significance and confidence by compatibility: A Preventive Medicine golden jubilee article. Preventive Medicine 164: 107127.
- Mansournia MA, Nazemipour M, Etminan M (2022) P-value, compatibility, and S-value. Global Epidemiology 4:100085.
-
Jos WR Twisk*. Refrain From Statistical Testing in Medical Research; It Does More Harm Than Good. Annal of Pub Health & Epidemiol. 2(3): 2024. APHE.MS.ID.000540.
-
Global warming; climate change; lac +ve and lac-ve mutants; microbiota; lactococcus lactis var. lactis; tornados; tsunami; hurricanes; plasmids; probiotics; public health; iris publishers; iris publisher’s group
-
This work is licensed under a Creative Commons Attribution-NonCommercial 4.0 International License.
- Abstract
- Introduction
- The effect of global warming-climate change on inducing unfavorable mutations in both the beneficial as well as harmful micro-organisms, including viruses, causing severe endemics, epidemics, and pandemics, proven through laboratory research experiments
- Materials and Methods
- Results and Discussion
- Conclusion of Experiment
- The Effect of Global Warming - Climate Change on Human Health
- The Adverse Effect of Global Warming- Climate Change on Agricultural Crops and Food Production
- The Effect of Global Warming- Climate Change on Inducing Adverse Natural Calamities, Around the World
- Possible Ways, Means and Remedial Measures to Control Global Warming- Climate Change to Protect Humanity
- Disclosure
- Acknowledgement
- Conflict of Interest
- References